A Comprehensive Approach to Image Analysis in Scientific Research
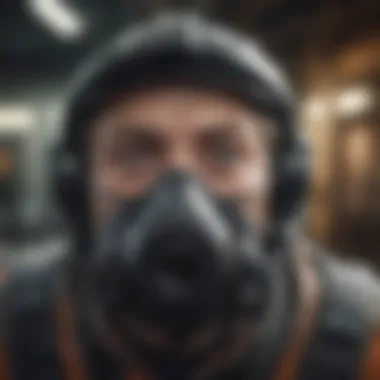
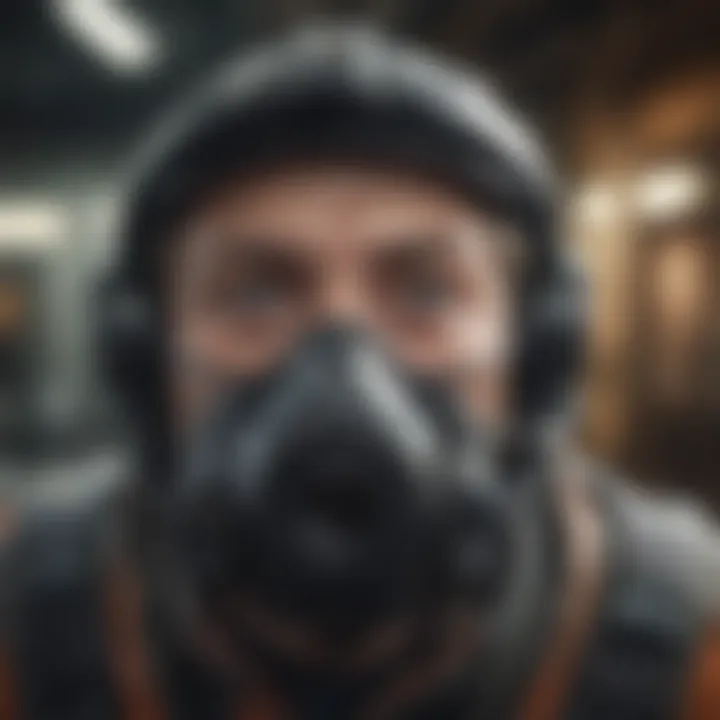
Intro
In the realm of scientific research, image analysis has become a pivotal component for understanding complex phenomena. This article scrutinizes the varied methodologies and tools employed in this critical domain. The objective is to provide a clear guide for students, educators, and professionals who aim to enhance their understanding of image analysis.
Research in various scientific disciplines, from biology to astronomy, often relies on visual data to draw conclusions and advance knowledge. However, the process of image analysis is not merely about capturing photos; it's about extracting meaningful information from these images. This article specifically addresses this need, covering essential techniques and methodologies while exploring their applications, challenges, and future directions.
Methodology
Study Design
A comprehensive approach to image analysis necessitates a robust study design. Researchers must first define their research question clearly. From there, they can determine which imaging techniques will best suit their needs. Common designs include experimental setups, observational studies, and retrospective analyses. Each design varies in its approach and intended outcomes. For example, in biomedical research, experimental designs might involve controlled environments where variables can be manipulated to observe effects in images.
Data Collection Techniques
Data collection is a crucial step in any research. In image analysis, techniques vary widely depending on the field of study. Typical methods include:
- Microscopy: This method allows for detailed examination of biological samples at the cellular level. Optical microscopes, electron microscopes, and fluorescence imaging are common tools employed in this area.
- Remote Sensing: Often used in geology and environmental studies, remote sensing utilizes satellite images to provide a broader context of phenomena occurring on Earth's surface.
- Photography: Simple yet effective, standard photography is often used in studies where capturing moments in time is essential, such as in behavior analysis in ethology.
These techniques must be chosen based on the research goals, available resources, and the types of analysis that will be conducted post-capture.
Discussion
Interpretation of Results
Interpreting results from image analysis requires a deep understanding of both the tools used and the data derived. Analyzing images often involves software that uses algorithms for feature extraction and comparison. The clarity and accuracy of these interpretations can dramatically influence the outcomes of a study. Thus, researchers must remain vigilant about methodological constraints.
Limitations of the Study
Like any scientific approach, image analysis is not without limitations. These may include problems with resolution, artifacts introduced during imaging, or biases introduced by the researcher or tools used. Researchers need to acknowledge these limitations clearly in their findings. Awareness of such challenges fosters a more rounded understanding of the results presented.
Future Research Directions
The landscape of image analysis is evolving swiftly, driven by advancements in technology and machine learning. Future research may include:
- The integration of artificial intelligence for more precise analysis.
- Development of higher-resolution imaging techniques that enable better data capture.
- Exploration of new application areas, such as personalized medicine or advanced materials science.
"As image analysis techniques advance, the possibilities for research applications continue to expand, pushing the boundaries of what we can discover."
By analyzing these components collectively, this article aims to impart substantial knowledge regarding image analysis. For those deep in their academic pursuits or professional investigations, understanding these methodologies is crucial in advancing their work.
Preface to Image Analysis
Image analysis plays a crucial role in scientific research. It provides methods and techniques for interpreting visual data across various disciplines. The importance of image analysis cannot be overstated, as it enhances our understanding of complex subjects. This process allows researchers to quantify information and extract meaningful insights from images, which would otherwise remain hidden in visual data.
Definition and Importance
Image analysis refers to the extraction of information from images using various mathematical and computational techniques. This can involve simple measurements of size and shape or complex evaluations using artificial intelligence. The significance of image analysis lies in its ability to interpret vast quantities of data efficiently. In many scientific fields, such as biology, chemistry, and earth sciences, visual data can hold the key to critical discoveries and validations of theories. By employing image analysis, researchers can facilitate data-driven conclusions rather than relying purely on subjective observations.
Moreover, accurate image analysis can lead to improved diagnostic methods in medical research, more effective material characterizations in chemistry, and enhanced geological predictions in earth sciences. The integration of software tools and techniques further amplifies its applicability, providing robustness and scalability to the research process.
Historical Development
The history of image analysis traces back to the advancement of imaging techniques and computational technologies. Initially, early optical devices enabled scientists to examine specimens closely, but the methods were primarily qualitative. With the development of digital imaging technologies in the latter half of the 20th century, researchers began utilizing computers to digitize images for more rigorous analysis.
In the 1980s, image processing started to gain popularity. Software such as ImageJ and MATLAB emerged to provide tools for analyzing visual data. These advancements continued into the 21st century with the integration of machine learning. Today, various sectors employ algorithms that automate the image analysis process, constantly improving accuracy and efficiency.
The historical journey of image analysis highlights its evolution from basic manual interpretations to sophisticated automated systems, revolutionizing the way scientists conduct research.
Fundamentals of Image Acquisition
The fundamentals of image acquisition serve as the foundation of effective image analysis in scientific research. This involves understanding various imaging modalities and capture techniques that are crucial for obtaining high-quality images necessary for analysis. Proper acquisition directly impacts the subsequent processing and interpretation of images. Without a solid grasp of these elements, the utility of the data gathered can be severely diminished.
Types of Imaging Modalities
Optical Imaging
Optical imaging is a widely employed imaging modality characterized by the use of light to capture images. It is particularly beneficial for its ease of use and relatively low cost. This technique allows researchers to visualize samples in real-time, making it essential for applications such as biological studies. A key characteristic of optical imaging is its non-invasiveness, which preserves sample integrity. However, it is limited by resolution factors that may hinder detailed analysis at the micro or nanoscale, impacting its effectiveness in advanced research contexts.
Electron Microscopy
Electron microscopy provides high-resolution images by using electrons instead of light. This modality is critical for revealing fine structural details of specimens, making it invaluable in materials science and biology. The key characteristic of electron microscopy is its ability to achieve much greater magnifications than optical imaging. This power enables researchers to observe structures as small as a few nanometers. However, it requires extensive sample preparation and can alter the sample due to the high-energy electrons used, which can be a drawback in some studies.
X-ray Imaging
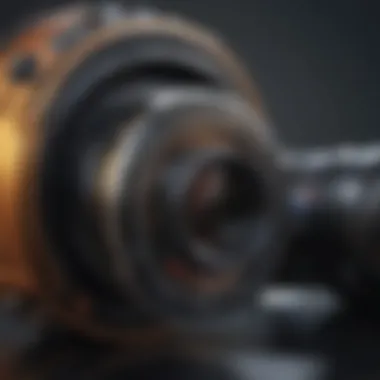
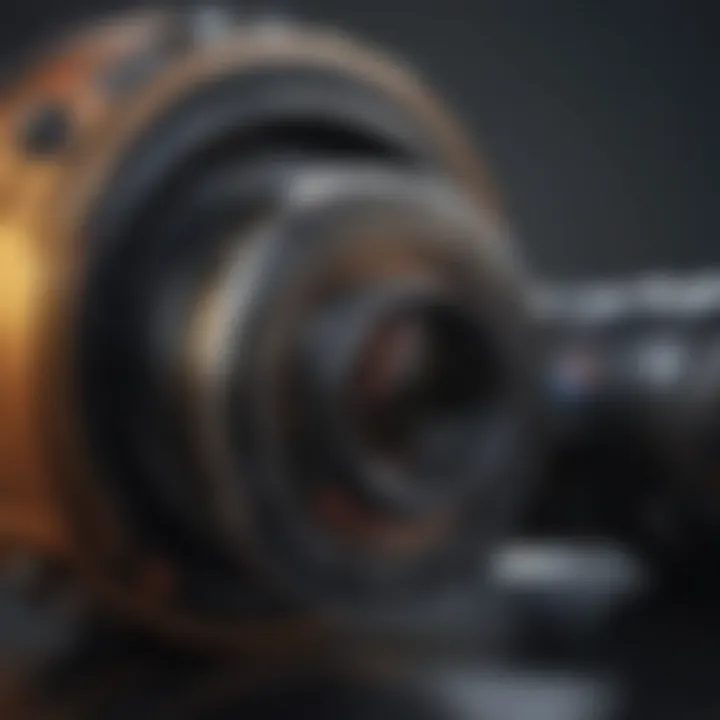
X-ray imaging is another fundamental modality that excels in visualizing dense and solid structures. Its primary application lies in medical research, allowing for internal visualization without invasive procedures. The key characteristic of X-ray imaging is its capacity to penetrate various materials and produce detailed images of dense tissues. However, the resolution might not match that of electron microscopy, limiting its use for finer analysis. Furthermore, X-ray exposure carries potential risks, requiring careful consideration in experimental design.
Capture Techniques
Camera Settings
Camera settings are integral to effective image capture. Proper calibration ensures images are not only clear but also appropriate for subsequent analysis. Key characteristics include adjusting exposure, aperture, and shutter speed to optimize image quality. This attention to detail is beneficial as it affects clarity and detail in the final images. However, overly complex settings may intimidate less experienced researchers, potentially leading to suboptimal capture conditions.
Lighting Conditions
Lighting conditions significantly influence the quality of acquired images. Proper illumination can enhance visibility of features, making them easier to analyze. The advantage of controlled lighting, such as using specific wavelengths or angles, can yield better images. On the downside, improper lighting can introduce glare or shadows that obscure important details, complicating analysis.
Sample Preparations
Sample preparations are crucial in ensuring that images accurately reflect the intended subjects of analysis. This involves various techniques, such as staining or mounting, to enhance contrast and preserve structures. Proper sample preparation allows researchers to maximize the information gleaned from images. It can be time-consuming and may introduce artifacts that mislead interpretations if not done carefully.
Proper image acquisition not only ensures clarity and detail but also sets the stage for successful and insightful analysis in scientific research.
Image Processing Techniques
Image processing techniques form a crucial component of image analysis in scientific research. They enhance image quality, making observations and measurements more accurate. Understanding these techniques is key for anyone involved in research where imaging plays a central role. Image processing allows researchers to extract meaningful information from raw data by applying systematic methods that can significantly improve analysis outcomes.
Preprocessing Methods
Preprocessing methods are essential in preparing images for further analysis. They focus on enhancing the quality of the input image, thus facilitating more accurate interpretations and decisions. Noise reduction and contrast enhancement are two primary preprocessing methods often employed.
Noise Reduction
Noise reduction refers to techniques aimed at minimizing unwanted random disturbances within an image. In scientific imaging, noise can obscure vital details, leading to incorrect conclusions. Therefore, noise reduction is seen as an essential first step in the image analysis process.
The key characteristic of noise reduction is its ability to improve clarity without significantly altering the original data. This is vital for preserving the integrity of scientific observations. Noise reduction can be implemented through various algorithms, such as Gaussian filters or median filters, which are widely used due to their popularity and effectiveness.
One unique feature of noise reduction techniques is their adaptability. They can be tailored for different imaging modalities, making them universally applicable in the context of scientific research. However, one disadvantage is that excessive noise reduction can blur critical details, potentially leading to information loss. Thus, a balance must be achieved to retain essential features while minimizing noise.
Contrast Enhancement
Contrast enhancement is another fundamental preprocessing technique that improves the visibility of features within an image. This method adjusts the brightness and contrast values to highlight specific areas of interest. Contrast enhancement significantly contributes to making subtle features more discernible, which is essential in many scientific examinations.
The primary advantage of contrast enhancement is its ability to draw attention to critical image features that might otherwise go unnoticed. Techniques such as histogram equalization or gamma correction are often used to achieve this outcome. These methods are popular because they provide a straightforward approach to improving the visual quality of images without complex interventions.
A unique aspect of contrast enhancement is that it can be tailored to specific requirements of different research fields. However, a potential downside is that it can lead to overemphasis on particular features, which might misrepresent the true nature of the data. Therefore, one must apply this technique judiciously to avoid misinterpretations.
Analysis Techniques
Following preprocessing, the next phase involves various analysis techniques to interpret the enhanced images. These techniques transform images into quantitative data that researchers can analyze and derive insights from. Key methods in this category include thresholding, edge detection, and segmentation.
Thresholding
Thresholding is an analytical technique that converts a grayscale image into a binary image by determining a specified intensity level. It plays a significant role in separating objects from the background, thus enhancing object visibility for further analysis.
The defining characteristic of thresholding is its simplicity and effectiveness. This method is beneficial because it allows clear distinction between objects and their backgrounds, making it easier for subsequent analysis stages. It is particularly popular in various applications, such as biomedical imaging, where distinguishing critical features from noise is paramount.
One unique feature of thresholding is its ability to be fine-tuned based on the specific images being analyzed. While it generally provides considerable benefits, excessive reliance on this method can lead to loss of crucial data, especially in images with subtle gradients or features. Managing these limitations is necessary for obtaining reliable results.
Edge Detection
Edge detection focuses on identifying points in an image where there is a significant change in intensity, highlighting the boundaries and contours of objects. This technique is vital for analyzing the shape and structure of various features in scientific images.
Edge detection stands out due to its critical role in object recognition and image analysis. It can greatly facilitate the understanding of complex images, making it a popular choice for researchers. By pinpointing edges accurately, one can simplify further investigations of the image’s content.
A unique aspect of edge detection techniques is their application to multiple scientific fields, including biology and materials science. However, one disadvantage can be sensitivity to noise, which may lead to the identification of false edges. Thus, it is essential to apply appropriate preprocessing methods before implementing edge detection to mitigate this issue.
Segmentation
Segmentation refers to the process of partitioning an image into multiple segments or regions. This is critical for isolating objects of interest within the image for more detailed analysis. Segmentation techniques employ algorithms that define and separate distinct features from one another.
The key characteristic of segmentation is its comprehensive capacity to reveal structures within an image. This method is particularly advantageous in biological studies, where distinguishing various cell types or components within tissue samples is often essential. It allows for more in-depth examination and understanding of the subjects being studied.
A unique feature of segmentation methods is their variety, which can cater to different image characteristics and research needs. However, determining the appropriate segmentation approach can be complex. If the segmentation is not conducted correctly, important nuances may be overlooked, leading to erroneous conclusions. Precision in this process is crucial for reliable outcomes.
Quantitative Image Analysis
Quantitative image analysis is essential in scientific research as it provides a systematic approach to extract meaningful data from images. This method allows researchers to convert visual information into quantifiable metrics, which can be further analyzed statistically. By measuring various image properties, scientists can derive insights that are critical for understanding biological phenomena, material characteristics, and geological transformations. Not only does quantitative image analysis enhance precision, but it also allows for reproducibility of results, which is a cornerstone of scientific inquiry.
Measurement of Image Properties
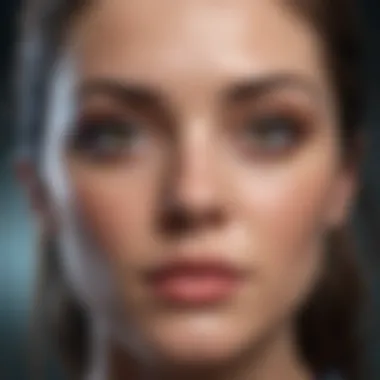
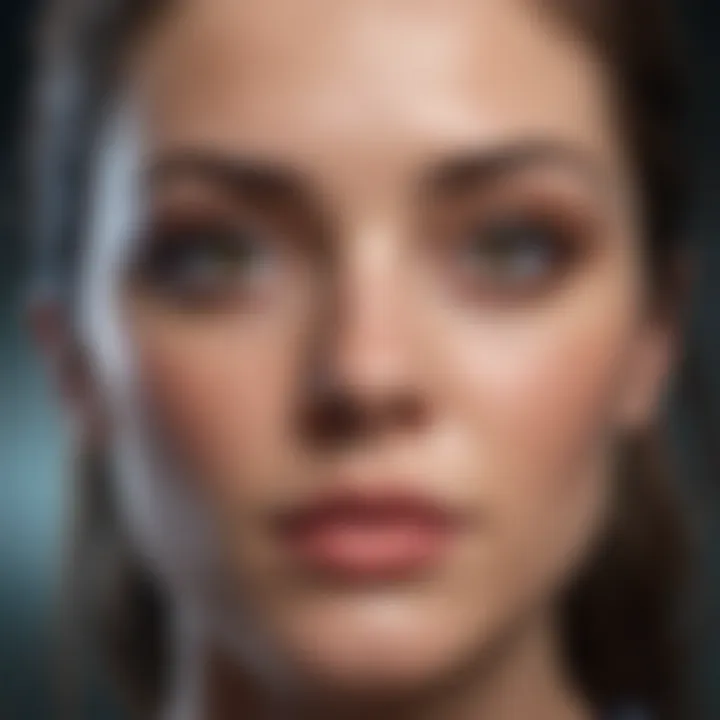
Area and Perimeter
The measurement of area and perimeter is a fundamental aspect of quantitative image analysis. By accurately quantifying these properties, researchers can assess the size and boundary of observed structures. For instance, in biological studies, measuring the area of cells can indicate growth rates and health status. This makes area and perimeter an accessible and beneficial choice for image analysis. One key characteristic of this approach is its straightforwardness. Researchers can use standard software to calculate these metrics, making it a popular choice for various applications.
An interesting feature of area and perimeter measurement is its adaptability. Different fields can apply these metrics in unique ways. In medicine, area calculations can help determine tumor sizes, while in materials science, perimeter measurements can reveal the shape of crystalline structures. However, care must be taken, as inaccuracies in edge detection can lead to misinterpretation of results, which is a potential disadvantage of this method.
Shape Analysis
Shape analysis is another significant aspect of quantitative image analysis that focuses on the geometric properties of structures. This method allows researchers to identify and classify shapes based on specific criteria. The ability to analyze shape makes it a powerful tool for applications like identifying cell types or assessing the morphology of materials. Its key characteristic is its effectiveness in discriminating between similar structures based on subtle shape differences.
Shape analysis becomes particularly relevant in fields such as biology and material science. For example, researchers can study the morphological alterations in cells when exposed to specific stimuli. A distinct feature of shape analysis is its reliance on advanced algorithms that can consider multiple parameters at once. Despite its advantages, it can be computationally intensive and may require sophisticated software, which could be a drawback for some laboratories.
Statistical Analysis in Image Data
Statistical analysis in image data is crucial for synthesizing and interpreting the results obtained from quantitative image analysis. This process allows researchers to identify trends and patterns, and it helps in making data-driven conclusions.
Data Normalization
Data normalization is a vital part of statistical analysis. This process adjusts the values measured across images to a common scale, facilitating comparison. It is crucial for ensuring that variations in conditions do not skew the results, making it a beneficial choice in diverse applications. Normalization helps in eliminating biases that can arise from differences in lighting, camera calibrations, or biological variability, which enhances the overall reliability of the data. However, while normalization improves accuracy, it can sometimes mask useful information if not applied judiciously.
Variable Correlation
Variable correlation examines the relationships between different measurable elements in image data. Understanding how variables relate can provide deeper insights into the system being analyzed. For example, in biological imaging, correlating cell size with proliferation rates might reveal important biological phenomena. This is a popular technique as it aids in hypothesis generation and testing. The unique feature of variable correlation is its ability to handle multivariate data, allowing researchers to observe interactions among multiple variables simultaneously. Nevertheless, it is essential to interpret correlations cautiously, as correlation does not imply causation, which can lead to misinterpretations of the data.
Applications Across Scientific Disciplines
The integration of image analysis within various scientific fields is essential for advancing research methodologies. Different disciplines harness image analysis to yield valuable insights, paving the way for innovation and deeper understanding. By employing specialized tools and techniques, researchers can extract meaningful data from visual representations, improving both the speed and accuracy of their findings. This section discusses applications in biology, chemistry, materials science, and earth sciences, illustrating the broad relevance of image analysis.
Biology and Medical Research
Cell Counting
Cell counting is a fundamental practice in biological and medical research. This technique enables researchers to quantify cell populations accurately. It is crucial for studies involving cellular responses, drug effects, and developmental changes. The key characteristic of cell counting is its ability to provide precise numeric data relevant for statistical analysis. This makes it a beneficial choice for this article.
Using automated systems like flow cytometers or image-based analyzers, researchers can process large sample sizes efficiently. However, limitations exist. Sample preparation and the complexity of visualizing certain cell types can hinder results. Yet, advances in technology continuously improve accuracy and reliability, making cell counting an invaluable tool in life sciences.
Histopathology
Histopathology refers to the microscopic examination of tissue samples. This technique is crucial for diagnosing diseases and understanding biological processes. The significance of histopathology lies in its ability to provide insights into disease progression and treatment responses. It is a popular area within this article due to its impact on patient care and clinical outcomes.
The unique feature of histopathology is its focus on cellular morphology and structure. Pathologists can distinguish between normal and abnormal tissues, aiding in early diagnosis. However, the interpretation of images can be subjective, leading to variability in results. Despite this, histopathological analyses play a critical role in modern medicine and research.
Chemistry and Materials Science
Microstructure Analysis
Microstructure analysis involves examining materials at the microscopic level to determine their properties. This technique is crucial for developing new materials and optimizing existing ones. The ability to observe grain size, phase distribution, and boundary characteristics makes microstructure analysis vital to this article's discussions.
Key characteristics include the use of techniques like Scanning Electron Microscopy and Atomic Force Microscopy. These methods allow for high-resolution imaging, revealing details that influence material behavior. However, challenges arise from sample preparation and environmental sensitivity, introducing variations in results. Despite these issues, microstructure analysis is indispensable in materials research.
Nano-materials Investigation
The investigation of nano-materials is an emerging field with vast implications for technology and medicine. This area focuses on materials with dimensions less than 100 nanometers, impacting their properties significantly. The importance of nano-materials investigation lies in its potential for innovation across various applications, making it a relevant topic in this article.
A key characteristic of this field is the ability to manipulate and analyze materials at the atomic level. Techniques such as Transmission Electron Microscopy play a crucial role in revealing structural insights. However, the complexity of nanoscale interactions can complicate data interpretation, presenting a challenge for researchers. Nevertheless, the advantages of exploring nano-materials are considerable, leading to advances in electronics, energy, and health.
Earth Sciences
Geological Mapping
Geological mapping is a process that involves detail observational work to create accurate representations of geological features. This technique is essential for resource exploration, environmental monitoring, and understanding geological hazards. Its importance in this article stems from its role in informing decisions related to land use and natural resource management.
The key characteristic of geological mapping is its reliance on high-resolution imagery combined with physical surveys. This method enables geologists to identify rock types, structures, and changes in the landscape effectively. However, variability in data quality can hinder comprehensive analyses. Despite this, geological mapping remains critical for tectonic studies and resource management.
Remote Sensing
Remote sensing uses satellite or aerial imagery to gather data about the Earth's surface and atmosphere. This technique is particularly relevant for environmental monitoring, agricultural assessments, and urban planning. The significance of remote sensing in this article lies in its ability to provide large-scale data efficiently and economically.
A key feature of remote sensing is its capacity for real-time data collection over expansive areas. This ability allows researchers to monitor changes and trends that are not easily observable through ground-based surveys. However, one challenge includes the interpretation of collected data, which necessitates expertise in both geography and data analysis. The benefits, though, are pronounced as remote sensing continues to revolutionize earth sciences and geography.
Emerging Technologies in Image Analysis
Emerging technologies are transforming the field of image analysis significantly. These advancements allow for greater precision, efficiency, and versatility in scientific research. With the ever-increasing volume of data generated by various imaging modalities, the ability to analyze images effectively is essential. The integration of new technological tools mitigates many traditional challenges faced in image analysis.
The benefits of adopting these technologies are numerous:
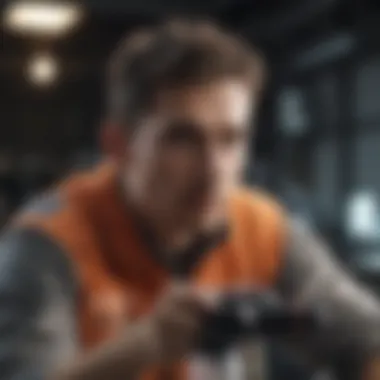
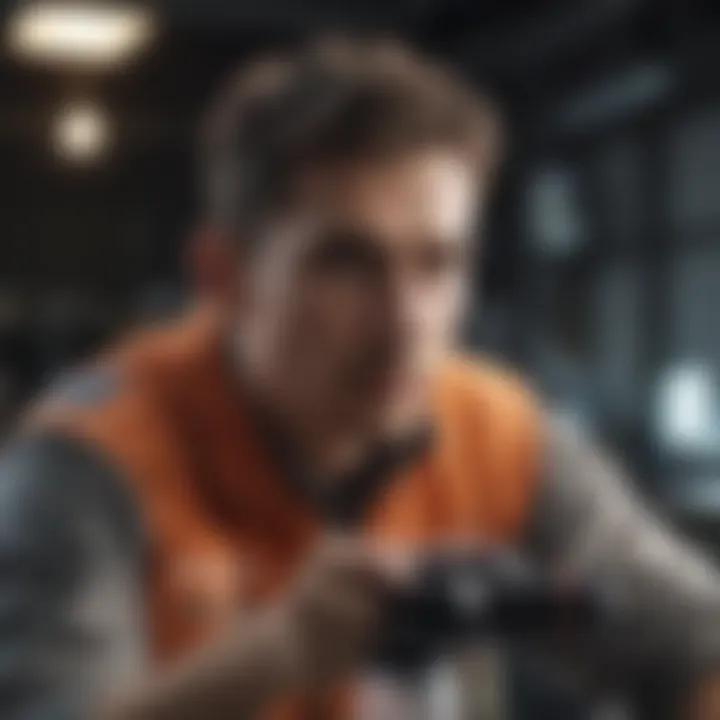
- Enhanced accuracy in image recognition and classification
- Reduced time required for data analysis
- Increased capacity to process large datasets
- Improved capabilities in predictive modeling and data interpretation
Considering these factors, it is critical for researchers and professionals to remain informed about developments in this domain.
Artificial Intelligence and Image Recognition
Artificial Intelligence (AI) is redefining the landscape of image analysis. Particularly, image recognition applications utilizing AI algorithms can automatically identify and classify objects within images. This capability has profound implications across various scientific disciplines.
For instance, in medical imaging, AI algorithms can assist radiologists in identifying tumors or anomalies with remarkable accuracy. These systems can analyze thousands of medical images rapidly, detecting patterns that may not be visible to the human eye. A key advantage of these AI-powered systems is their ability to learn from vast datasets, enhancing their performance over time.
Furthermore, AI applications in image analysis are benefiting fields such as biology and environmental science. Researchers can enhance biodiversity studies by utilizing AI for species identification in images collected from camera traps.
In addition, the implementation of AI solutions must consider the quality and diversity of training data to ensure that the models are robust and reliable.
Machine Learning Applications
Machine learning (ML), a subset of AI, plays a significant role in advancing image analysis techniques. ML algorithms are designed to learn from data and improve their predictive capabilities. In the context of image analysis, these methods can process large amounts of data and identify trends and correlations that may not be immediately apparent.
For example, in chemistry and materials science, ML can analyze the microstructure of materials captured through imaging. By training models on known data, researchers can predict the properties of new materials based on their microstructural characteristics. This application accelerates the discovery of new compounds and materials.
Additionally, machine learning enhances the interpretation of complex data sets. It can automate the tedious tasks associated with image classification, allowing researchers to focus on more significant inquiries. However, successful implementation of ML techniques often requires a strong foundation in statistical analysis to guide the development of algorithms and validation of results.
As image analysis becomes increasingly complex, the integration of AI and machine learning technologies provides a pathway to harnessing data in innovative and impactful ways.
Challenges in Image Analysis
Image analysis plays a critical role in scientific research, yet it is not without its challenges. This section addresses key obstacles that researchers encounter when utilizing image analysis techniques. Understanding these challenges is essential for improving the reliability and validity of research outcomes.
Data Quality and Variability
Data quality is paramount in image analysis. Poor quality data can distort analysis results, leading to incorrect conclusions. Factors that contribute to data quality include capturing methods, imaging modalities, and environmental conditions. Each of these factors can introduce noise, artifacts, or other imperfections in the finalized images, thus impacting the analysis. Variability between samples can also pose significant issues.
- Key aspects of data quality include:
- Consistency in data collection methods
- Calibration of imaging equipment
- Management of environmental controls when capturing images
Inconsistent data can lead to variations that affect measurement precision. Statistical methods can mitigate some variability; however, these methods require quality data to function optimally. Hence, understanding and improving data quality must be a priority in any image analysis endeavor.
Interpreting Complex Data Sets
Complex data sets arise frequently in scientific research, particularly in fields utilizing high-resolution imaging. The interpretation of these data sets presents its own challenges. Data sets may contain a multitude of variables which can be interrelated or even confounding. This complexity makes it difficult to establish clear relationships or patterns, hindering effective analyses.
Researchers often face the dilemma of how to present and convey findings drawn from complex data. Insightful visualization techniques can aid in interpretation, yet choosing the right method requires significant expertise. Common strategies include:
- Utilizing appropriate software tools: Software such as ImageJ or MATLAB can be employed for advanced data manipulation.
- Employing machine learning algorithms: These can help in predicting outcomes based on complex variables.
- Developing robust statistical models: Such approaches can assist in elucidating relationships within the data.
Developing an understanding of these complexities is not just important; it’s fundamental in ensuring that research findings are valid and actionable.
The Future of Image Analysis in Research
The landscape of image analysis in scientific research is rapidly evolving. As technology advances, the methods used for image analysis are becoming more sophisticated, allowing researchers to extract detailed insights from images in various fields. Understanding the future of this discipline is critical for scientists who wish to stay at the forefront of their research.
Emerging technologies such as artificial intelligence and machine learning are playing a pivotal role in shaping the future of image analysis. These technologies streamline the analytical processes, increasing accuracy and efficiency. They can analyze vast amounts of image data quickly, uncovering patterns and trends that would be laborious or impossible for human analysis alone. Moreover, as the volume of image data generated in research grows, the importance of automated systems becomes even more pronounced. Researchers must adapt to these new tools and methodologies to maintain relevance in their respective fields.
Trends to Watch
Several trends are leading the way for the future of image analysis in research:
- Integration of AI: The incorporation of artificial intelligence will likely lead to more intuitive image analysis tools. These tools will not only assist in analyzing raw data but also suggest hypotheses based on observed patterns.
- Collaboration Across Disciplines: Image analysis will increasingly become a collaborative effort between disciplines. Biologists may work with computer scientists to develop new imaging techniques, enhancing both fields.
- Real-time Analysis: As processing power increases, real-time image analysis will become commonplace in research settings. This will allow for immediate feedback and adjustments during experiments, leading to faster iterations.
Predicted Innovations
Looking ahead, several innovations are anticipated to further revolutionize image analysis:
- Advanced Imaging Techniques: Techniques such as super-resolution microscopy will provide unprecedented detail in images, allowing researchers to observe phenomena at the molecular level with increased clarity.
- Customized Software Solutions: Tailored software that adapts to specific research needs will emerge, providing more relevant analytical capabilities for diverse scientific domains.
- Enhanced Data Visualization: As data becomes more complex, innovative visualization tools will be necessary. These tools will help researchers interpret their findings more effectively, allowing for better communication within the scientific community and with the public.
"The advancement of image analysis tools will not only enhance research outcomes but also redefine our understanding of complex biological systems."
In summary, the future of image analysis in research signifies a shift towards more integrated, automated, and sophisticated methods. Staying abreast of these trends and innovations is essential for researchers aiming to leverage the full potential of image analysis in their work.
End
In any scholarly work, the conclusion serves as a pivotal element. It encapsulates the essence of the discussion and reiterates the significance of the topic analyzed. In this article, the role of image analysis in scientific research has been explored in depth, demonstrating its transformative potential across disciplines.
Through this comprehensive approach, we highlight how scientific advancements hinge on effective image analysis. From biology to earth sciences, image processing is not merely a supportive tool but a fundamental component of research methodologies. It ensures that data is not only captured but also accurately interpreted.
The implications of these discussions are profound. Researchers and students must recognize how image analysis can refine their investigative processes, allowing for deeper insights and more robust conclusions. The techniques explored in the article, such as statistical analysis and measurement of image properties, offer tangible methods to enhance the validity of findings. This in-depth understanding equips professionals to tackle the complexities of image data with confidence.
Moreover, the challenges identified in image analysis, such as data variability and complexity, demand ongoing attention. Recognizing these pitfalls is crucial for developing more resilient analytical strategies and ensuring high-quality research outputs. The integration of emerging technologies and methodologies presents a promising future for image analysis, making it essential for researchers to stay abreast of these developments.
In summary, the conclusion emphasizes that image analysis is an indispensable facet of modern scientific inquiry. It enhances communication of results, supports innovative approaches, and bridges various scientific fields. Adopting a proactive stance toward image analysis will empower researchers to elevate their work, fostering a richer and more informed scientific community.