Exploring Modern Applications of Artificial Intelligence
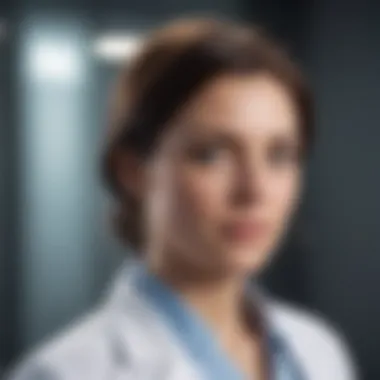
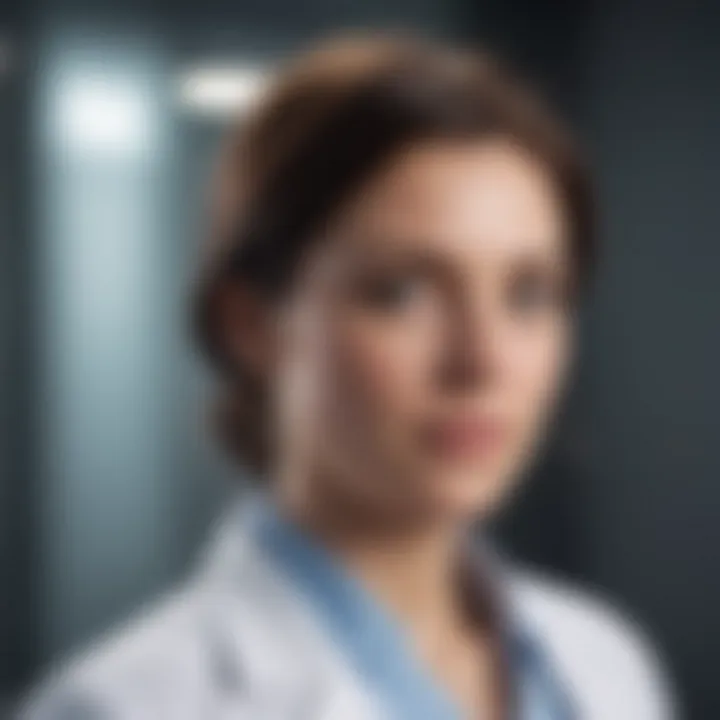
Intro
Artificial intelligence has become ubiquitous in our lives, shaping various industries and significantly changing how we operate. From predictive algorithms in finance to autonomous vehicles in transportation, AI is rewriting the playbook across sectors. It's not just about efficiency or speed; it's about the profound impact AI has on decision-making processes and outcomes. With the ever-increasing data availability, organizations are leveraging AI to gain insights that once seemed unattainable.
Now, as we explore the latest developments in AI applications, we will dive into how this technology affects healthcare, finance, transportation, and education. Each one of these fields shows a different side of AI’s potential, along with the complexities and dilemmas that arise from its use.
Before we embark on this journey, it's worth noting the key areas we will touch upon. We will highlight real-world examples, assess both the advantages and drawbacks, and examine ethical considerations surrounding these emerging technologies. This comprehensive guide aims to provide clarity on the multifaceted roles AI plays today.
Methodology
Study Design
The approach taken for this exploration was multifaceted, combining qualitative and quantitative methods. Case studies from diverse industries were gathered, focusing on recent implementations of AI technologies. This design allows for a thorough analysis, yielding a broad understanding of AI's latest applications.
Data Collection Techniques
Data was collected through a variety of means. Interviews with industry professionals provided firsthand insights, while academic journals and industry reports contributed to a foundational knowledge base. Online platforms, including Reddit discussions and articles from reliable sources such as Wikipedia and Britannica, also helped shape our understanding of ongoing trends.
"The future of AI is not just about technology; it's about shaping the ethics of our society."
— Anonymous
Discussion
Interpretation of Results
The outcomes of this deep dive into AI applications reveal several critical themes. In healthcare, for instance, machine learning algorithms are enhancing diagnostic accuracy. A notable example is the use of AI in radiology, where AI-powered systems analyze imaging data faster than human specialists, leading to quicker and more precise diagnoses.
On the financial front, algorithmic trading is transforming investment strategies. By analyzing market patterns and predicting trends, AI helps investors make calculated decisions that were once the domain of seasoned experts. However, there remains a lingering debate about the implications of such technologies on job displacement.
In education, adaptive learning technologies personalize teaching methods for students, catering to individual learning paces. This application supports teachers in identifying areas where students struggle, promoting a more supportive learning environment.
Limitations of the Study
Despite the comprehensive nature of the study, certain limitations exist. The rapidly evolving nature of AI means that what is considered cutting-edge today could be outdated tomorrow. Additionally, the generalizability of some case studies may be limited by their specific context or industry.
Future Research Directions
Moving forward, further research is required to explore the ethical implications and long-term impacts of AI technologies. How will they reshape the workforce? How will regulations need to evolve? Addressing these questions will be crucial for stakeholders across all sectors to ensure responsible AI development.
Foreword to Artificial Intelligence Applications
Artificial Intelligence (AI) has become a significant player across diverse sectors, reshaping the way we think about technology and its possibilities. In the contemporary landscape, AI isn’t just an academic concept; it's a force that drives change in healthcare, finance, education, and beyond. The importance of exploring the latest applications of AI lies in its potential to enhance efficiency, improve decision-making, and drive innovative solutions to complex problems.
Understanding these latest applications is crucial. First, it enables professionals across various fields to harness AI's full potential, leading to transformative outcomes. For instance, in healthcare, AI tools are not merely add-ons; they help in early diagnosis and personalized treatment, which can save lives and reduce costs.
Secondly, this exploration offers insight into the challenges that accompany AI adoption. Ethical considerations surrounding bias in algorithms, privacy concerns, and the need for transparency cannot be overlooked. Recognizing these challenges early on helps organizations shape their strategies and policies for responsible AI use.
Lastly, keeping abreast of AI developments equips stakeholders with the knowledge to adapt to ever-changing technological advancements. From students to entrepreneurs, everyone can benefit from an understanding of AI applications, fostering a culture of innovation that responds effectively to the needs of society.
Definition of Artificial Intelligence
Artificial Intelligence refers to the simulation of human intelligence in machines that are programmed to think and learn like humans. This broad definition encompasses a range of technologies, including machine learning, natural language processing, and robotics. At its core, AI aims to create systems capable of performing tasks that typically require human cognition—such as visual perception, speech recognition, decision-making, and language translation.
A common yet informative perspective is provided by researchers, who emphasize that AI systems operate through data processing and pattern recognition, enabling machines to adapt their responses based on new data without explicit programming. Therefore, a more focused definition might highlight AI as a subset of computer science aimed at building systems that can automate intellectual tasks.
Overview of AI Evolution
The evolution of AI can be seen as a fascinating journey marked by several milestones. Initially, the concept emerged in the mid-20th century, with pioneers like Alan Turing laying the groundwork through theoretical explorations of machine intelligence. As we moved into the 1960s, the first neural networks were created, revealing the potential for machines to learn from data.
Fast forward to the 1980s, and we witness the rise of expert systems, which used AI to solve specific problems through knowledge-based approaches. However, progress slowed, leading to periods known as “AI winters,” where funding and interest dwindled. The real breakthrough came in the 21st century with the advent of big data and improved algorithms, which reignited interest and investment in AI technologies.
These technological advancements have culminated in the development of sophisticated machine learning models that can now outperform humans in certain aspects, such as image recognition and strategic game play.
"AI is not just a tool; it is embedded into the fabric of our everyday lives, influencing how decisions are made across sectors." - Researcher on AI impacts.
In summation, the trajectory of AI evolution illustrates a continual advancement toward creating systems that are more efficient, intuitive, and capable of solving complex challenges. Understanding this backdrop provides context for recognizing its latest applications and implications throughout various domains.
Healthcare Innovations
The modern healthcare landscape is being revolutionized through the integration of artificial intelligence. One of the most impactful areas where AI is making waves is in healthcare innovations. From improving diagnostic accuracy to personalizing treatment plans, the role of AI is multifaceted. The benefits are myriad; AI helps streamline processes, enhance patient outcomes, and even reduce costs. However, it is not without its hurdles as ethical concerns and data privacy issues loom large.
AI in Diagnostics
Image Analysis
Image analysis stands as a cornerstone in AI-driven diagnostics. Utilizing advanced algorithms, this technology can interpret medical images such as X-rays, MRIs, and CT scans with remarkable precision. A key characteristic of image analysis is its ability to highlight anomalies that might be missed by the human eye. This capability is particularly beneficial in oncology, where early detection of tumors can significantly alter patient outcomes.
What sets image analysis apart is its unique feature of deep learning. This methodology allows the system to learn from vast datasets, continually refining its diagnostic accuracy. However, challenges remain, particularly regarding the interpretability of AI results.
"While AI excels at analysis, the need for human oversight is critical for truly informed medical decisions."
Predictive Analytics
Predictive analytics, in the realm of healthcare, harnesses historical data and machine learning to make informed predictions about patient health. This aspect is vital in creating preventative care strategies. The significant characteristic of predictive analytics lies in its proactive approach; rather than waiting for a medical issue to arise, this AI application anticipates potential health risks.
A unique feature of predictive analytics is its ability to analyze trends over time. By monitoring changes in patient data, healthcare providers can intervene before problems escalate. On the downside, dependence on accurate data means that any inaccuracies can lead to faulty predictions, which may compromise patient care.
Personalized Medicine
Genomic Analysis
Genomic analysis serves as a vital facet of personalized medicine, tapping into the genetic makeup of individuals to tailor treatments specifically for them. This specificity allows practitioners to move beyond generalized treatment approaches. One of the primary reasons genomic analysis is becoming increasingly popular is its ability to identify genetic mutations that influence drug responses.
The unique feature of genomic analysis is its reliance on next-generation sequencing technologies. Though the benefits of personalized treatments are numerous, challenges persist. Issues related to genetic data security and the accessibility of comprehensive genomic information for all patients have sparked heated debate in the medical community.
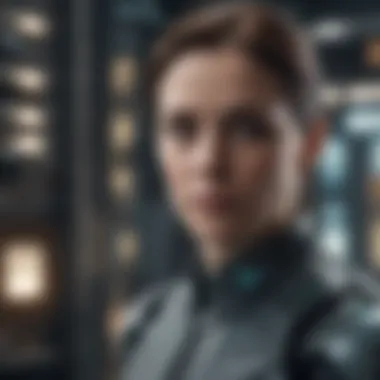
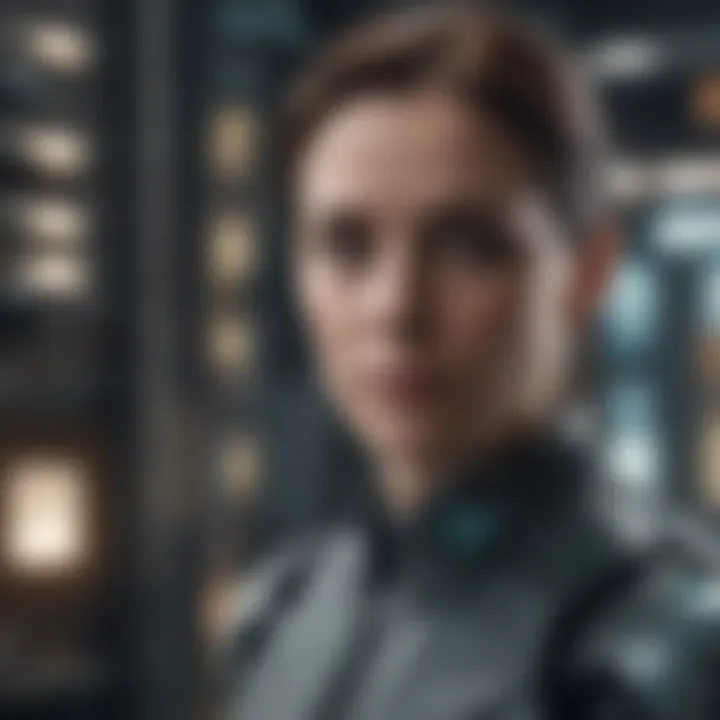
Treatment Optimization
Treatment optimization, closely linked to personalized medicine, involves fine-tuning medical interventions based on patient characteristics and responses. This strategic approach aims to enhance the efficacy of treatments while minimizing side effects. A critical aspect that enhances its appeal is the integration of AI algorithms with clinical decision support systems.
What sets treatment optimization apart is the capacity to adapt in real-time. The health landscape is dynamic, and so should be the treatment plans. However, continuous monitoring and assessment can be resource-intensive, raising questions about scalability in large healthcare systems.
Telemedicine and Remote Monitoring
Telemedicine and remote monitoring represent groundbreaking advancements within healthcare, facilitated by AI. As patient needs evolve, these tools offer the ability to deliver healthcare services remotely, breaking geographical barriers. This accessibility is a game-changer, especially in rural areas. With AI-driven applications, healthcare providers can monitor patient health metrics in real-time, ensuring timely interventions.
While the advantages are clear, reliance on technology poses challenges. Internet connectivity issues and discrepancies in technology literacy among patients can hinder the effectiveness of such systems. Nonetheless, the potential of AI in telemedicine continues to promise a future where quality healthcare is within reach for all.
AI in Finance
The role of artificial intelligence in finance has grown tremendously over the past few years. The sector has started adopting AI systems not just for operational efficiency but also for enhancing decision-making processes. AI isn’t just a buzzword in financial corridors anymore; it’s reshaping how banks, investment firms, and even insurance companies operate. There’s a myriad of advantages, from faster execution of transactions to better risk management strategies.
AI empowers finance with advanced data analytics, enabling companies to sift through mountains of data. But, implementing these technologies isn’t without its hurdles. Regulatory considerations and ethical implications around data privacy stand at the forefront of these discussions. In this way, AI is forging a new path while navigating a minefield of challenges.
Algorithmic Trading
Algorithmic trading is one of the most impactful applications of AI in finance. It's the backbone of modern trading systems, allowing trades to be executed at lightning speed with minimal human intervention. Algorithms can analyze multiple market conditions in real-time, offering traders a crucial edge in decision-making.
High-Frequency Trading
High-frequency trading (HFT) takes algorithmic trading to new heights. It involves executing a large number of orders at extremely high speeds, driven by powerful algorithms. The essence of HFT lies in its ability to capitalize on minute price discrepancies among securities, often making trades within milliseconds.
This technique is widely seen as favorable because of its efficiency. However, it comes with its share of criticisms. Some argue that HFT increases market volatility and may disadvantage regular investors, giving an edge to well-funded institutional players. Yet, despite these concerns, the practice remains a staple in financial markets due to its potential for profit.
Market Predictions
Market predictions are another critical aspect that underscores AI's presence in finance. These AI systems analyze historical data, market sentiment, and even news articles to forecast future movements in stock prices. By identifying patterns, traders can better position themselves in the market.
The highlight of market predictions is their reliance on machine learning. This unique feature allows algorithms to learn from past data, improving their accuracy over time. However, the unpredictability of financial markets can make these predictions only as reliable as the data fed into them. While many traders jump on the AI trend, it's essential to remember that market predictions are not foolproof; they come with risks that need careful consideration.
Fraud Detection
Fraud detection frequently falls into the spotlight when discussing the applications of AI in finance. It’s crucial not only for protecting financial institutions but also for instilling confidence in consumers. AI helps identify and respond to suspicious behaviors faster than traditional methods could.
Anomaly Detection
Anomaly detection is a key technique in the fight against financial fraud. By establishing a baseline of normal customer behavior, AI can quickly spot deviations that might indicate fraudulent activity. This method is beneficial because it can operate in real-time, alerting security teams to potential issues before they escalate.
The standout feature of anomaly detection lies in its adaptability. Over time, as more data accumulates, these systems become better at identifying false positives, enhancing overall accuracy. However, one downside is that even advanced algorithms can miss subtle fraud schemes or lead to false accusations against innocent users.
Behavioral Analysis
Behavioral analysis complements anomaly detection by scrutinizing the actions of users to flag any unusual patterns. It delves into deeper insights, analyzing customers' interaction with services, which can reveal tendencies that automated systems might overlook.
This approach is particularly favorable because it builds a more nuanced picture of user behavior. However, it also raises privacy concerns, as extensive user data collection can be seen as invasive. Striking a balance between security and customer privacy remains a challenge.
Customer Insights and Personalization
In finance, tailoring services to meet customer needs is becoming increasingly crucial. AI has the potential to turn customer data into profound insights, allowing companies to create personalized experiences.
With AI analytics, financial institutions can identify individual preferences, improving everything from service offerings to communication strategies. This personal touch tends to enhance customer satisfaction, fostering loyalty and long-term client relationships. In this rapidly evolving landscape, it's clear that AI-driven insights and personalization are not just conveniences; they are becoming essentials for any forward-thinking financial organization.
Transformative Effects in Transportation
The integration of artificial intelligence in transportation is reshaping how we move from one place to another, introducing innovations that were once the stuff of science fiction. This section highlights key aspects of AI’s role in the transportation sector, where it promises to boost efficiency, enhance safety, and reduce environmental impact. The current and emerging applications of AI in this domain reflect a profound shift in operational paradigms, fostering benefits while also raising important considerations.
Autonomous Vehicles
Technological Challenges
Autonomous vehicles represent a groundbreaking leap in transportation technology. However, they come with a bundle of technological challenges that can't be brushed aside. The primary concern lies in the complexity of algorithms that allow these vehicles to interpret sensory data and make split-second decisions. For instance, how does a self-driving car react in differing weather conditions, or how does it predict the unpredictable movements of pedestrians?
One of the key characteristics of these challenges is the need for extensive data training; autonomous systems must learn from vast datasets to function effectively. This makes them a compelling point of study in this article. The unique feature here is that while these vehicles can significantly reduce human error, their reliance on technology brings new concerns about data security and privacy, particularly if they are constantly collecting information from their environment.
The advantages of addressing these technological challenges include increased road safety and more efficient traffic flow, yet there are severe disadvantages too, such as the potential for system malfunctions and the steep costs of development and deployment.
Regulatory Considerations
Alongside the technological challenges, the regulatory considerations regarding autonomous vehicles are equally demanding. Lawmakers are navigating a tricky path trying to establish rules that both encourage innovation and prioritize public safety. Integral to this discourse is the question of liability in accidents involving self-driving cars.
A critical aspect of these regulatory considerations revolves around how to classify autonomous vehicles within existing traffic laws. This is paramount, it's a beneficial point for this article since it can either hinder or accelerate the adoption of these technologies. The regulations need to balance the unique features of autonomous vehicles’ technology with public safety needs, which can result in conflicting interests.
Thus, while effective regulation could lead to standardized protocols and foster consumer trust, stringent regulations might stifle innovation and delay deployment.
Traffic Management Solutions
Smart Traffic Lights
In this age of rapid urbanization, smart traffic lights have emerged as a remarkable solution aiming to optimize traffic flow. By using AI, these traffic signals adapt in real-time to changing conditions, such as fluctuating traffic volumes or incidents causing delays. What makes smart traffic lights particularly compelling is their ability to communicate with other connected devices, enabling a coordinated response that minimizes congestion.
One notable characteristic of these systems is their reliance on machine learning, which is continuously improving traffic strategies based on historical data. This is not just a beneficial element for this article but also crucial for city planners aiming to reduce commute times and emissions. However, these systems also raise questions around infrastructure costs and the need for regular maintenance.
Predictive Traffic Patterns
In conjunction with smart traffic solutions, the study of predictive traffic patterns is gaining momentum. This application focuses on analyzing data from various sources, including sensors and GPS, to forecast traffic conditions before they occur. By understanding these patterns, city planners and officials can implement timely interventions to enhance road usage efficiency.
The key characteristic of predictive traffic patterns lies in their capacity for proactive decision-making. This potential makes them a popular choice for this article as it aligns with the goals of minimizing delays and improving safety. One unique feature of this system is its ability to incorporate factors like weather forecasts and local events, creating a comprehensive overview of expected traffic trends.
The advantages of using predictive analytics in traffic management include improved safety and less congestion, but on the flip side, it also risks reliance on data accuracy which can be misleading if not monitored correctly.
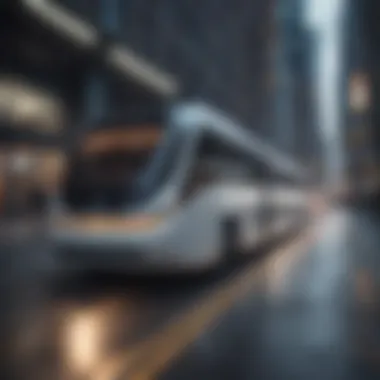
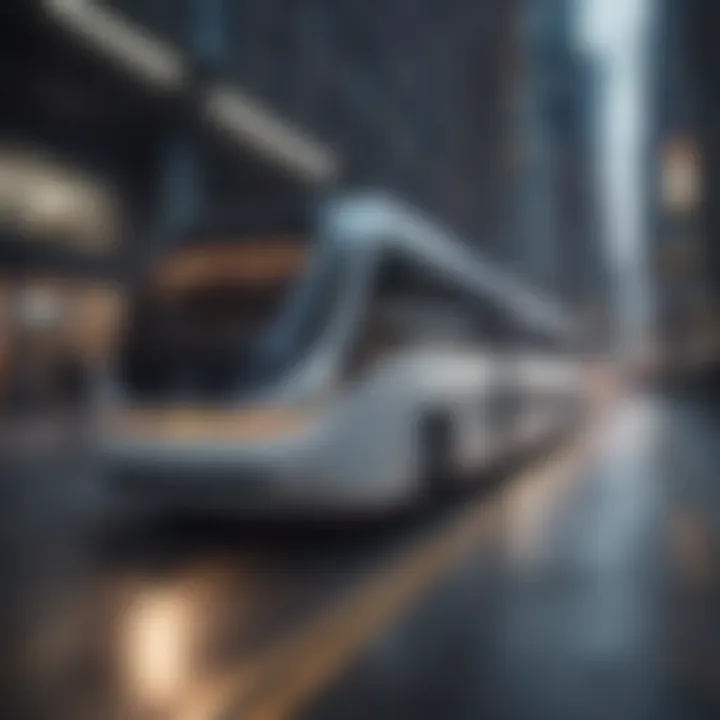
"With the rapid advancement of technology, the future of transportation will not just depend on innovation; it will hinge on regulatory frameworks that guide its integration into societal norms."
As we delve deeper into the transformative role of AI in transportation, it becomes increasingly clear that these applications are not just about enhancing efficiency; they are about envisioning a new paradigm of mobility.
AI in Education
The influence of artificial intelligence in education cannot be overstated. It holds the potential to reshape how teaching and learning happen, paving the way for personalized learning experiences that adapt to the needs of individual students. This section examines the significant aspects of AI's role in education, encompassing specific elements like adaptive learning systems and virtual learning environments.
Adaptive Learning Systems
Adaptive learning systems are tailored to meet the unique needs of each student. They rely heavily on data analysis and AI algorithms to customize the learning experience. By assessing a learner's abilities, preferences, and areas where they might struggle, these systems can adjust the learning path accordingly. This means that a student who grasps certain concepts quickly can move on, while another who requires more time can receive additional resources and support.
The key characteristic of adaptive learning systems is their flexibility. They offer a dynamic learning experience that was unheard of a few decades ago. This personalized approach not only enhances engagement but also promotes a deeper understanding of the subject matter.
However, implementing adaptive learning does come with challenges. It requires robust technological infrastructure and ongoing adjustments based on feedback, making it a continuous process rather than a one-time solution. Yet, the potential benefits – like improved student outcomes and satisfaction – often outweigh these challenges.
Virtual Learning Environments
Virtual learning environments (VLEs) represent another significant facet of AI in education. They provide platforms where students can access course materials, communicate with instructors, and collaborate with peers, all from the comfort of their homes. Here, technology breaks the geographical barriers that traditionally hampered education.
AI Tutors
AI tutors are increasingly becoming a fixture in virtual learning environments. They can offer personalized assistance, answering questions, providing resources, and even engaging students in discussions. One major advantage of AI tutors is that they are available 24/7, accommodating students who may have different schedules or those who need additional help outside regular hours.
A standout feature of AI tutors is their ability to analyze student interactions and performance data. This analysis allows them to identify weaknesses and suggest tailored strategies to improve. Furthermore, they can adapt their teaching methods based on the student's learning pace. However, while AI tutors can complement traditional teaching, they shouldn't replace human interaction entirely, as students benefit from social learning and mentorship.
Engagement Analytics
Engagement analytics harnesses AI to monitor and assess student participation and interaction with learning materials. By analyzing data, educators can identify trends and patterns in student engagement, enabling them to determine which resources resonate most and which may need re-evaluation. The primary benefit of this approach is that it allows educators to adjust their strategies in real time, enhancing the overall effectiveness of the learning experience.
One of the notable features of engagement analytics is its predictive capability. By understanding how various factors influence student engagement, educators can anticipate potential drop-offs or challenges, allowing them to implement preventative measures.
Yet, while engagement analytics can significantly enhance the learning process, it raises questions about data privacy and the ethical use of student information. Striking a balance between effective monitoring and respecting student privacy is crucial as schools navigate this landscape.
In summary, artificial intelligence is deeply engrained in modern educational practices. From adaptive learning systems that personalize the learning experience to AI tutors and engagement analytics, these technological advancements not only hold promise but also bring forth ethical considerations. Embracing AI in education requires a thoughtful approach, recognizing that these tools are meant to enhance rather than replace the invaluable human elements of teaching.
AI in Manufacturing
In the realm of manufacturing, the integration of artificial intelligence has ignited a transformation that redefines industry standards and operational protocols. The significance of AI in this sector cannot be overstated as it provides manufacturers with tools to enhance productivity, reduce costs, and improve quality. Companies are not just adopting these technologies for the sake of modernity; they are doing so to stay competitive in an increasingly global market. From predictive maintenance to quality control automation, employing AI is no longer viewed as optional but rather essential to achieving success in manufacturing.
Predictive Maintenance
Predictive maintenance stands at the forefront of smart manufacturing practices. This concept revolves around utilizing AI algorithms to analyze data from machinery, detecting wear and tear before it leads to failure. By leveraging machine learning and data analytics, organizations can anticipate equipment issues that would otherwise result in costly downtimes and extensive repairs.
For instance, consider a manufacturing plant that produces automotive parts. With sensors implanted in critical machinery, real-time data is collected and analyzed. AI systems can identify slight variations in machine performance, signaling an impending malfunction. This foresight enables maintenance teams to schedule repairs during non-productive hours, minimizing disruptions in the production line. Such proactive strategies are akin to changing the oil in your car before the engine grinds to a halt – addressing problems before they escalate.
"The best repair is the one you never have to make."
The benefits of predictive maintenance extend beyond mere cost savings; they help in extending the lifespan of equipment significantly. Industries can witness tangible improvements in efficiency, can align human resources effectively, and can make informed decisions regarding the purchase of new machinery.
Quality Control Automation
Another pivotal application of AI in manufacturing is in quality control automation. Quality assurance processes have traditionally relied on manual inspections, which can be subjective and prone to error. AI circumvents these challenges by employing neural networks and computer vision technologies that allow for precise and consistent quality assessments.
Imagine an assembly line where each product goes through a visual inspection. AI-powered cameras can instantly analyze every item against a set of predefined quality parameters. This technology not only speeds up the inspection process but also increases accuracy, ensuring that only products meeting quality standards move forward to the next phase of production.
The impact of quality control automation is profound. Manufacturers can reduce the incidence of defective products, which in turn minimizes waste and boosts consumer confidence. Moreover, this technology facilitates continuous improvement. Data collected during the inspection process can be fed back into the manufacturing system to enhance product designs or production techniques.
To summarize, as manufacturing continues to evolve, the role of AI becomes more prominent. Predictive maintenance and quality control automation are two clear examples where artificial intelligence not only improves efficiency but also redefines operational standards. Organizations that harness the power of AI are likely to see not just an uptick in productivity, but also a more sustainable approach to manufacturing challenges.
Artificial Intelligence in Retail
The retail sector is undergoing a seismic shift, and at the center of this transformation is artificial intelligence. Retailers are leveraging AI technologies to optimize operations, enhance customer experiences, and navigate an increasingly complex marketplace. As consumer demands evolve, retailers must adapt quickly, and AI provides tools for making educated decisions based on data analysis, predictive models, and real-time insights. The integration of AI into retail not only streamlines processes but also fosters personalized shopping experiences, thus creating a win-win situation for both businesses and their customers.
Inventory Management Systems
Effective inventory management is the backbone of any retail operation. With the advent of AI, businesses can now monitor stock levels in real-time, anticipate demand fluctuations, and manage supply chains more effectively. An AI-driven inventory management system can analyze historical sales data, seasonal trends, and even social media sentiment to predict which products will be in demand. This proactive approach reduces the costs associated with overstocking or understocking products.
Additionally, these systems can automatically reorder products when stock reaches a certain threshold, ensuring that popular items are always available without the hassle of manual monitoring. In an industry where timing can be everything, AI provides the agility required to stay ahead.
- Key Benefits of AI in Inventory Management:
- Real-time tracking of stock.
- Improved demand forecasting.
- Enhanced decision-making from data insights.
Customer Experience Enhancement
With competition continually rising in the retail sector, enhancing the customer experience is vital for retaining loyalty and driving sales. AI technologies such as chatbots and personalized recommendations play crucial roles in achieving this goal.
Chatbots
Chatbots are revolutionizing how retailers interact with consumers. These AI-powered virtual assistants provide immediate responses to customer inquiries, whether that’s trackin packages or answering product-related questions. They operate around the clock and can handle multiple customer interactions simultaneously, which eases the workload on human staff. This immediacy not only improves customer satisfaction but also minimizes wait times, leading to higher conversion rates.
One key characteristic of chatbots is their ability to learn from each interaction. As they gather data over time, they become more adept at addressing complex queries and providing tailored support. Additionally, since they do not tire or require breaks, they can consistently deliver a high level of service.
- Advantages of Chatbots:
- Instant support for customers, 24/7.
- Reduces operational costs by managing simple queries.
- Gains insights from customer interactions to improve offerings.
Personalized Recommendations
Personalized recommendations driven by AI algorithms have become a mainstay in modern retail. By analyzing purchasing habits, browsing histories, and user preferences, retailers can offer tailored product suggestions. This level of personalization helps to create a unique shopping experience for each customer.
This capability serves to foster customer loyalty, as consumers appreciate feeling understood by a brand that suggests products aligned with their interests. Moreover, AI can continuously update recommendations based on recent data, ensuring relevancy. Such a dynamic approach not only boosts sales but enhances overall customer engagement.
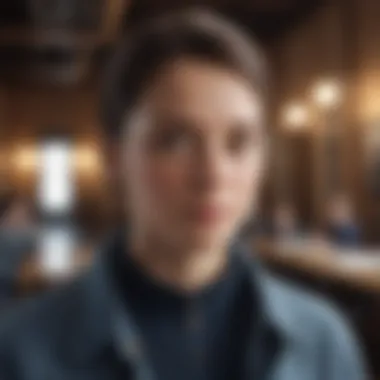
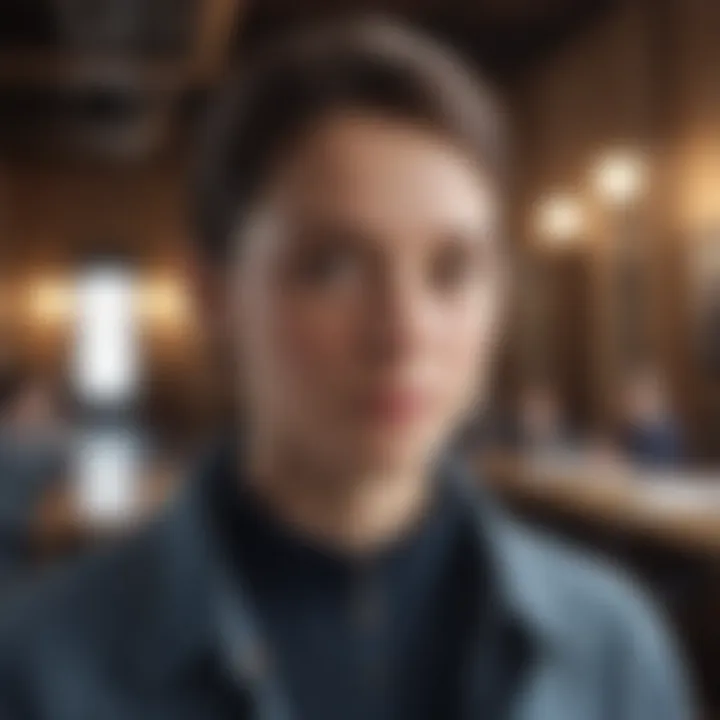
- Key Features of Personalized Recommendations:
- Tailored offerings based on previous purchases.
- Real-time adjustments as user behaviors change.
- Increased conversions due to relevance of suggestions.
In summary, integrating artificial intelligence within retail operations significantly affects inventory management and customer engagement. Through AI-driven inventory systems and personalized consumer interactions, retailers not only streamline their workflows but also enhance the shopping experience, ultimately resulting in increased profitability and customer satisfaction.
The successful incorporation of AI technologies allows retailers to navigate the complexities of modern commerce, keeping them firmly positioned in the ever-evolving marketplace.
Ethical Considerations
Understanding the ethical landscape that surrounds artificial intelligence applications is crucial for several reasons. As AI technologies take hold across sectors—from healthcare to finance—the implications of their deployment can shape society significantly. With increasing capabilities come hefty responsibilities. The focus on ethics in AI ensures that these technologies are used fairly and transparently, protecting individuals and communities from unintended harm.
When we talk about ethical considerations in AI, we are typically referencing two vital areas: bias in algorithms and the need for transparency and accountability. By delving into these aspects, we can better comprehend the potential pitfalls and dangers AI poses to society at large.
Bias in AI Algorithms
The issue of bias in AI algorithms is akin to a double-edged sword. On one hand, artificial intelligence is heralded for its ability to process vast amounts of data rapidly and accurately. On the other hand, if this data contains inherent biases, the algorithms can magnify those biases, leading to unethical outcomes.
For instance, AI systems used in hiring processes may favor candidates from certain backgrounds while unfairly disadvantaging others. This scenario highlights a troubling truth: if the data fed into AI systems is biased, the outputs will also be biased. This doesn't just affect job applicants; it can also impact loan approvals, law enforcement, and health care diagnostics.
Moreover, it's worth noting that the algorithms themselves might not be inherently biased. The way they learn from data is crucial. If the initial datasets are unbalanced or represent only a specific group, the chance for skewed results skyrockets. To combat this, companies need stricter guidelines around dataset curation and algorithm training.
Here’s a short list of the implications of biased AI:
- Discrimination: Certain groups may be unfairly targeted or overlooked in various applications, causing systemic inequality.
- Mistrust: Algorithms that produce biased outcomes can erode public trust in institutions that employ these technologies.
- Legal Repercussions: Businesses could face legal action if their AI systems are shown to systematically favor certain demographics over others.
Transparency and Accountability
In addition to addressing biases, the importance of transparency and accountability in AI cannot be overstated. When AI systems operate as a ‘black box,’ it often leads to confusion and skepticism from users and stakeholders. If people can't see how decisions are being made, they are less likely to trust those decisions.
For instance, consider a scenario where an AI system is deployed to determine credit scores. If applicants are denied loans but cannot access the criteria used for these decisions, they are left in the dark, unable to understand or challenge the ruling. This situation calls for robust transparency measures, ensuring that the mechanisms behind AI decisions can be scrutinized and understood by those affected.
Here are some essential elements of promoting transparency and accountability:
- Clear Documentation: AI developers should provide comprehensive information about how algorithms function and the type of data being utilized.
- Explainable AI: Efforts should be made to develop AI systems that can articulate their decision-making processes in a user-friendly manner.
- External Audits: Regular audits by independent third parties can help ensure that AI systems comply with ethical standards and regulations.
"Trust in AI is not merely about its intelligence; it's about its ability to explain itself to us in a meaningful way."
By prioritizing ethical considerations in AI deployment, organizations can mitigate risks and foster an environment where technology serves humanity rather than hinders it. As we forge further into a future dominated by AI, keeping these ethical principles at the forefront will be crucial for societal well-being.
Future Directions of AI Applications
The future of artificial intelligence applications is an intriguing topic, not just for tech enthusiasts but for anyone invested in the progress of society. With the rapid pace of technological advancements and the increasing integration of AI in everyday life, understanding potential future directions becomes crucial. This exploration focuses on emerging technologies and societal impacts, laying out a fundamental framework for what lies ahead.
Emerging Technologies
Quantum Computing
Quantum computing stands out as a substantial leap forward in computing capabilities, primarily due to its unique approach to processing information. Unlike classical computers that use bits, quantum computers utilize quantum bits or qubits, which can represent and store information in multiple states at once. This characteristic allows them to tackle complex problem-solving tasks far more efficiently than traditional machines. In terms of AI, this capability holds immense promise.
One of the most significant contributions of quantum computing to AI is its potential to enhance machine learning algorithms. The processing speeds can be magnitudes faster, allowing for quicker training of models. Quantum computing could revolutionize industries reliant on vast data processing, like finance and healthcare, offering opportunities for advancements that were previously deemed unattainable. However, developing practical quantum systems remains a challenge; they require precise conditions to function and are currently costly to maintain.
Neuromorphic Computing
Neuromorphic computing simulates the neural structure and functioning of the human brain, offering a fresh approach to computing that mirrors how biological systems operate. The key characteristic of this tech is its ability to process information in real-time while consuming significantly less power than conventional computing methods. This feature makes it particularly attractive in the context of mobile and IoT (Internet of Things) devices.
A unique advantage of neuromorphic systems is their capability to learn from their environments continuously. Unlike traditional AI models that require extensive datasets to reach satisfactory performance levels, neuromorphic systems adapt on the fly. However, the development of this technology also faces obstacles, including the lack of robustness and difficulties in scaling it for broader application.
Potential Societal Impacts
Job Displacement
As artificial intelligence continues to develop, job displacement has become a pressing concern. Automation, propelled by AI, is transforming industries in ways that may render certain jobs obsolete. This transformation affects various sectors, from manufacturing to customer service, where autonomous systems can outperform human workers in speed and efficiency. The discourse surrounding job displacement highlights a challenging dilemma: while AI can improve productivity, it can also lead to significant layoffs and economic uncertainty for workers in vulnerable positions.
Nevertheless, it’s critical to understand that job displacement isn’t the end of the road. New job categories could emerge in fields requiring human oversight of AI systems, therefore creating a shift in the job market rather than an outright loss. The ideal scenario is encouraging workers to upskill and transition into roles where they can effectively collaborate with new technologies.
Economic Shifts
Another fundamental aspect of AI's future involves potential economic shifts it may bring to societies worldwide. AI technologies could disrupt traditional business models, creating opportunities for agile startups while challenging longstanding corporations that may struggle to adapt. This shift can also modify consumer behavior; for instance, personalized AI recommendations can alter purchasing patterns, influencing how products are marketed and sold.
While these changes can lead to economic growth and innovation, they can also exacerbate existing inequalities. Wealth generated by AI might not reach all layers of society evenly, leading to increased economic divides. As societies increasingly rely on AI, there arise calls for thoughtful policies to mitigate negative impacts and ensure equitable growth.
"Understanding these emerging technologies and their societal implications is crucial for navigating and shaping our future in a world increasingly driven by AI."
In summary, the future directions of AI applications portend exciting possibilities. Both quantum and neuromorphic computing stand out as pivotal technologies that may redefine how AI operates, while job displacement and economic shifts represent challenges we must collectively confront. Continued dialogue and research in these areas are essential for creating a balanced and informed approach to the integration of artificial intelligence into our society.
Finale
The impact of artificial intelligence on various domains cannot be overstated. From revolutionizing healthcare practices to enhancing financial strategies, AI applications have reshaped how industries function and evolve. The significance of AI, especially in its latest iterations, is tied not only to operational efficiency but also to its ability to create personalized experiences and predict future outcomes.
Summary of Findings
In this article, we explored several key areas where AI has made significant advances:
- Healthcare Innovations: The ability of AI to analyze images for quicker diagnostics and tailor treatments based on patient data has shown remarkable promise. Predictive analytics helps foresee health declines before they manifest.
- AI in Finance: The rise of algorithmic trading and fraud detection systems underscores AI's potential in safeguarding financial interests and optimizing trading strategies. Customer insights are now deeper and more actionable.
- Transformative Effects in Transportation: Autonomous vehicles are not just a futuristic concept; they’re a reality facing technological and regulatory hurdles. Smart traffic systems utilize AI to ease congestion.
- AI in Education: Adaptive learning programs allow for personalized educational experiences, promoting better engagement and effectiveness.
- Ethical Considerations: As AI's reach expands, so too does the need to address issues of bias and system transparency. Understanding these factors is crucial for sustainable growth.
All these insights show that understanding AI's applications isn’t a mere academic pursuit; it’s a practical necessity for staying relevant in an AI-driven world.
Call for Continued Research
The discussion surrounding AI cannot end with the current findings. There is an urgent need for continuous research and dialogue regarding:
- Emerging Technologies: New methods, like quantum computing, could redefine what is feasible with AI.
- Societal Impacts: As AI takes on a larger role in society, its influence on job markets and economic structures will need expansive study to address potential upheavals.
Calling for ongoing exploration, it's critical for scholars, engineers, and policymakers to cooperate. They should meticulously assess the ramifications of AI technology and strive for frameworks that ensure ethical development and equitable distribution of AI's benefits.
"Without a clear roadmap for responsible AI advancement, we risk jeopardizing the very foundations of trust and innovation we've been striving to build."
To encapsulate, the journey into AI's vast applications is just beginning. As we probe deeper and expand our comprehension, both the challenges and opportunities will unfold like pages of an unwritten tale. Let’s get involved in creating the next chapters together.