Understanding Life Science Statistics: Key Insights
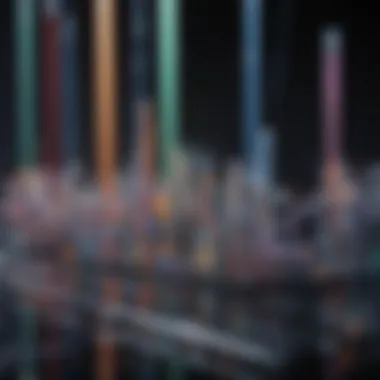
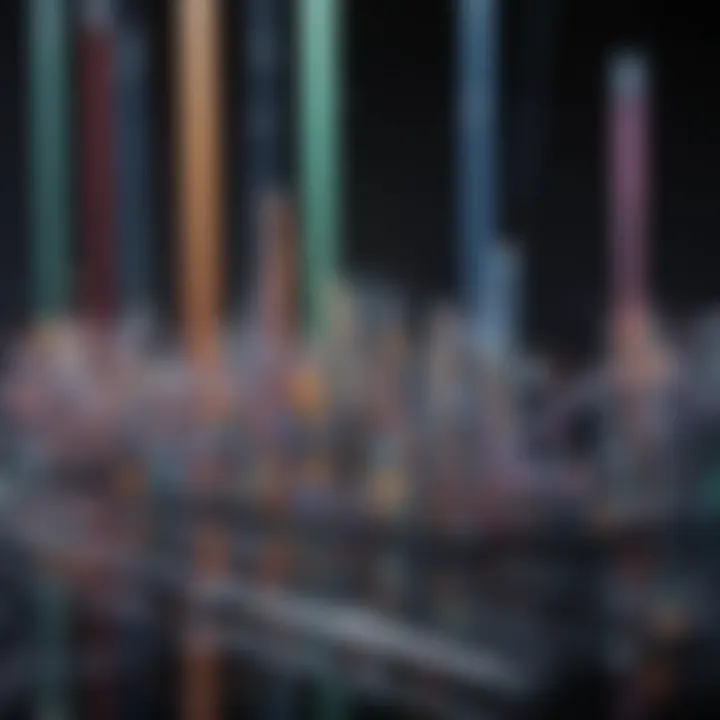
Intro
Statistics play a crucial role in the life sciences, guiding researchers in understanding complex biological systems. The beauty of applying statistics in these fields lies in its ability to unveil patterns, provide insights, and validate findings in a systematic way. Without a solid grasp of statistical methods, researchers may find it difficult to make sense of large sets of data generated during experiments.
In this article, we will explore several areas of life science statistics. We will discuss fundamental methodologies, key concepts, and their real-world applications. By understanding these topics, students, researchers, and educators can appreciate how statistics contribute to biological discoveries.
Methodology
Statistics in life sciences depend heavily on robust methodologies to ensure reliability and validity.
Study Design
Study design serves as the backbone of any research project. It lays the groundwork for how data will be collected and analyzed. Common designs include:
- Randomized Controlled Trials (RCTs): Participants are randomly assigned to different treatment groups. This reduces bias and helps establish cause-and-effect relationships.
- Cohort Studies: Researchers observe a group over time, examining the impact of various factors on health outcomes.
- Cross-Sectional Studies: Data is collected at a single point in time, providing a snapshot of a population.
Data Collection Techniques
Once the study design is set, data collection becomes the next critical phase. Several techniques are widely used:
- Surveys and Questionnaires: These tools gather self-reported data from participants, providing insights into behaviors and experiences.
- Experiments: Controlled experiments allow researchers to manipulate variables and observe effects, leading to more conclusive results.
- Observational Studies: Researchers collect data without intervening, useful for studies where experimental manipulations are not feasible.
"Effective methodology is essential in life science statistics, as it lays the foundation for reliable conclusions."
Discussion
After the data collection, the next step is interpretation.
Interpretation of Results
Results should be interpreted carefully, taking into account context and limitations. Proper statistical analyses, including regression and ANOVA, help in understanding the significance of findings. Well-illustrated graphs and tables enhance clarity and presentation of data.
Limitations of the Study
Every research project has limitations. These may arise from sample size, measurement accuracy, or potential biases. Recognizing limitations does not diminish the value of findings; instead, it provides a roadmap for future exploration.
Future Research Directions
Future research can address current limitations, explore uncharted questions, and refine methodologies. Areas for further investigation might include:
- Longitudinal Studies: Following subjects over time to observe changes.
- Meta-Analyses: Combining data from multiple studies to find broader trends and effects.
By embracing robust statistical methodologies, researchers can significantly contribute to advancements in biology and environmental science, enhancing understanding in these vital fields.
Foreword to Life Science Statistics
Statistics in life sciences is not merely an accessory; it serves as a cornerstone for analyzing data related to living organisms. The role of statistics extends beyond simple calculations to encompass the interpretation, validation, and communication of scientific findings. In the context of life sciences, statistical methods are vital for drawing conclusions from experimental data, whether it be in biology, medicine, or environmental studies.
The introduction to this topic outlines the significance of understanding statistical processes and frameworks that guide research methodologies. In a field where every data point can influence critical outcomes, the proper application of statistical techniques becomes imperative.
Definition and Scope
Life science statistics refers to a collection of principles and methodologies used to gather, analyze, and interpret data pertaining to biological and biomedical phenomena. This discipline covers a wide array of practices, from designing experiments to analyzing complex datasets. The scope encompasses various areas, including:
- Biostatistics
- Epidemiology
- Clinical trials
- Environmental statistics
By offering a structured approach to data analysis, life science statistics equips researchers with the necessary tools to derive meaningful insights. This enables scientists to address queries related to health outcomes, biological variations, and ecological impacts.
Historical Context
The historical foundations of life science statistics trace back to early attempts at understanding natural occurrences through quantifiable measures. Notably, in the 18th century, John Graunt's work on mortality records in London laid the groundwork for modern epidemiology. The evolution continued through the 19th and 20th centuries with contributions from figures like Ronald Fisher, who introduced concepts of statistical significance and experimental design.
In contemporary science, the importance of life science statistics has only grown, paralleling the rapid advancements in biological research and technology. With the advent of computer technology, the complexity of datasets has increased, necessitating sophisticated statistical methods for effective analysis. Today, the integration of life science statistics into research has become standardized and indispensable in validating scientific discoveries.
Importance of Statistics in Life Sciences
Statistics is fundamental in life sciences. It facilitates the analysis and interpretation of complex data. Accurate statistical methods are essential for making informed decisions based on empirical evidence. The role of statistics extends through various life science fields, including biology, medicine, and environmental science. In these domains, science relies heavily on numerical data to assess hypotheses and draw conclusions.
Data Interpretation
Data interpretation is a critical aspect of statistics in life sciences. It involves analyzing collected data to extract meaningful information. Without proper interpretation, raw data lacks value. Biologists use statistics to convert data points into insights that help clarify intricate biological processes.
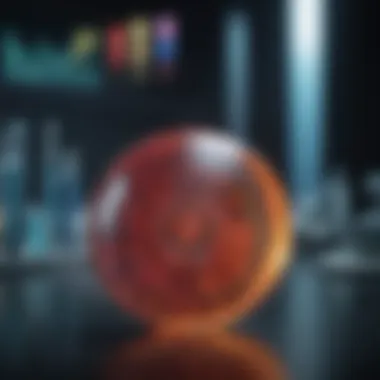
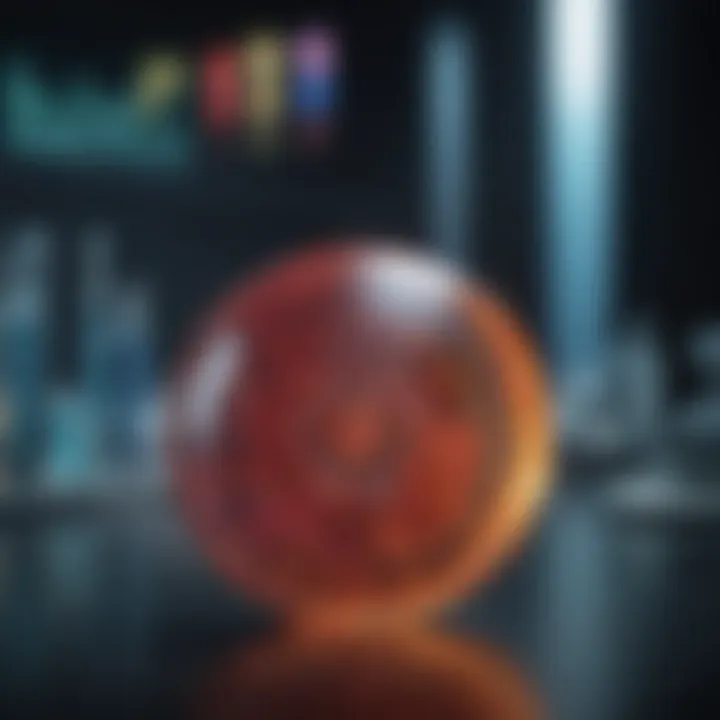
To interpret data effectively, researchers often employ various statistical tools. Techniques like regression analysis or hypothesis testing allow scientists to establish relationships within their data set. The correct interpretations can influence future research directions and determine the success of medical trials and ecological studies. Moreover, inadequate data interpretation can lead to misleading conclusions, impacting public health policies or conservation efforts.
- Accurate interpretation promotes understanding of biological phenomena.
- It influences hypotheses formation and testing in scientific research.
- Misinterpretation can have significant consequences, leading to incorrect decisions.
Enhancing Research Validity
Validity is crucial for any scientific research. Statistics enhances this validity by ensuring that the results presented are reliable and generalizable. When researchers apply statistical methodologies correctly, they bolster their findings' authenticity. Life science researchers must account for biases and variability in their data, which can cloud research findings.
Implementing statistical techniques helps minimize errors and bias that might otherwise distort results. For instance, random sampling ensures each member of a population has an equal chance of being included in the study, thereby enhancing the external validity. It is also essential for researchers to understand the limitations of their statistical methods to avoid overstating conclusions.
Good statistical practice leads to:
- Higher consistency in experimental results.
- Improved credibility among peers and stakeholders.
- Better-informed policy and clinical decisions.
"The power of statistics lies in its ability to distill complexity into clarity, providing a foundation for informed decision-making in life sciences."
Fundamental Statistical Concepts
In the domain of life sciences, a strong grasp on fundamental statistical concepts is imperative. These concepts serve as the bedrock for data analysis, influencing both the methodology and the interpretation of results. Understanding the distinctions between population and sample, along with the various forms of statistics, builds the framework for robust research designs. Mastery of these topics not only enhances the accuracy of findings but also ensures the integrity of scientific conclusions.
Population vs. Sample
In statistical research, the terms population and sample hold significant value. A population refers to the complete set of instances or subjects that meet a particular set of criteria. This could encompass all the individuals with a certain disease, all cells of a particular type, or any other comprehensive group of interest. Conversely, a sample is a subset of the population, selected to represent the broader group. Samples are essential because they allow researchers to conduct studies when accessing the entire population is impractical or impossible.
The importance of understanding the difference between these concepts lies in the implications for generalization and accuracy. If a sample is not representative of the population, the conclusions drawn from it may lead to incorrect assumptions and bias in research outcomes. Researchers must therefore design sampling methods carefully. For example, simple random sampling or stratified sampling might be employed to enhance representation.
Descriptive Statistics
Descriptive statistics are crucial in summarizing and presenting data in a meaningful way. They provide a concise overview of the data set, allowing researchers to quickly ascertain patterns or trends. Common descriptive statistics include measures of central tendency such as mean, median, and mode, as well as measures of variability like range, variance, and standard deviation.
Using descriptive statistics, scientists can create visual representations of data, such as graphs and charts. This is particularly useful in life sciences, where complex data often need simplification. For instance, a histogram can effectively depict the frequency of different values in a data set, offering immediate insights into its distribution. Moreover, descriptive statistics help in identifying anomalies and trends that may warrant further investigation.
Inferential Statistics
Inferential statistics take analysis a step further by allowing researchers to make predictions or inferences about a population based on sample data. This method is integral to hypothesis testing and determining the potential significance of results. Inferential statistics typically involve the use of confidence intervals and p-values to assess the validity of hypotheses.
In life sciences research, inferential statistics help scientists deduce whether observed effects are likely due to chance or represent genuine phenomena. For instance, if a clinical trial compares the effectiveness of two drugs, inferential statistics enable researchers to declare one drug superior based on the data from a sample of patients, extrapolating the results to the wider population under study.
The application of inferential statistics requires a deep understanding of statistical power and effect size, as these concepts impact the reliability of conclusions drawn from data. This is particularly essential in life sciences, where anecdotal evidence is insufficient to support medical or ecological claims.
Understand these fundamental concepts well. They are vital for accurate data interpretation and valid research conclusions.
Overall, a solid comprehension of fundamental statistical concepts is indispensable in life sciences. They form the basis for methodological integrity, ensuring that research findings are credible and informative.
Data Collection Methods
Data collection is a critical aspect of life science statistics. The choice of method can determine the integrity and relevance of research outcomes. Proper data collection allows researchers to gather valuable evidence and identify patterns or correlations essential for scientific exploration. In life sciences, robust data collection methods link theoretical concepts with practical applications, driving advancements in fields like medicine, ecology, and biology.
Experimental Design
Experimental design refers to the structure and planning of experiments. It ensures that data collected has high validity and can effectively address the research question. Key benefits of a well-structured experimental design include:
- Control of Variables: A solid design helps isolate the effects of the variable under study, reducing confounding factors.
- Reproducibility: Results from well-designed experiments can be replicated by other researchers, strengthening the reliability of findings.
- Statistical Power: Adequate sample size and randomization enhance the ability to detect significant effects.
Researchers must also consider potential biases. Sample selection, timing of data collection, and environmental influences can all affect results. A/1 systematic approach is thus necessary to mitigate these issues and enhance the study's credibility. Proper training of personnel involved in the collection process is also essential for maintaining data integrity.
Observational Studies
Observational studies serve as a complementary method to experimental designs. In these studies, researchers observe subjects without manipulation, focusing on correlations rather than cause-and-effect relationships. This method is particularly valuable in scenarios where experimenting is impractical or unethical, such as in certain medical or ecological research settings.
The advantages of observational studies include:
- Real-world Context: They provide insights into natural behaviors and conditions, allowing for more generalizable results.
- Cost-effectiveness: Such studies often require fewer resources than experimental designs.
- Ethical Considerations: They provide a framework to study sensitive topics without creating ethical dilemmas.
However, observational studies also come with limitations, such as potential biases in data selection and the inability to definitively establish causality. Researchers must maintain an awareness of these limitations during their analysis and when interpreting results.
"Well-planned data collection strategies enhance the robustness of findings and promote better understanding of phenomena in life sciences."
In summary, both experimental design and observational studies are foundational to effective data collection in life sciences. Each method has unique strengths and considerations that researchers must weigh carefully to ensure the validity and reliability of their findings.
Statistical Techniques in Life Sciences
Statistical techniques form the cornerstone of research in life sciences. They enable scientists to analyze complex data, assess variability, and draw meaningful conclusions. As research evolves, the integration of sound statistical practices becomes indispensable. By applying robust methodologies, researchers enhance the credibility and reliability of their findings. This section delves into key statistical techniques commonly employed in life science research, specifically focusing on hypothesis testing, regression analysis, and ANOVA techniques.
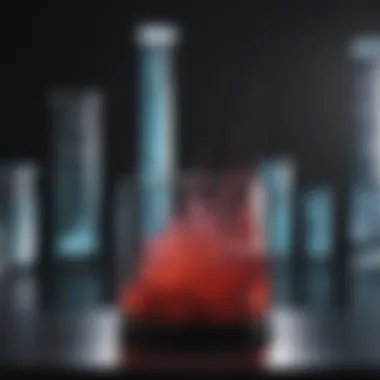
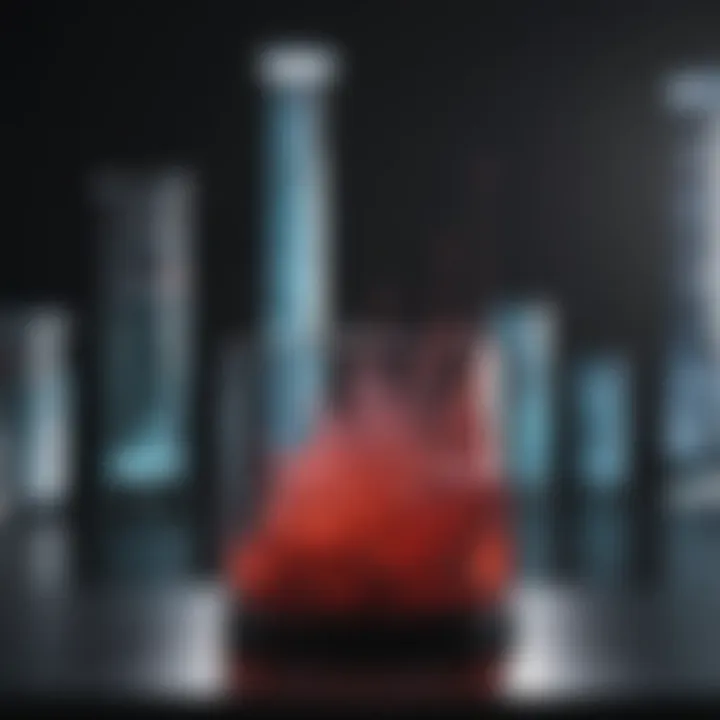
Hypothesis Testing
Hypothesis testing is a critical component in life sciences, serving as a formal mechanism to evaluate scientific claims. It provides a structured approach to decision-making based on data. Researchers typically identify a null hypothesis, which posits no effect or relationship, and an alternative hypothesis that suggests an effect or relationship exists.
One primary benefit of hypothesis testing is its ability to reduce uncertainty. By employing statistical tests such as the t-test, Chi-square test, or Mann-Whitney U test, researchers can quantify the likelihood of observing data under the null hypothesis. Additionally, p-values play a crucial role in this process. A low p-value indicates strong evidence against the null hypothesis, prompting researchers to consider the alternative hypothesis as valid.
However, caution is paramount in interpreting results. Misinterpretations can occur, especially with multiple comparisons. Researchers should embrace corrections, like the Bonferroni correction, which mitigates the risk of false discoveries.
Regression Analysis
Regression analysis is another vital statistical technique used extensively in life sciences. It allows researchers to examine the relationships between variables. Through linear regression, for example, scientists can quantify how changes in an independent variable are associated with variations in a dependent variable.
The utility of regression lies not only in predictive modeling but also in the ability to identify influencing factors. For instance, in clinical studies, a researcher might use regression to understand how age, sex, or medical history affects drug efficacy. Multiple regression takes this further, incorporating several independent variables simultaneously to assess their combined impact.
Nevertheless, it is crucial to validate regression models. Checking assumptions like linearity, homoscedasticity, and multicollinearity ensures the robustness of the findings. Thus, careful consideration and validation in regression analysis are essential for drawing accurate conclusions.
ANOVA Techniques
ANOVA, or Analysis of Variance, is a powerful statistical technique employed to compare means across multiple groups. It diverges from traditional t-tests by assessing several groups simultaneously, which enhances the efficiency of data analysis.
One key advantage of ANOVA is its ability to detect significant differences among group means while controlling for Type I error. For instance, in a study investigating the effects of various diets on weight loss, ANOVA allows researchers to compare weight loss across three or more dietary groups in one consolidated test.
Among the different types of ANOVA, one-way ANOVA is commonly used when comparing a single factor, while two-way ANOVA integrates two factors, providing deeper insights into interaction effects.
Furthermore, post-hoc tests, like Tukey's HSD, are essential following ANOVA when significant differences are found. These tests pinpoint specific group differences, leading to more detailed conclusions.
Overall, the application of statistical techniques in life sciences facilitates informed decisions based on data analysis. By understanding and applying these methods correctly, researchers contribute to the body of scientific knowledge and advance their respective fields.
Statistical Software in Research
Statistical software plays a crucial role in the life sciences. As research becomes more data-driven, the ability to analyze large sets of information efficiently is vital. Various software programs provide researchers with tools to perform complex statistical analyses, visualize data, and interpret results. The selection of appropriate software can significantly affect the outcome of scientific studies.
The benefits of using statistical software include:
- Efficiency: Automates calculations, saving time over manual methods.
- Accuracy: Reduces the likelihood of human error in data analysis.
- Visualizations: Creates graphs and plots that help in the comprehension of results.
- Reproducibility: Facilitates the sharing of methods, making studies easier to reproduce.
- Support for Complex Analyses: Many statistical methods require advanced mathematical understanding that software can handle.
Given these factors, selecting the right statistical software is an important consideration for researchers. Compatibility with the data type, user experience, and the range of statistical analyses supported are vital aspects in this decision.
Overview of Popular Softwares
There are several popular statistical software programs widely used in life sciences:
- R: A free and open-source programming language, R provides extensive statistical libraries and is favored for its flexibility and breadth of application.
- SAS: Known for its robustness in data management and advanced analytics, SAS is often preferred in clinical trials and large datasets analysis.
- SPSS: A user-friendly software, SPSS has a strong presence in social sciences and health research, making it accessible to non-programmers.
- Python: Increasingly used for data analysis, Python's libraries, like Pandas and NumPy, are gaining popularity in life science research.
- Stata: This software is known for its efficient handling of time-series data and is often used in epidemiological studies.
Each software has unique features, making it suitable for certain research contexts or preferences. Familiarity with one or more of these tools is valuable for students and professionals alike.
Application in Data Analysis
Statistical software is essential for effective data analysis in life sciences. Researchers utilize it for various tasks:
- Data Cleaning: Prepares raw data for analysis, addressing missing values and errors.
- Descriptive Statistics: Computes measures like mean, median, and standard deviation to summarize data characteristics.
- Inferential Statistics: Conducts hypothesis testing and estimations for broader population insights using sample data.
- Regression Analysis: Models relationships between variables, helping to predict outcomes based on trends.
- Graphical Representation: Enhances data presentation through histograms, scatter plots, and other visual aids, aiding in results interpretation.
The application of statistical software aids in ensuring that findings are reliable and actionable. These tools empower researchers to draw meaningful conclusions that advance knowledge in fields like biology, medicine, and environmental science.
"Statistical software not only facilitates complex analyses, but also enhances the overall research validity through rigorous data examination."
In summary, the use of statistical software in life sciences is indispensable. It streamlines the research process, improving efficiency and accuracy, and ultimately contributes to scientific advancement.
Statistical Challenges in Life Sciences
The realm of life sciences is often shaped by intricate statistical methodologies and their respective challenges. Recognizing and addressing these challenges is crucial for the advancement of reliable research outcomes. Accurate conclusions in life sciences rely heavily on robust statistical practices. Ignoring potential pitfalls may compromise the overall integrity of research, leading to questionable findings and unreliable applications in healthcare, environmental policy, and biological insights.
Among the myriad challenges faced, data bias and integrity, as well as the complexities of overfitting and underfitting, stand out as paramount. Both require meticulous attention and a thoughtful approach to ensure that results reflect true phenomena rather than artifacts of flawed data or misapplied methods.
Data Bias and Integrity
Data bias poses a significant threat to the credibility of research findings in life sciences. This bias can arise from various sources, including sampling errors, measurement inaccuracies, or selective reporting. When data collection methods or participant selection are flawed, the results may not be representative of the larger population. Such consequences can lead to erroneous conclusions that skew understanding in fields like epidemiology and pharmacology.
To combat data bias, researchers must adopt rigorous study designs. This includes ensuring that sample populations are adequately diversified and representative of the broader demographic being studied. Moreover, maintaining data integrity is essential. This means implementing robust validation methods, such as cross-checking data entries and utilizing standardized measurement techniques.
Some strategies to ensure data integrity include:
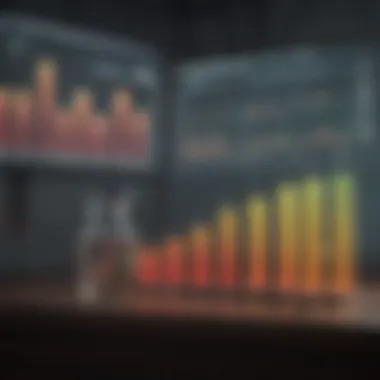
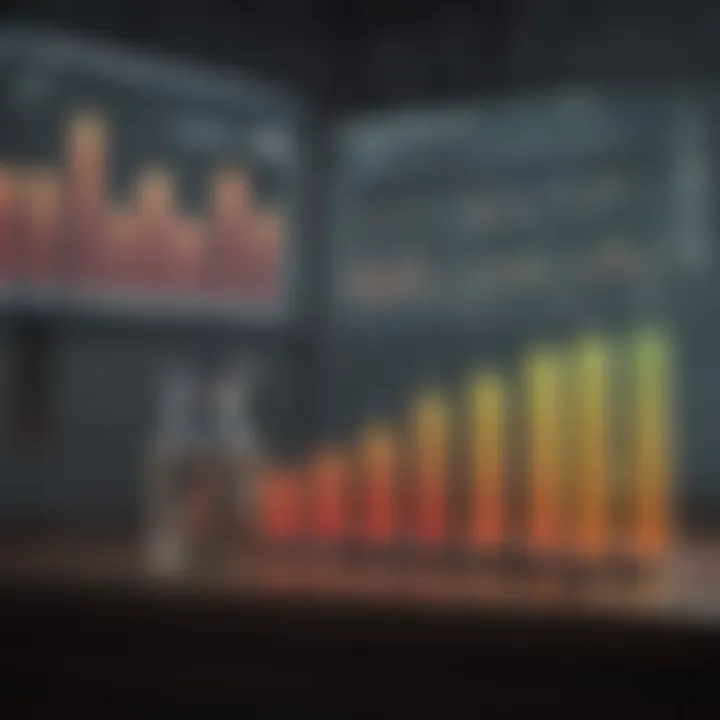
- Rigorous Training: Ensuring that all personnel involved in data collection understand the methods and principles involved.
- Quality Control Measures: Regular audits and checks to confirm the accuracy and reliability of the data collected.
- Transparent Reporting: Providing accessible, comprehensive documentation of data sources and methodologies used in the research process.
"Bias in research can lead to fatal misjudgments, hence why it is essential to recognize potential sources and devise ways to mitigate them."
Overfitting and Underfitting
Overfitting and underfitting are statistical challenges that arise during the modeling process. These issues can impact the ability to generalize findings from a study to the broader population. Overfitting occurs when a model learns noise or random fluctuations in the training data instead of the actual signal. This results in a model that is too complex, capturing details that do not help in making predictions on new data. In contrast, underfitting denotes a model that is too simplistic, failing to capture the underlying trend of the data.
Addressing these issues requires a balance between model complexity and interpretability. The use of techniques such as cross-validation helps to ascertain how well a model performs on unseen data. Moreover, selecting the right statistical methods, as well as employing regularization techniques, can mitigate risks associated with overfitting. These approaches enhance the robustness of predictive models, promoting more reliable conclusions in research.
To summarize, awareness of overfitting and underfitting is crucial for researchers. Regular evaluations and adjustments can enhance model performance and ensure that results are not merely a reflection of data peculiarities.
In the landscape of life science statistics, navigating these challenges with effective strategies leads to a richer understanding of biological data, ultimately benefiting research practices and outcomes.
Case Studies in Life Science Statistics
Case studies in life science statistics illustrate the practical application of statistical methods in real-world scenarios. These studies are vital as they bridge the gap between theoretical knowledge and applied research. They showcase how statistics contribute to critical findings within various domains of life sciences, such as medicine and ecology. Understanding these case studies enriches our grasp of both statistical inference and biological phenomena.
Clinical Trials
Clinical trials stand as a cornerstone of medical research. They involve applying statistical principles to assess the efficacy of new drugs and treatments. The design of these trials is crucial; a poorly designed trial can lead to invalid conclusions. Key statistical considerations include randomization, control groups, and blinding. Each element helps to mitigate biases and ensure the reliability of results.
A well-structured clinical trial typically follows phases:
- Phase I: Focuses on safety and dosage.
- Phase II: Evaluates efficacy and side effects.
- Phase III: Confirms effectiveness on a larger group.
The significance of statistics in this process cannot be overstated. For instance, during data analysis, methods such as hypothesis testing determine whether the treatment shows statistically significant effects compared to a control group. Moreover, power analysis calculates the sample size needed to detect a treatment effect, which is essential for avoiding underpowered studies.
In summary, clinical trials represent a prime example of applied life science statistics, showcasing how statistical rigor informs patient care decisions and regulatory approvals.
Ecological Research
Ecological research relies heavily on statistical methods to understand complex interactions within ecosystems. Researchers utilize statistics to analyze and interpret data related to populations, communities, and environments. This type of research often involves large datasets, making robust statistical approaches necessary to draw meaningful conclusions.
For instance, regression analysis helps to assess relationships between species abundance and environmental factors, such as temperature or rainfall. Additionally, ecological studies frequently employ multivariate techniques to evaluate the impacts of multiple variables simultaneously. This is critical in today's world, where climate change and habitat loss affect biodiversity.
"Statistics allows ecologists to make sense of the variability inherent in natural systems, enabling evidence-based conservation strategies."
Finally, ecological case studies not only highlight statistical applications but also underscore the importance of these methods in shaping policies for conservation and resource management. Through a statistical lens, researchers can advocate for practices that protect endangered species and restore ecosystems.
Future Trends in Life Science Statistics
The landscape of life science statistics is evolving rapidly. Researchers and professionals in the field must stay informed about emerging trends that impact methodologies, tools, and overall outcomes. As technology advances, integrating sophisticated statistical techniques becomes essential. Understanding these trends can shape a researcher’s approach and enhance the quality of scientific investigations. This section will delve into two major components of future trends: machine learning integration and big data analytics, outlining their importance and implications.
Machine Learning Integration
Machine learning is engaginly transforming the field of life science statistics. This integration enhances data analysis capabilities. With machine learning, complex datasets can be processed efficiently. It allows for more accurate predictions and insights. For instance, when dealing with genetic data, machine learning algorithms can uncover patterns that traditional statistical methods might miss.
Moreover, the ability to automate data processing helps minimize human error. This creates a more reliable basis for decision-making in research. In clinical settings, machine learning models assist in predicting patient outcomes, optimizing treatment plans based on historical data. Thus, embracing machine learning is becoming indispensable in life science statistics.
Benefits of machine learning integration include:
- Enhanced analysis speed: Processing large datasets much faster than traditional methods.
- Improved prediction accuracy: More reliable forecasts based on past trends.
- Automation: Reducing manual intervention in data processing.
However, challenges exist. Understanding model interpretation and the risk of overfitting can complicate results. Knowledgeable statisticians must navigate these issues to leverage machine learning effectively.
Big Data Analytics
Another trend gaining traction in life sciences is big data analytics. The massive volume of biological, medical, and environmental data available today calls for advanced analytical methods. Big data provides opportunities to understand complex biological phenomena at unprecedented scales. The insights derived from big data can lead to groundbreaking discoveries.
Utilizing big data analytics enables researchers to:
- Identify correlations: Detect relationships between variables that were not apparent before.
- Monitor trends: Capture real-time data trends over wide ranges of parameters.
- Personalize medicine: Tailor treatments to individuals based on comprehensive patient data analysis.
The intersection of big data and life science statistics generates fascinating possibilities. For example, analyzing large-scale clinical trial data can improve drug development efficiencies. However, it also raises questions about data privacy, security, and ethical considerations. Researchers must balance capitalizing on big data’s potential with responsibility in handling personal information.
"The integration of machine learning and big data analytics in life science statistics will lead to an unprecedented level of understanding and insight in the scientific community."
Culmination
The conclusion of this article serves a critical function in encapsulating the overall essence of life science statistics and its multifaceted implications. Stats in life science is more than a tool; it is an essential foundation upon which sound research is constructed. Through this article, we have traversed the landscape of various statistical methods and principles that underlie research in biology, medicine, and environmental science.
Summary of Key Points
In summarizing key points, a few essential aspects stand out:
- Importance of Statistics: Statistics provide clarity and precision in the interpretation of complex biological data. They allow researchers to draw informed conclusions from their experiments and observational studies.
- Diverse Techniques: The range of statistical techniques discussed, including hypothesis testing and regression analysis, plays a significant role in diverse scientific inquiries. These methods are vital for validating findings and supporting hypotheses.
- Challenges Facing Researchers: We also touched on challenges, like data bias and the risks of overfitting or underfitting models. These factors can significantly influence the integrity and reliability of research outcomes.
- Future Directions: As life sciences evolve, integrating modern statistical practices, such as machine learning and big data analytics, will enhance research capabilities and efficiency.
Overall, the concluding remarks highlight that a robust understanding of statistical methods is not only beneficial but necessary for anyone engaged in life sciences. This knowledge empowers individuals to navigate the complexities of data analysis with confidence, fostering more reliable scientific discovery.