Scientific Data Management: Principles and Innovations
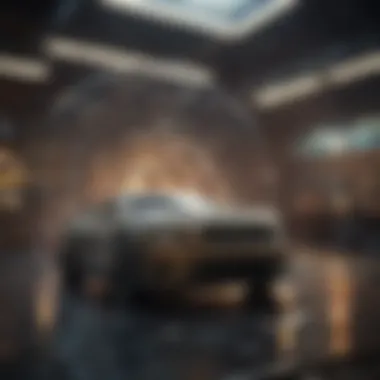
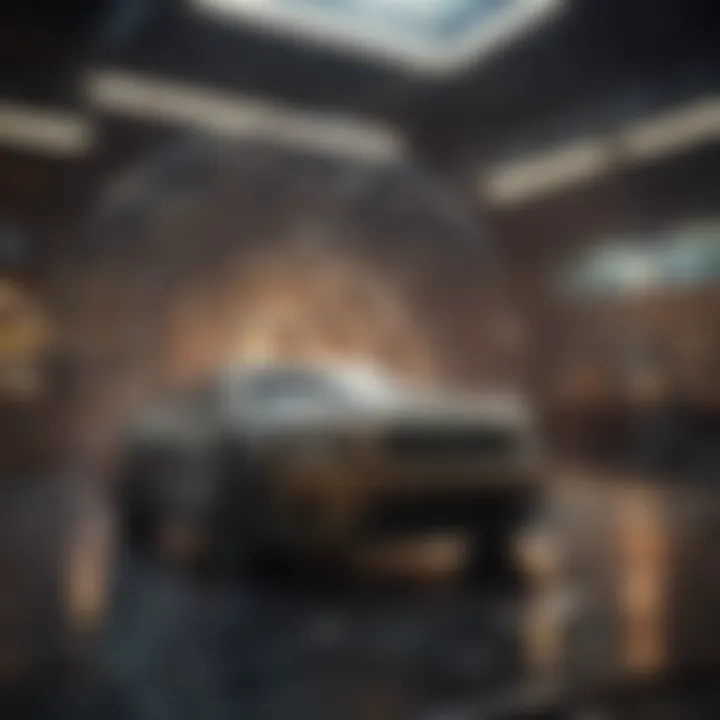
Intro
Scientific data management is a crucial area of focus in modern research. As the volume of scientific data continues to grow, effective management becomes increasingly vital. This article will unpack essential principles and practices in the field, delving into how these approaches enhance reliability and accessibility in research.
Understanding the complexities of scientific data management involves dissecting various methodologies used in this domain. From data collection to storage, every aspect is paramount in ensuring that scientists can confidently share and reproduce their findings.
Moreover, this discourse will highlight the ethical considerations that govern data practices. Ethical data governance not only fosters trust among researchers but also promotes transparency within the scientific community. By addressing current challenges and emerging technologies, we aim to provide a comprehensive overview that serves as a guide for professionals in the field.
Methodology
Study Design
An effective study design should clearly outline how data will be managed throughout the research process. This includes specifying protocols for data collection, storage, and dissemination. A blueprint that incorporates best practices enables researchers to ensure integrity and accessibility in their findings.
Data Collection Techniques
Data collection is the cornerstone of any scientific inquiry. Employing a variety of techniques ensures comprehensive data gathering. Some common methods include:
- Surveys and Questionnaires: Useful for collecting qualitative data from diverse audiences.
- Experiments: Controlled settings help yield quantitative data, allowing for statistical analysis.
- Observational Studies: Collecting data through observation can provide rich insights, particularly in ecological and social sciences.
- Secondary Data Analysis: Using already existing data can save time and resources, with careful consideration of the data's context and credibility.
Regardless of the chosen method, maintaining meticulous records is essential.
Discussion
Interpretation of Results
Once data has been collected, interpreting results is critical. This involves analyzing the data in the context of the hypotheses posed at the study's outset. Clear interpretation can reveal trends, correlations, and potential causations.
Limitations of the Study
It is important to acknowledge limitations. Factors such as sample size, data quality, and methodological constraints can influence results. Being transparent about these limitations can inform future research and guide others on how to refine their approaches.
Future Research Directions
The landscape of scientific data management is not static. Continuous evolution in technology and methodology presents new opportunities. Future research could explore:
- Integration of artificial intelligence in data analysis.
- Development of tools for enhanced data sharing platforms.
- Establishing standardized protocols across different fields to ensure cross-compatibility of data.
"Data management is not a hindrance, but a foundational element of scientific progress."
Foreword to Scientific Data Management
Effective data management is fundamental to scientific research. This introduction will lay the groundwork for understanding the significance of scientific data management. As the quantity of data generated in research increases, the need for robust management practices becomes more pressing. Poorly managed data can lead to lost insights, wasted resources, and ultimately hinder the progress of scientific discovery.
Scientific data management encompasses the planning, organization, and handling of data throughout its lifecycle. This ensures that researchers can easily access reliable data and make informed decisions. A clear understanding of the principles and practices in this field can enhance research outcomes.
Definition and Scope
Scientific data management refers to a set of practices and processes used to effectively handle data generated during research. This includes data collection, storage, sharing, and preservation. The scope is vast, involving various disciplines and methodologies tailored to specific requirements. It can incorporate elements such as metadata management, data documentation, and compliance with standards.
The realm of scientific data management is not static. It evolves alongside advancements in technology and the changing landscape of research itself. Researchers must be aware of the tools and techniques available for effective management. Moreover, these methods ensure that data remains accessible and reliable over time.
Importance of Data Management in Scientific Research
Data management is critical in scientific research for numerous reasons. Firstly, it promotes data integrity, ensuring that data is accurate, consistent, and trustworthy. Without proper management, data can become corrupted or misinterpreted. This can lead to unreliable conclusions and potentially derail research efforts.
Secondly, effective data management fosters collaborative research. When data is well-organized and documented, it becomes easier to share with other researchers. This transparency is vital for advancing knowledge in various fields. Promoting a culture of sharing and collaboration can significantly enhance research outcomes.
Data transparency enhances trust in research findings and promotes a collaborative environment.
In addition, robust data management practices are essential for compliance with regulatory requirements. Many funding agencies and organizations require adherence to specific data management plans. Researchers must understand these obligations to avoid potential funding issues.
Lastly, a focus on data management facilitates better long-term data preservation. As research progresses, having organized data archives ensures that valuable information remains accessible for future research initiatives. This, in turn, enriches the scientific community and aids in the cumulative nature of knowledge.
Fundamental Principles of Scientific Data Management
Scientific data management is crucial, providing a framework for organizing, storing, and sharing research data. Understanding fundamental principles is essential for ensuring that data is reliable and accessible. This section will discuss three key principles: Data Integrity, Data Stewardship, and Data Lifecycle Management. Together, these principles establish a strong foundation for effective data management practices in research.
Data Integrity
Data integrity refers to the accuracy and consistency of data over its lifespan. It is the bedrock of scientific research, as it ensures that researchers can trust the data they use and share. Without data integrity, research findings could become suspect, impacting the broader scientific community.
Maintaining data integrity involves several practices:
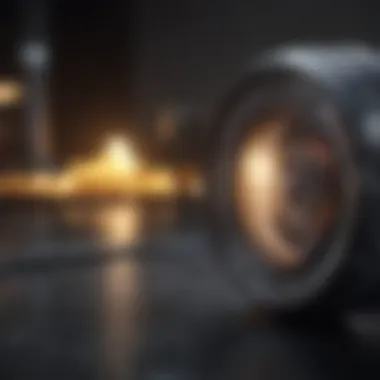
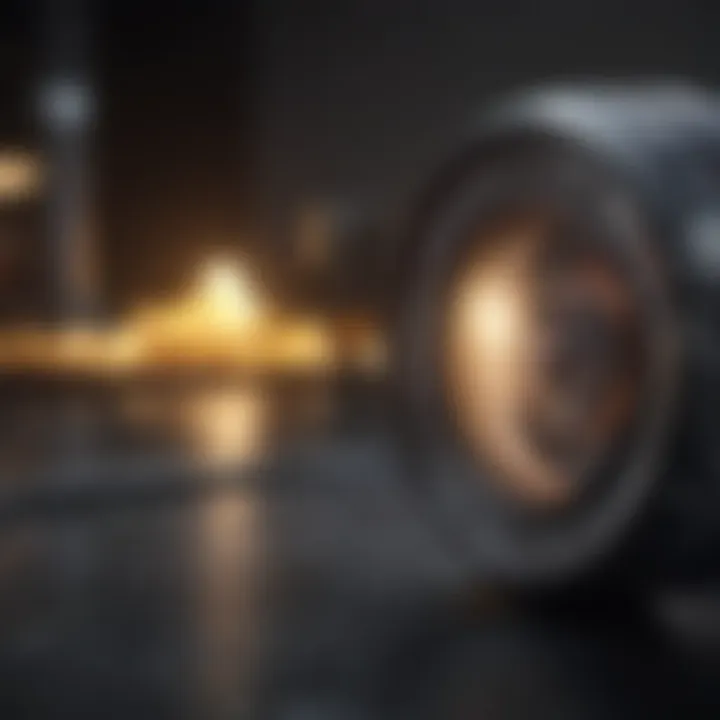
- Validation of Data: Ensure that data is collected methodically, following established protocols. This validation minimizes errors and enhances reliability.
- Version Control: Using version control systems helps track changes over time. This practice allows researchers to revert to previous versions if necessary.
- Access Controls: Limiting who can modify data helps preserve its integrity. Only authorized personnel should have the ability to alter datasets.
Ultimately, ensuring data integrity builds trust among researchers and stakeholders. If integrity is compromised, it can lead to flawed conclusions and waste resources.
Data Stewardship
Data stewardship is about responsibly managing data throughout its lifecycle. This principle emphasizes the role of individuals or teams who oversee data management activities, ensuring ethical usage, proper documentation, and compliance with regulations.
Key aspects of data stewardship include:
- Documentation: Maintaining clear records of data sources, collection methods, and processing steps is essential. Thorough documentation aids in transparency and reproducibility.
- Ethical Usage: Adhering to ethical guidelines is critical. This means respecting participant confidentiality and ensuring consent for using personal data.
- Collaboration: Fostering collaboration among researchers encourages shared stewardship. When teams work together, they can leverage each other’s expertise in managing data.
Effective data stewardship leads to better decision-making and promotes a culture of responsible research. It encourages a holistic view of data management as part of broader scientific responsibilities.
Data Lifecycle Management
Data lifecycle management encompasses the processes involved in managing data from its creation to its deletion. This principle recognizes that data has a finite life and must be handled appropriately at every stage.
Some important stages in data lifecycle management are:
- Data Creation: This is the initial phase, where data is generated. Clear guidelines on how data should be collected should be established from the start.
- Data Storage: Secure and organized storage solutions are needed. This may include cloud storage options that offer scalability and reliability.
- Data Sharing: Researchers need to establish protocols for sharing data with collaborators and the public. Open data practices can enhance scientific progress but require careful planning.
- Data Archiving and Disposal: Finally, archiving data for future use, or responsibly deleting it when no longer needed, are important to ensure data remains relevant and manageable.
The focus on data lifecycle management helps researchers be more proactive with their data, ensuring it is not only preserved but also made available for long-term research initiatives.
The principles of scientific data management are interwoven, guiding researchers to uphold the integrity, stewardship, and lifecycle processes essential for reliability and accessibility in scientific inquiry.
Methods and Frameworks for Effective Data Management
Effective data management forms the backbone of scientific research, providing a structured approach to managing data throughout its lifecycle. The methods and frameworks developed for this purpose influence how data is collected, stored, analyzed, and shared. These frameworks help researchers establish consistency and promote best practices, enhancing the overall quality and reliability of scientific data.
Data Management Plans
A Data Management Plan (DMP) is a formal document that outlines how data will be handled during a research project. DMPs are crucial for anticipating data-related challenges and ensuring compliance with institutional and funding requirements. They typically include elements such as data description, metadata standards, data sharing strategies, and long-term preservation methods.
- Importance of DMPs:
- They provide a clear roadmap for researchers.
- They facilitate communication between team members and stakeholders.
- They enhance funding applications by demonstrating diligence in data management.
For example, an effective DMP addresses how data will be collected, organized, and archived. It specifies the use of tools for documentation and the responsibilities assigned to different team members.
Standard Operating Procedures
Standard Operating Procedures (SOPs) are essential in data management as they standardize processes across different research teams. By having SOPs in place, organizations ensure that data handling practices are consistent, reproducible, and compliant with regulatory standards. This prevents variations that can compromise data quality and integrity.
- Key Features of SOPs:
- They offer detailed instructions on data collection methods, storage solutions, and data analysis protocols.
- They help in training new members, ensuring everyone follows the same guidelines.
- They mitigate risks by establishing a clear course of action during data-related incidents.
SOPs should be reviewed regularly and updated as necessary to adapt to changing technologies and research conditions.
Data Standards and Protocols
Data standards and protocols provide a framework for ensuring interoperability and consistency in data. They set guidelines for how data should be formatted, described, and shared. Employing recognized standards enhances data usability and helps facilitate data sharing across different platforms.
- Benefits of Adhering to Standards:
- They ensure that data from multiple sources can work together seamlessly.
- They reduce the risks associated with data misinterpretation.
- They support compliance with legal and ethical obligations.
Commonly accepted standards include the Dublin Core Metadata Standard and the ISO 19115 for geospatial data. Following data protocols is particularly important in fields that rely on collaborative research and data sharing.
"Implementing robust methods and frameworks for data management not only safeguards the integrity of scientific research but also enhances collaborative opportunities and the potential for new discoveries."
In sum, focusing on methods and frameworks for effective data management creates a foundation for successful research endeavors. It addresses compliance, consistency, and quality, all of which are essential for advancing scientific knowledge in a responsible manner.
Technological Innovations in Data Management
Technological innovations are transforming the landscape of scientific data management. They enable researchers to manage vast amounts of data more efficiently while enhancing accessibility and security. This section examines three key innovations: cloud computing solutions, big data analytics, and machine learning applications. Each plays a crucial role in optimizing data management strategies in contemporary research environments.
Cloud Computing Solutions
Cloud computing has emerged as a pivotal tool for scientific data management. It allows researchers to store and access data remotely, fostering collaboration across geographic boundaries. The benefits of cloud computing include:
- Scalability: Researchers can easily scale storage up or down according to their needs, avoiding the costs associated with physical storage infrastructure.
- Accessibility: Data can be accessed from anywhere at any time, given an Internet connection. This facilitates greater collaboration among researchers.
- Cost-efficiency: Many cloud services operate on a pay-as-you-go model, which can save organizations money on data management expenses.
However, there are considerations regarding data security and compliance with regulations such as the General Data Protection Regulation (GDPR). Researchers must ensure that their cloud services adhere to strict privacy and security protocols.
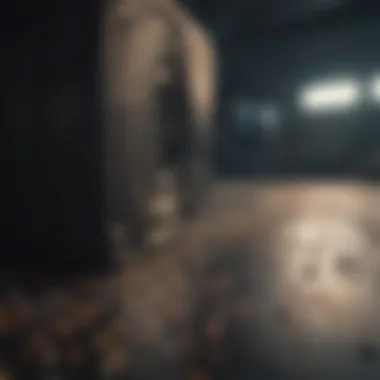
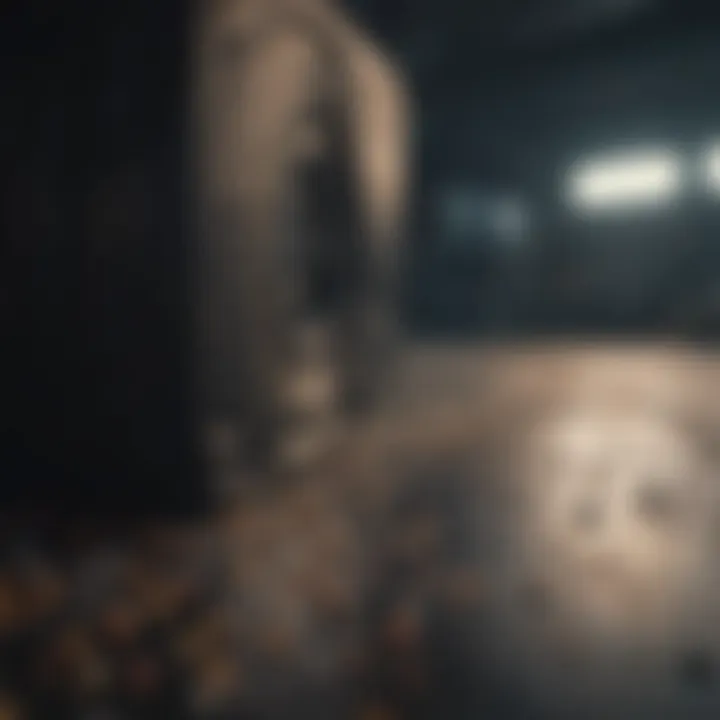
Big Data Analytics
Big data analytics is another technological innovation enhancing scientific data management. This involves analyzing large, complex datasets to extract meaningful insights. Benefits of big data analytics include:
- Improved Decision-Making: By analyzing large volumes of data, researchers can draw conclusions that may not be apparent from smaller datasets. This leads to more informed decision-making in research and policy.
- Identifying Patterns: Advanced analytical techniques can uncover trends that help in predicting future outcomes, particularly in fields like healthcare and environmental research.
- Enhanced Research Quality: With the ability to analyze large datasets, researchers can improve the reliability and reproducibility of their findings by validating results across diversified datasets.
It is essential to have appropriate tools and skills to leverage big data analytics effectively. Not all researchers may have the training to navigate such technologies, which can be a barrier to integration.
Machine Learning Applications
Machine learning applications are gaining traction in scientific data management. These systems utilize algorithms to learn from data patterns and make predictions or classifications. The significance of machine learning lies in:
- Automating Data Processing: Machine learning can automate routine data processing tasks, saving researchers time and effort. This allows them to focus on more complex analytical tasks.
- Predictive Analytics: Researchers can use machine learning to predict outcomes based on historical data, which is invaluable in fields like pharmaceuticals and climate science.
- Anomaly Detection: Machine learning algorithms can identify outliers or anomalies in data, which is critical in maintaining data integrity and reliability.
While machine learning holds promise, it also raises questions about the transparency of algorithms and the need for rigorous validation of models to avoid bias.
"Technological innovations like cloud computing, big data analytics, and machine learning are not just tools but essential pillars in the realm of scientific data management, guiding researchers towards efficiency and enhanced credibility in their findings."
Challenges in Scientific Data Management
Scientific data management faces numerous challenges that can impact research efficiency and the overall integrity of scientific endeavors. Understanding these challenges is essential for researchers and professionals in the field. This section highlights significant difficulties such as data security and privacy concerns, barriers to data sharing, and issues with reproducibility. Each challenge has unique implications for the management of scientific data.
Data Security and Privacy Concerns
Data security is a critical aspect of scientific data management. Researchers must safeguard their data against unauthorized access, data breaches, and cyber threats. Sensitive data, particularly in fields like healthcare and environmental studies, often involves personal information that requires stringent protection measures.
To combat these issues, several strategies can be adopted:
- Encryption: Data encryption ensures that even if unauthorized access occurs, the data remains unreadable.
- Access Control: Limiting access to data only to authorized individuals reduces the risk of data misuse.
- Regular Security Audits: Conducting routine reviews of security protocols helps identify vulnerabilities.
Researchers must also comply with regulations such as the General Data Protection Regulation (GDPR) that demands robust data protection standards. Failure to comply can lead to legal repercussions and loss of trust among stakeholders.
Data Sharing Barriers
Data sharing is vital in scientific research, yet many obstacles impede this practice. One major barrier includes disagreements over authorship and ownership of data. Researchers may be reluctant to share their data if it undermines their competitive advantage or intellectual property rights. Furthermore, institutions often have policies that complicate data sharing.
Other barriers to consider are:
- Technical Standards: Differences in data formats and standards can hinder effective sharing.
- Lack of Infrastructure: Not all researchers have access to platforms designed for data sharing, particularly in less funded institutions.
Addressing these barriers is crucial for promoting a collaborative research environment. Encouraging data sharing can enhance transparency and facilitate advancements in various scientific fields.
Reproducibility Issues
Reproducibility is a cornerstone of scientific validity yet remains a pervasive challenge. Many studies fail to provide enough detail for others to replicate results reliably. Factors contributing to this challenge include:
- Incomplete Reporting: Oftentimes, researchers don’t publish all relevant methods, which prevents others from reproducing experiments properly.
- Data Management Practices: Poor data organization and documentation can lead to loss of critical information over time.
- Variation in Conditions: Slight changes in experimental conditions can lead to different outcomes, complicating reproducibility.
To improve reproducibility, scientists can utilize standardized methodologies and improve documentation practices. Additionally, promoting a culture of transparency can significantly benefit the scientific community.
"Enhancing scientific data management practices can lead to more reliable research outcomes and a stronger foundation for future scientific inquiry."
Ethical Considerations in Data Management
In the realm of scientific research, ethical considerations play a pivotal role in data management. These considerations ensure that research is conducted responsibly, promoting trust and integrity in the scientific community. By establishing clear ethical guidelines for data management, researchers can address potential risks and safeguard participant welfare, aligning their work with broader societal values.
Research Ethics and Compliance
Research ethics focus on the principles guiding researchers in their data management practices. Compliance with established ethical norms not only protects individual rights but also enhances the credibility of the research outcomes. This approach encompasses several key elements:
- Respect for Persons: This principle underlines the necessity to acknowledge autonomy and protect those with diminished autonomy. Researchers must ensure that participants are aware of how their data will be used and stored.
- Beneficence: Researchers should strive to maximize benefits and minimize any potential harm. Proper data management practices contribute to this by ensuring that sensitive information is handled with care.
- Justice: Fair distribution of the benefits and burdens of research is crucial. It involves being equitable in the selection of research subjects and ensuring that no group bears an undue burden.
Researchers must comply with institutional review boards (IRBs) or ethics committees, which rigorously evaluate research proposals. These bodies exert significant influence in enforcing ethical standards, insisting on fact-based assessments on participants’ vulnerability and the potential risks involved in handling data.
"Adhering to ethical standards is not merely a legal obligation but a moral imperative that enhances the legitimacy of scientific inquiry."
Informed Consent in Data Usage
Informed consent is an aspect of ethical data management that allows individuals to make informed decisions about their participation and the use of their data. This process involves several important components:
- Information Disclosure: Participants must receive clear, comprehensive, and accessible information about the study, including how their data will be collected, stored, and used. The clarity of this information directly impacts participants’ understanding and their ability to make informed decisions.
- Voluntariness: Consent must be given freely, without any coercion. Participants should be made aware that their involvement is optional and that their decision to withdraw at any time is respected.
- Competence: Researchers should evaluate the capacity of individuals to understand the information provided. This is particularly essential when engaging with vulnerable populations.
- Ongoing Process: Informed consent is not a one-time act but a continuous process. Researchers should facilitate ongoing communication regarding changes in data usage and address participants’ questions throughout the study.
Ensuring informed consent leads to trust between researchers and participants. When individuals understand their rights and the implications of their participation, they are more likely to engage meaningfully in the research process. This, in turn, fosters a culture of respect, transparency, and ethical responsibility across the scientific community.
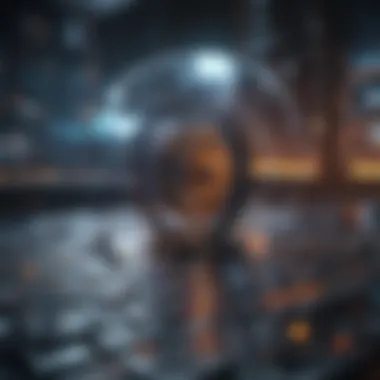
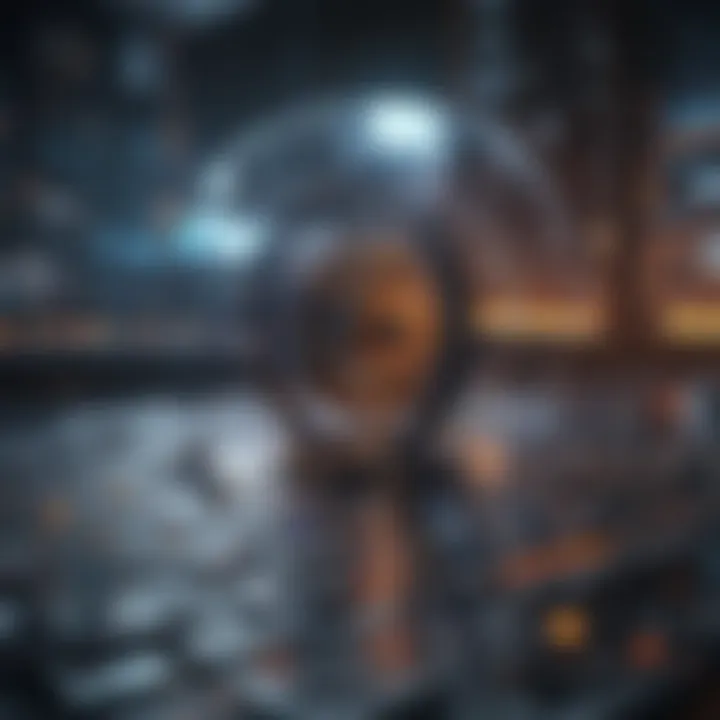
Best Practices for Data Preservation
Effective data preservation is a cornerstone of scientific data management. It ensures that valuable research data remains accessible, intact, and reliable over time. This is increasingly important as scientific inquiry and collaboration expand in scope and complexity. By following best practices for data preservation, researchers enhance the longevity of their work and support reproducibility, which is crucial for validation and further study.
Long-term Storage Solutions
Long-term storage is essential for safeguarding scientific data against loss due to technical failures, environmental conditions, and human errors. Researchers should consider various storage options when planning their data management strategies.
- Cloud Storage: Services like Amazon Web Services, Google Cloud, and Microsoft Azure provide scalable solutions tailored for data durability and accessibility. These platforms offer redundancies that protect data from accidental deletions and hardware failures.
- Institutional Repositories: Many universities and research institutions maintain repositories that support data sharing. Utilizing these services not only aids in compliance with funding agency requirements but also ensures that data is preserved in a stable environment.
- Data Archiving Services: Entities such as Dryad and figshare are designed specifically for long-term archival of research datasets. These services promote discoverability and provide proper citation structures for datasets.
Considerations for long-term storage must also include:
- Regular Audit and Assessment: Continuously checking the integrity of stored data is critical. Schedule regular audits to ensure all data is accessible and complete.
- Data Formats: Use open and widely accepted formats for data storage. This choice protects against obsolescence and ensures future usability.
Regular Data Backups
Having a reliable backup strategy is vital to mitigate data loss risks. Regular backups not only secure data but also facilitate recovery in case of unexpected events.
- Automated Backup Systems: Implement systems that automatically perform backups at predefined intervals. This reduces the likelihood of human error and ensures that the most current data versions are stored.
- Off-Site Backup Locations: Storing backups in a different geographic location protects data from local disasters. Options include external hard drives, secure network drives, or remote cloud-based services.
- Testing Recovery Procedures: Regularly test the backup recovery process to confirm that data can be restored successfully. This can reveal potential issues before a crisis occurs.
"Data loss can cripple research efforts, highlighting the need for rigorous backup strategies."
Following these practices for data preservation will result in enhanced trust among researchers and stakeholders. This approach promotes the responsible management of scientific data, ultimately contributing to the integrity of research and collaborative efforts across disciplines.
Case Studies of Effective Data Management
Case studies in data management offer unique insights into real-world applications and outcomes of data strategies. They serve as a foundation to understand how theoretical principles translate into practice. A well-documented case study can illustrate the challenges faced, the solutions implemented, and the overall impact achieved. Through these examples, we can identify best practices and areas for improvement, ultimately enhancing the effectiveness of scientific data management across various domains.
Successful Implementation in Academia
In academia, data management is increasingly recognized as a critical component of research integrity. Institutions like Harvard University have developed robust data management frameworks that ensure compliance with funding agency requirements. This includes creating data management plans that outline how data will be collected, processed, preserved, and shared.
A notable example is the implementation of the Research Data Management (RDM) service at the University of Edinburgh. This initiative allows researchers to consult with data management experts to improve their data handling processes. Effective RDM training programs have also been instituted, emphasizing best practices for storing and sharing data. Such structured approaches help mitigate risks of data loss and improve accessibility.
In addition, collaborations among different departments have demonstrated the impact of centralized data management efforts. For instance, when various research teams at Stanford University unified their data governance protocols, they noticed significant improvements in data consistency and quality. Researchers benefited from shared resources and reduced duplication of effort, demonstrating the value of coordinated data management in an academic setting.
Industry Examples
On the industrial front, companies are increasingly leveraging data management practices to drive business decisions and innovation. One strong case is that of IBM, which has developed its own data governance framework to manage the vast amounts of data generated in its operations. The company employs machine learning algorithms coupled with strict data governance measures to maintain data integrity and compliance with regulations. This has allowed them to use their data as a strategic asset, improving decision-making processes and enhancing customer insights.
Similarly, Pfizer exemplifies effective data management within the pharmaceutical industry. The company initiated a data strategy that emphasizes the integration of data from clinical trials with real-world evidence. By employing advanced analytics and ensuring efficient data flow across departments, Pfizer successfully improved its drug development lifecycle. This case underscores the competitive advantage gained through meticulous data management practices in a data-intensive field.
"The implementation of effective data management strategies is no longer optional; it is essential for organizations striving to remain competitive and innovative in today’s data-driven world."
These case studies, both from academia and industry, highlight the diverse applications and successful outcomes derived from thoughtful data management practices. They enrich the understanding of best practices while inspiring ongoing improvements in processes related to scientific data management.
Future Directions in Scientific Data Management
The landscape of scientific data management is continuously evolving. Recognizing future directions is critical for researchers, educators, and professionals aiming to enhance their data practices. Anticipating these trends not only fosters innovation but also ensures that data management systems remain robust and responsive to emerging challenges. As the volume and complexity of data grow, the reliance on effective data management becomes more evident. The aim is to improve data sharing, accessibility, and collaboration across disciplines.
Emerging Trends
Several trends are shaping the future of scientific data management.
- Open Data Initiatives: These initiatives promote transparency and accessibility. Researchers are increasingly encouraged to share their datasets openly, enabling others to validate findings and foster collaborative research.
- Data Visualization Tools: Easy-to-understand visual presentations of complex data sets are gaining traction. These tools help to convey findings clearly and make the data more accessible to a broader audience.
- Interdisciplinary Collaboration: Scientists from various fields are working together, creating demands for interoperable data systems. This trend emphasizes the need for universal standards and protocols.
- Data Quality and Standardization: There is an increasing focus on ensuring high data quality. Standardized formats make it easier to share and compare data across studies, reducing inconsistencies.
These trends are not only reshaping how data is managed, but also influencing the broader research environment.
The Role of AI and Automation
Artificial Intelligence (AI) and automation are becoming vital in data management for several reasons. These technologies can handle large datasets more efficiently than manual processes. They streamline data collection, analysis, and storage, allowing researchers to focus on interpreting results rather than managing data. Some key aspects of AI and automation in this context include:
- Automated Data Cleaning: AI algorithms can identify and correct errors in datasets. This process ensures higher data integrity, crucial for valid research findings.
- Predictive Analytics: Machine learning models can analyze historical data to make forecasts. This capability supports decision-making in research design and helps identify potential gaps in current studies.
- Enhanced Security: AI-driven security measures can detect anomalies in data access. This ensures better protection against breaches, thereby addressing data privacy concerns.
- Resource Optimization: Automating routine tasks reduces the administrative burden on researchers. This efficiency translates into more time available for critical analysis.
The integration of AI and automation not only improves the effectiveness of data management processes but also opens new possibilities for innovative research applications.
In summary, the evolving landscape of scientific data management presents both challenges and opportunities. By staying ahead of emerging trends and leveraging AI and automation, researchers can ensure that their data practices are effective and forward-thinking.
The End
The conclusion of this article serves to reinforce the significance of scientific data management in the realm of research. Well-managed data is not just an operational necessity; it is a foundation upon which credible findings are formulated. Hence, effective scientific data management enhances the reliability and accessibility of data, which in turn supports the validity of research outcomes.
Summarizing Key Takeaways
In this section, we recap crucial insights from our exploration of scientific data management. Key elements include:
- Data Integrity: Ensures accuracy and reliability of data throughout its lifecycle.
- Ethical Considerations: Compliance with regulations that govern data usage and sharing is paramount.
- Technological Innovations: The evolution of tools and methods such as cloud computing and big data analytics are reshaping the landscape of data management.
- Best Practices: Adopting standardized protocols is essential for long-term data preservation and integrity.
It is evident that these points illustrate the complexities faced by researchers in managing data while navigating privacy issues, compliance requirements, and technological changes. Efficient data management is critical not only for improving research quality but also for fostering collaboration across disciplines.
The Path Forward for Researchers
Looking ahead, researchers must embrace emerging trends and advancements in data management. It calls for continuous education and adaptation to new tools and practices that ensure data integrity and ethical compliance. Also, understanding data sharing protocols can lead to greater accessibility and utilization of research outputs.
A paradigm shift towards automation and artificial intelligence applications is inevitable. By staying updated with these innovations, researchers can enhance their capabilities and productivity. Overall, the commitment to refining data management strategies will undoubtedly elevate the impact of scientific research in the future.