Understanding Decision Support Systems in Healthcare

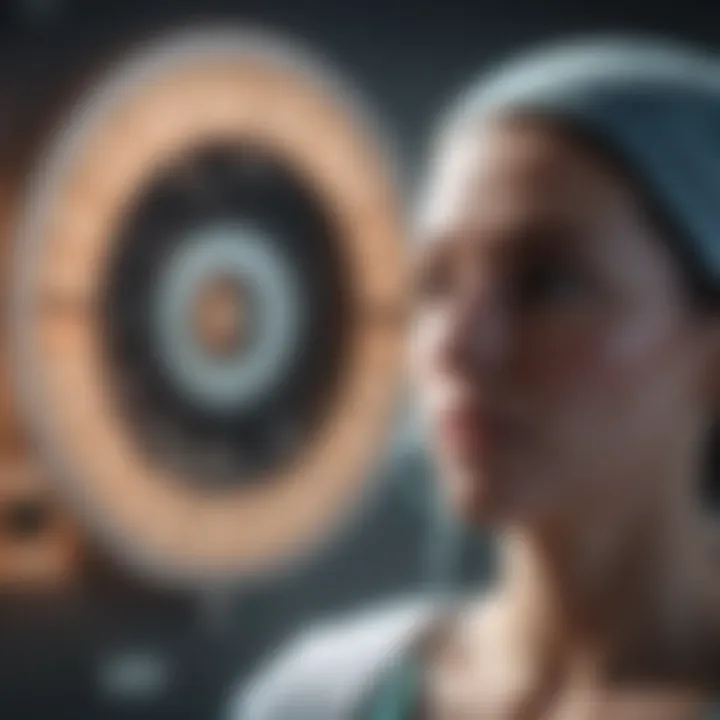
Intro
Decision support systems (DSS) have gradually become a crucial part of healthcare. Their purpose is to enhance decision-making in clinical settings. As healthcare grows more complex, DSS helps professionals make informed choices based on various data inputs. These systems integrate clinical, administrative, and patient data, which provide actionable insights for healthcare providers.
Understanding the components and significance of DSS is essential for both practitioners and stakeholders involved in healthcare management. This understanding can lead to improvements in patient care, administrative efficiency, and overall healthcare outcomes.
The following sections will explore how DSS functions, the challenges it faces, its impact on decision-making, and the future of these systems in healthcare delivery.
Methodology
Study Design
A comprehensive analysis of decision support systems in healthcare requires a detailed study design that includes qualitative and quantitative research. This approach supports a broader understanding of how DSS is implemented and its results on patient care. Surveys can be sent to healthcare professionals about their experiences with DSS. Additionally, statistical data may be collected from hospitals that have integrated these systems.
Data Collection Techniques
Data collection for understanding DSS can utilize several techniques, including:
- Surveys: Collecting feedback from healthcare providers who use DSS.
- Interviews: Conducting structured interviews with information technology experts and clinicians.
- Case Studies: Reviewing specific implementations of DSS in various healthcare settings.
- Literature Review: Analyzing existing research on DSS efficacy and its influence on clinical outcomes.
These diverse strategies provide a well-rounded view of DSS and its role in healthcare. They also help identify common strengths and weaknesses across various implementations.
Discussion
Interpretation of Results
The results gathered from these methodologies can reveal critical insights into how DSS influences clinical decision-making. Many studies show that DSS leads to improved diagnostic accuracy and safer prescribing practices. The use of these systems can streamline processes, allowing healthcare professionals to focus on patient interactions rather than administrative duties.
Limitations of the Study
However, there are limitations to consider. For instance, some healthcare facilities may not fully utilize DSS due to lack of training or resources. Furthermore, reliance on technology can sometimes overshadow clinical judgment. Variability in data input quality can also lead to inconsistent outcomes.
Future Research Directions
Future research should focus on overcoming current limitations. Developing more user-friendly interfaces could enhance user experience. Additionally, investigating the long-term effects of DSS on healthcare outcomes would provide greater clarity. Exploring the role of artificial intelligence in DSS development can also lead to innovations that further improve patient care.
Prologue to Decision Support Systems
The significance of Decision Support Systems (DSS) in healthcare cannot be overstated. They act as integral components that enhance the decision-making processes of healthcare professionals. DSS provide crucial information and data analysis, allowing clinicians to make informed choices regarding patient care. This is particularly important in an environment where rapid, accurate decisions can directly influence patient outcomes.
Decision Support Systems utilize data from multiple sources, including patient records, clinical guidelines, and research papers. This integration of information supports healthcare providers in diagnosing illnesses, selecting appropriate treatments, and anticipating potential complications. As such, DSS can reduce errors and improve overall healthcare delivery.
Moreover, DSS also play a role in optimizing resource allocation and operational efficiency within healthcare organizations. By streamlining processes and workflows, healthcare facilities can better manage costs while maintaining high standards of care.
In summary, the introduction of DSS in healthcare signifies a shift toward more data-driven decision-making processes. Their importance lies not only in improving clinical outcomes but also in advancing efficiencies across healthcare systems.
Definition of Decision Support Systems
Decision Support Systems in healthcare are software tools designed to assist in making informed clinical decisions. They analyze vast amounts of medical data to provide recommendations, alerts, and insights tailored for healthcare professionals. By employing algorithms, these systems can help identify potential diagnoses or suggest treatment options based on patient-specific information. DSS can be classified into three main types: clinical, operational, and strategic systems, each serving distinct purposes in the healthcare environment.
Historical Development of DSS
The evolution of Decision Support Systems can be traced back to the 1970s. Initially, DSS were rudimentary and focused mainly on data management. As technology progressed, these systems began to incorporate more complex algorithms. The late 1980s marked a significant turning point, as the integration of artificial intelligence technologies allowed for more intelligent systems that could mimic human decision-making processes. Over the years, advancements in computing power and data analytics further propelled the capabilities of DSS, leading to their current form that significantly enhances clinical decision-making in todayβs healthcare landscape.
Importance of DSS in Healthcare
The relevance of Decision Support Systems (DSS) in healthcare cannot be overstated. Such systems enhance the decision-making processes that professionals engage in daily. They use data and intelligent algorithms to assist in making informed choices regarding patient care. The benefits of DSS span multiple dimensions, impacting not only clinical outcomes but also operational efficiency.
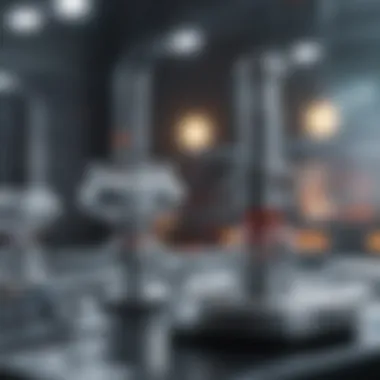
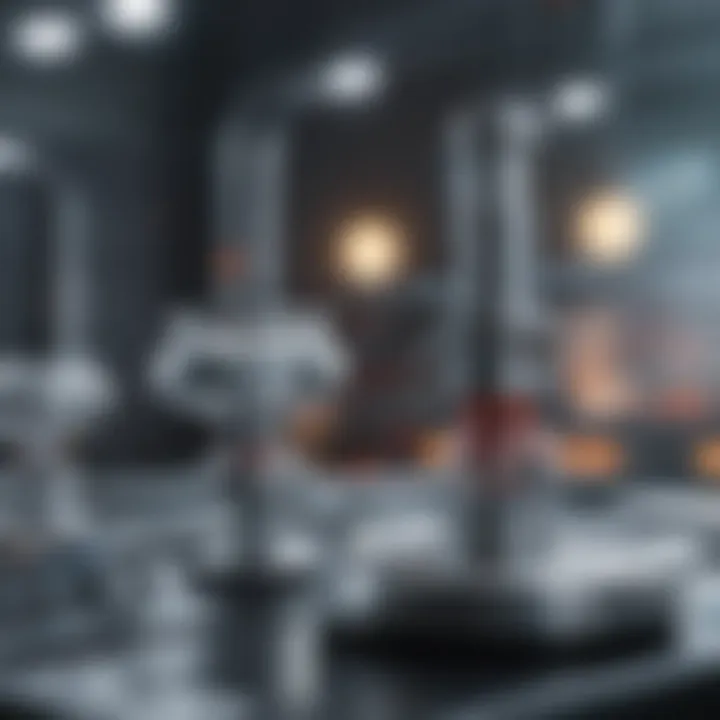
Enhancing Clinical Decision-making
Clinical decision-making is complex and requires careful analysis of vast amounts of data. Decision Support Systems offer tools that help healthcare professionals synthesize information quickly and accurately. These systems often integrate evidence-based guidelines, which support clinicians in diagnosing conditions and selecting optimal treatment pathways.
For instance, Clinical Decision Support Systems (CDSS) leverage patient data along with clinical guidelines to suggest specific interventions personalized to individual cases. The precision in recommendations can minimize mistakes associated with human error, thus strengthening patient safety and care outcomes.
Improving Patient Care Quality
Quality of patient care is a critical focus in healthcare. Decision Support Systems play a pivotal role in maintaining high standards by ensuring treatment adherence to best practices. By providing real-time data and insights, DSS fosters continuous monitoring of patient health, which allows for timely interventions. This proactive approach reduces complications and improves the overall effectiveness of care.
Moreover, DSS can enhance patient engagement. Systems that include features for sharing information with patients encourage more active participation in their healthcare journey. Educated patients are more likely to adhere to treatment plans, leading to better health outcomes.
Streamlining Healthcare Operations
Operational efficiency is crucial for the sustainability of healthcare systems. Decision Support Systems streamline processes such as scheduling, resource allocation, and patient flow management. Automating these aspects frees healthcare providers to focus more on delivering care rather than administrative tasks.
The use of DSS can also reduce redundancy. For instance, through data sharing, healthcare professionals can avoid unnecessary repeat tests or interventions. This not only saves time but also reduces costs associated with patient care. In a landscape where resources can be limited, every bit of efficiency contributes to the overall effectiveness of healthcare delivery.
"DSS in healthcare not only supports clinicians in making better decisions but also enhances the overall quality and efficiency of healthcare services."
In summary, the importance of Decision Support Systems in healthcare lies in their ability to improve clinical decision-making, elevate patient care quality, and streamline operations. As these systems continue to evolve, their integration into everyday practices will undoubtedly continue to reshape the healthcare landscape.
Components of Decision Support Systems
The construction of effective decision support systems (DSS) in healthcare relies heavily on specific components that work together to ensure optimal functionality. Understanding these components is key for stakeholders, as they enhance both the reliability and the effectiveness of DSS. The integration of these crucial building blocks not only advances clinical decision-making but also amplifies patient care quality across the healthcare landscape. The main components include data management, knowledge base systems, and user interface design.
Data Management Components
Data management serves as the backbone of any decision support system. It involves the collection, storage, processing, and dissemination of data in a manner that supports analysis and informed decision-making. Key elements of data management in DSS include:
- Data Collection: This refers to how data is gathered from various sources such as EHRs (Electronic Health Records), laboratories, and digital imaging. Accurate collection is crucial to ensure that the system works effectively and provides valid recommendations.
- Data Storage: Proper storage methods must be in place to maintain the integrity of the data. The use of secure databases can minimize risks related to data loss or corruption.
- Data Processing: This involves transforming raw data into useful information through various algorithms and analytical methods. The efficiency of this process greatly affects the speed and accuracy of the system's outputs.
- Data Governance: Ensuring compliance with regulations is mandatory. Organizations must manage data responsibly while protecting patient privacy and security.
Each of these aspects contributes towards enhancing the overall performance of DSS, making data management an indispensable component.
Knowledge Base Systems
Knowledge base systems are at the heart of a decision support system. They consist of a vast repository of information that informs clinical guidelines, protocols, and expertise. The importance of these systems cannot be overstated:
- Clinical Guidelines: A well-designed knowledge base includes up-to-date clinical guidelines which are essential for accurate decision support.
- Best Practices: Knowledge bases provide access to evidence-based practices that can lead to standardization in care delivery.
- Integration of Previous Cases: Historical medical cases can offer valuable insights into patient outcomes, enabling better forecasting and recommendations.
Furthermore, utilizing a robust knowledge base allows healthcare professionals to make decisions backed by data and expert advice, leading to improved patient outcomes.
User Interface Design
User interface design plays a crucial role in the health care effectiveness of DSS. A well-designed interface makes it easy for clinicians to interact with the system without hindrance. Key considerations include:
- Usability: Interfaces should be user-friendly, allowing for quick navigation. This reduces cognitive load on healthcare professionals, enabling them to focus on patient care.
- Customization: Personalization features allow users to tailor the interface to meet their specific needs, streamlining workflows.
- Accessibility: Ensuring the system is accessible across various devices enhances usability for clinicians who may work in different environments.
Designing an effective user interface not only improves user satisfaction but also encourages broader adoption of DSS in clinical settings.
"A robust user interface design elevates the utility of decision support systems, directly impacting the quality of care delivered."
In sum, the components of decision support systems should not be viewed in isolation. Collectively, they create a framework that empowers healthcare providers to make informed decisions, ultimately leading to better patient care and efficient healthcare operations.
Types of Healthcare Decision Support Systems
Decision Support Systems play various crucial roles in healthcare. Understanding the different types of systems is key to grasping their applications and potential benefits. Each type serves distinct purposes, tailored to specific needs within the healthcare landscape.
Clinical Decision Support Systems (CDSS)
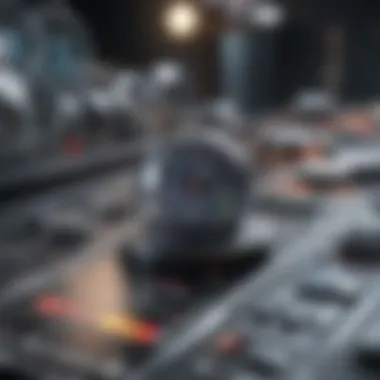
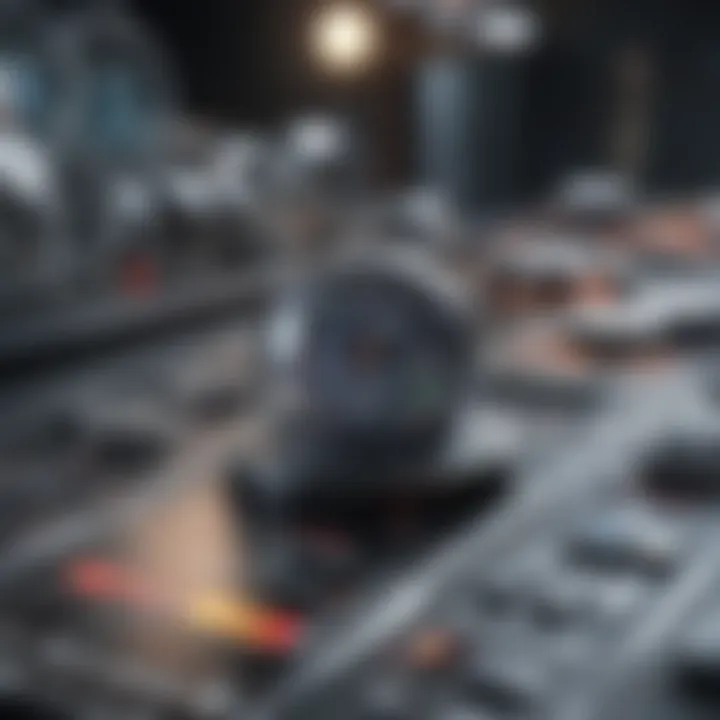
Clinical Decision Support Systems are designed to assist healthcare professionals in making informed clinical decisions. These systems analyze patient data and provide insights that can lead to better diagnosis and treatment options. For example, a CDSS might alert a doctor about potential drug interactions based on a patient's medication list. This can significantly improve patient safety and outcomes by reducing the chances of medical errors.
Furthermore, CDSS can be integrated with Electronic Health Records (EHR) systems, creating a seamless flow of information. This integration allows for real-time access to patient data and clinical guidelines, streamlining the decision-making process.
"The effective use of CDSS can enhance clinical performance and increase the quality of care provided to patients."
Operational Decision Support Systems
Operational Decision Support Systems focus on the daily management of healthcare operations. These systems are essential for optimizing resource allocation, scheduling, and workflow management. By providing analysis and suggestions for operational efficiencies, they help healthcare providers to manage their facilities effectively. For instance, scheduling systems can predict patient volumes and adjust staff requirements accordingly, ensuring that resources are used efficiently.
These types of systems also play a role in supply chain management within healthcare organizations. They provide visibility into inventory levels and enable proactive ordering of supplies, preventing shortages that could disrupt service delivery. As a result, Operational Decision Support Systems can lead to cost savings and increased productivity.
Strategic Decision Support Systems
Strategic Decision Support Systems are more focused on the long-term objectives of healthcare organizations. They assist leaders in making high-level decisions regarding policy, strategic initiatives, and market positioning. By analyzing large datasets, these systems can identify trends that inform future planning and investment.
For example, a hospital might use a Strategic Decision Support System to assess population health trends in their service area. This information can guide decisions on expanding services or resources, ultimately impacting the community's health positively.
In summary, understanding the various types of Healthcare Decision Support Systems is essential for recognizing their benefits. Each type contributes uniquely, from enhancing clinical outcomes to optimizing operations and shaping strategic direction. By leveraging these systems effectively, healthcare organizations can improve overall efficiency and patient care.
Technological Advances in DSS
The realm of decision support systems (DSS) in healthcare is rapidly evolving. The incorporation of technological advances significantly enhances their effectiveness. As healthcare becomes increasingly data-driven, the role of technology becomes critical. Therefore, a detailed analysis of these advances provides essential insights into their ongoing impact on healthcare delivery and decision-making processes.
Integration of Artificial Intelligence
Artificial Intelligence (AI) is at the forefront of technological advancements in DSS. Its integration reshapes the ways healthcare providers approach patient management and treatment plans. AI algorithms can analyze large datasets much faster than human capabilities. This leads to more accurate predictions and improves the quality of decision-making based on historical data.
One notable application of AI in DSS is in diagnostic support systems. These systems can assist physicians in identifying diseases much earlier than traditional methods. By using machine learning, they constantly improve their accuracy as they process more data. AI integration also allows for personalized medicine approaches, where treatments can be tailored to individual patient profiles, enhancing outcomes significantly.
Big Data Analytics in Decision Making
Big data analytics plays a crucial role in handling the vast amounts of information generated in healthcare. DSS can leverage this data to provide actionable insights to healthcare professionals. With advanced analytics, it becomes possible to discover patterns and trends that inform clinical decisions.
For instance, predictive analytics can identify patient risks based on historical health data. This helps in the proactive management of diseases. Additionally, real-time data processing can enhance operational efficiencies, ensuring that healthcare facilities can respond quickly to patient needs. This responsiveness ultimately leads to improved patient care and resource allocation.
Cloud Computing and DSS Deployment
Cloud computing offers immense advantages for the deployment of DSS. It provides scalable resources and enhances accessibility, allowing healthcare professionals to access decision support tools from anywhere. This flexibility is critical in modern healthcare, where timely information is key to effective patient care.
Furthermore, cloud solutions enable seamless data sharing among stakeholders. This interconnectedness fosters collaboration across various healthcare settings, enhancing the overall decision-making process. The deployment of DSS through the cloud also simplifies updates and maintenance, ensuring that systems utilize the latest technology and data.
Challenges in Implementing DSS
Implementing Decision Support Systems (DSS) in healthcare settings presents several challenges. These obstacles must be addressed to ensure successful integration and optimal functionality. Each challenge demands careful consideration by stakeholders, as it can significantly affect both the adoption process and the overall efficacy of DSS solutions.
Data Privacy and Security Concerns
Data privacy and security are paramount when deploying DSS in healthcare. The sensitivity of patient information requires stringent measures to protect against breaches. Regulatory frameworks like HIPAA in the United States set strict guidelines for managing health data. Any data leak or unauthorized access could result in severe penalties and undermine patient trust.
Many healthcare providers are hesitant to adopt DSS due to fears surrounding data misuse. Concerns about third-party vendors accessing sensitive information can further complicate implementation efforts. Therefore, organizations must prioritize robust security protocols and educate staff about data management practices. This combination can mitigate risks and reinforce trust.
"The challenge of data security in healthcare is not just technical; it is fundamentally about trust and integrity of patient care."
Resistance to Change in Clinical Practice
Another significant barrier in DSS implementation is the resistance to change among healthcare professionals. Many clinicians have established routines and may be skeptical of new technologies. They might believe that DSS undermines their expertise or complicates their decision-making process. This can lead to a lack of adoption or improper use of the systems.
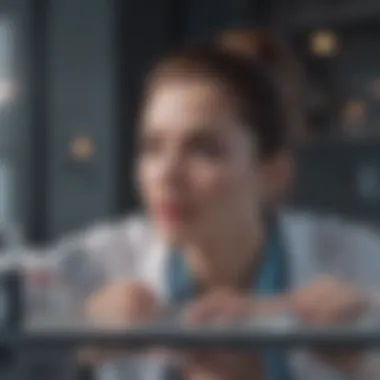
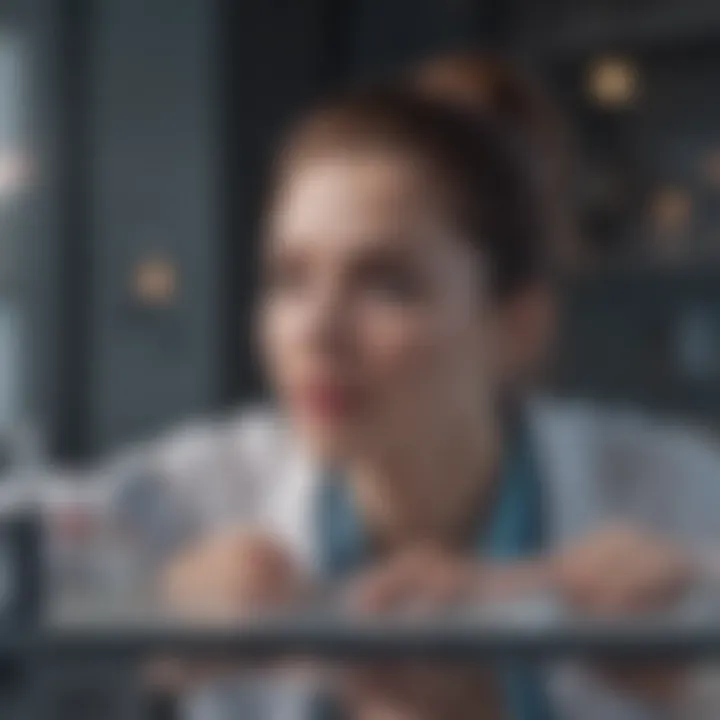
To overcome this resistance, healthcare leaders should engage clinicians early in the process. Providing training and demonstrating how DSS can enhance their work, rather than hinder it, is crucial. Involving teams in discussions about technology can create a sense of ownership and encourage a more receptive attitude towards new systems.
Cost of Implementation and Maintenance
Cost considerations play a critical role in the effectiveness of DSS in healthcare. The financial burden of acquiring, implementing, and maintaining these systems can be significant. Many organizations need to allocate resources not only for the software and hardware but also for training staff and ongoing support.
Finding balance between quality and budget is essential. Some organizations may opt for basic systems that meet immediate needs while delaying full integration of advanced features. This can be short-sighted if it results in further problems down the line.
Organizations must constantly assess the return on investment from DSS solutions. Making a case for long-term benefits can persuade stakeholders to commit resources to these systems, ensuring better patient outcomes and operational efficiency.
Evaluating Effectiveness of DSS in Healthcare
Evaluating the effectiveness of Decision Support Systems (DSS) in healthcare is crucial for understanding their impact on clinical outcomes, operational efficiency, and overall patient care. This evaluation helps stakeholders identify strengths and weaknesses in existing systems, guiding enhancements and future implementations. Considering the rate at which technology evolves, ongoing assessment is necessary to ensure that DSS remains aligned with the ever-changing healthcare landscape.
Metrics for Assessment
To assess the effectiveness of DSS, various metrics can be employed. Significant focus is typically placed on quantitative measures, but qualitative insights also hold importance. Here are some key metrics often used:
- Clinical Outcomes: Measures such as mortality rates, readmission rates, and treatment success rates can indicate how DSS influences patient care.
- User Satisfaction: Surveys and feedback from healthcare professionals using the systems can gauge their satisfaction and perceived utility of the DSS.
- Efficiency Gains: Analysis of time saved in decision-making processes and reduced redundancy can reflect operational improvements.
- Cost-effectiveness: Comparing costs associated with system implementation and maintenance against savings in treatment and administration can provide insights into financial viability.
- Compliance Rates: Monitoring how well clinicians adhere to guidelines suggested by DSS can reveal the system's impact on clinical practice.
Incorporating these metrics into a cohesive evaluation strategy can yield a comprehensive understanding of how effective a DSS is in improving various aspects of healthcare.
Case Studies of Successful Implementations
Real-world applications of DSS in healthcare provide valuable insights into their effectiveness. Examining successful implementations can highlight best practices, challenges overcome, and the tangible benefits of these systems. Here are notable examples:
- Epic Systems: A prominent healthcare provider, Epic has integrated clinical decision support features into its Electronic Health Records (EHR) platform. The results showed improved adherence to clinical guidelines and a reduction in medication errors, demonstrating the positive effects of DSS on patient safety.
- IBM Watson Health: Watson Health has collaborated with healthcare institutions for oncology decision support. By analyzing vast datasets, it assists oncologists in treatment planning. Studies reported that Watson's recommendations often matched expert opinion, showing potential for improved treatment decisions.
- Cerner Corporation: Cernerβs Health Information Exchange allows seamless sharing of patient data across different providers. As a result, clinicians reported a faster retrieval of patient history and better-informed decisions. This case exemplifies how DSS can enhance collaborative care.
"DSS can transform the way healthcare providers analyze information, leading to more informed decisions and better patient outcomes."
By documenting such case studies, stakeholders can learn from successful applications and adapt their strategies accordingly, ultimately enhancing the effectiveness of DSS implementation in healthcare.
Future Outlook for Decision Support Systems
The future of decision support systems (DSS) in healthcare is critical to understand, as it is directly tied to potential improvements in patient outcomes and operational efficiency. With the rapid advancements in technology and the growing complexity of healthcare needs, the evolution of DSS is both a challenge and a significant opportunity. This section examines both emerging trends in technology and potential areas for research that will shape the landscape of DSS.
Emerging Trends in Healthcare Technology
The integration of technology into healthcare continues to advance, with several key trends standing out:
- Artificial Intelligence and Machine Learning: AI is expected to play a larger role in DSS. These technologies can analyze vast data sets, improving the accuracy of predictions and recommendations for clinical decisions. AI can help identify patient patterns that may not be immediately visible to human analysts.
- Telemedicine Integration: As telemedicine gains popularity, DSS must adapt to support remote healthcare providers. These systems can provide real-time data and support to clinicians in various settings, ensuring that patients receive consistent care regardless of location.
- Interoperability: There is a rising need for systems to communicate with each other effectively. Future DSS must focus on integrating with Electronic Health Records (EHR) and other data sources to ensure comprehensive patient information is available for decision-making.
- Enhanced User Interfaces: The design of user interfaces will play a critical role in DSS adoption. Future systems must be intuitive, allowing healthcare providers to navigate complex data effortlessly.
This evolution in technology not only enhances existing systems but also presents new opportunities to develop innovative solutions tailored to specific healthcare challenges.
Potential Research Directions
As the field of decision support systems in healthcare progresses, various research directions are developing:
- Evaluating AI-driven DSS: More studies are needed to assess how AI impacts clinical outcomes. Researchers can explore how such systems influence the quality of care and the efficiency of healthcare providers.
- User Experience Studies: Research focusing on the user interface and experience can provide insights into how clinicians interact with DSS. This can help design systems that align closely with the workflows of healthcare professionals.
- Data Privacy Research: With the growing use of big data and analytics, understanding how to maintain privacy and comply with regulations is paramount. Research can investigate best practices for securing sensitive health data within DSS.
- Impact of System Interoperability: Studying how improved interoperability between systems can enhance healthcare delivery is critical. Research can focus on the benefits of seamless data sharing across platforms.
Ending
The conclusion serves as an essential component of this discussion on Decision Support Systems (DSS) in healthcare. It encapsulates the insights and findings presented throughout the article, consolidating the value of DSS technologies in modern medical practices.
One pivotal aspect to emphasize here is the transformative role DSS is playing in the healthcare landscape. These systems are no longer just an adjunct; they are a critical part of how clinical decisions are made, impacting patient outcomes and enhancing healthcare delivery processes. By integrating various data sources, including clinical records and research findings, DSS allows healthcare professionals to make more informed, evidence-based decisions.
The benefits of DSS are numerous. Clinicians can rely on these systems to reduce diagnostic errors, streamline treatment protocols, and improve overall patient safety. Furthermore, DSS contributes to operational efficiency within healthcare facilities, helping staff manage resources better and allocate time effectively.
However, it is also important to consider the complexities involved in the implementation of these systems. Issues like data privacy, user acceptance, and the costs of investment come into play. Therefore, stakeholders must weigh the advantages against these challenges when considering DSS integration.
In summary, DSS are an evolving element of the healthcare environment. As technology continues to advance, these systems will likely become even more integrated into everyday practice, providing robust support for clinicians in delivering high-quality care.
"DSS is not just about technology; it is about transforming the way we think about decisions in healthcare."
This perspective highlights the need for ongoing evaluation and adaptation of DSS as the healthcare landscape evolves. Through continuous improvement and innovation, DSS can align with both clinician needs and patient expectations, thereby further enhancing their value in care settings.