Artificial Intelligence Forecast: Future Insights Revealed
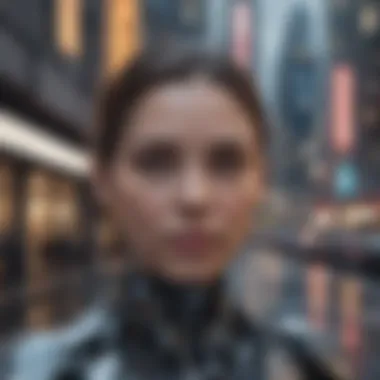
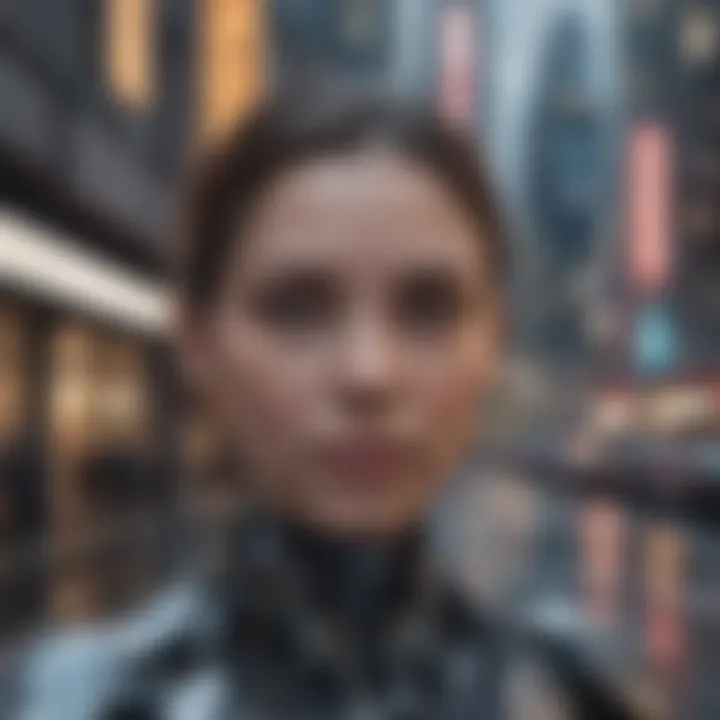
Intro
Artificial Intelligence (AI) is rapidly evolving. This article investigates the current landscape and future predictions in the realm of AI. Crucial advancements take place across a multitude of sectors, each influencing the technology's trajectory. The analysis will cover not only significant developments but also address the accompanying ethical and economic questions.
Understanding AI forecasts is vital for students, researchers, and professionals alike. This exploration frames the context of AI advancements and how they integrate with ongoing scientific inquiry. Moreover, as AI continues to influence various disciplines, its implications must be scrutinized carefully to navigate potential challenges ahead.
Methodology
Study Design
The exploration of AI forecasts in this article follows a systematic methodology. It begins by defining core aspects of AI, outlining its technological evolution. The analysis focuses on multiple domains, including healthcare, finance, and autonomous systems. Each domain provides a concrete example of how AI is currently utilized and what can be expected in the future.
Data Collection Techniques
To inform this study, diverse data sources were employed:
- Literature Review: Academic journals and reputable publications provide a theoretical foundation for highlighting significant trends in AI development. Sources like Wikipedia and Britannica offer curated insights into the history and evolution of AI.
- Surveys and Reports: Industry reports, such as those from McKinsey and Gartner, supply real-world applications and case studies of AI's impact.
- Expert Interviews: Conversations with AI professionals contribute practical perspectives on challenges, opportunities, and ethical considerations.
Discussion
Interpretation of Results
The gathered data reveals several key themes. Some advancements showcase AI's ability to enhance efficiencies across sectors. For example, in healthcare, AI algorithms hold promise for improving diagnostic accuracy. However, these developments also raise questions about data privacy and equity.
Limitations of the Study
Despite the comprehensive approach, the study faces notable limitations. The rapidly changing nature of AI technology means some information may become outdated quickly. Additionally, the reliance on selected sources may not encompass all perspectives, especially from emergent technology hubs worldwide.
Future Research Directions
Future investigations should encompass a broader geographical scope to include varied perspectives on AI development. Further analysis could also explore specific case studies that highlight the intersection of AI with other scientific fields. As AI evolves, continuous inquiry into ethical implications, regulatory frameworks, and societal impacts will be crucial in shaping its future trajectory.
"The unfolding narrative of artificial intelligence is not purely technological; it is inherently social and ethical in its implications."
Prologue to AI Forecasting
Artificial Intelligence (AI) forecasting is an essential topic within the broader scope of technological advancements. It involves predicting how AI will evolve, influence various sectors, and reshape daily life in the coming years. Understanding this concept is crucial as it allows individuals and organizations to prepare for disruptive changes, harness opportunities, and mitigate potential risks.
Among the various elements surrounding AI forecasting, one of the most significant is the role of predictive analytics. This method utilizes current and past data to anticipate future trends. Accurate predictions can lead to informed decision-making and strategic planning across sectors, from business to healthcare. With AI's growing footprint, possessing foresight can equate to a competitive advantage in rapidly changing markets.
It is also important to recognize AI forecasting's implications thoughtfully. These predictions form the groundwork upon which policies and investments are made, meaning that inaccuracies can lead to misguided strategies. Stakeholders must critically assess and validate such forecasts, balancing ambition with realism for effective implementation.
Understanding AI involves more than technical specifications. It encapsulates the socio-economic impacts resulting from technological integrations. If businesses adopt a limited view of AI, they might miss broader implications that transcend organizational boundaries. Forecasting helps expand this view by connecting dots across industries and disciplines, unveiling the deeper narrative of transformation underway.
As we delve deeper into AI forecasting, it becomes increasingly evident that our perception of technology is not simply academic. It's about shaping a resilient future that acknowledges both possibilities and limitations. This discourse serves as a foundation for discussions on the advancements and pressing challenges we face in this ever-evolving landscape.
Understanding AI and Its Scope
Artificial Intelligence as a field encompasses a wide range of technologies and methodologies. At its core, AI seeks to simulate human intelligence through machines. This includes capabilities such as learning, reasoning, problem-solving, and language comprehension. The scope of AI extends to various applications, including natural language processing, computer vision, and robotics. Each of these areas shows immense promise.
Additionally, AI continues to intersect with other technologies, enhancing its potential. For instance, machine learning and deep learning drive significant improvements in data interpretation, leading to smarter systems. Autonomous vehicles, smart assistants, and predictive healthcare AI exemplify the real-life applications of this technology today.
To understand AI comprehensively, it is critical to examine how these technologies fit within societal frameworks. They are shaping industries and influencing policy discussions. As such, AI is not merely a tool; it is a phenomenon that carries implications for all aspects of life.
The Importance of Forecasting in Technology
Forecasting is indispensable in the tech world, particularly as innovations accelerate. In various ways, it functions as a navigational tool, guiding stakeholders through complex landscape of change.
- Guidance for Planning: Companies that engage in effective forecasting can better allocate resources, anticipate market shifts, and strategize future developments. For example, understanding the trajectory of AI can help in talent acquisition and product development.
- Investment Insights: Investors rely on forecasts to identify burgeoning sectors. Accurately predicting an AI's growth not only influences venture capital but also shapes public interest.
- Policy Development: Governments can benefit from AI forecasts to formulate responsible policies. Informed predictions allow for better regulation and governance, ensuring that technological advancements do not outpace ethical standards.
In summary, the role of forecasting transcends simplistic viewpoints. It is about creating a comprehensive narrative around technology that envelops various considerations—from economic ramifications to ethical dilemmas. As AI continues to intrude upon every layer of life, the need to forecast its evolution becomes more pressing.
Current State of Artificial Intelligence
The current state of Artificial Intelligence is characterized by rapid advancements and increasing integration into various sectors. Understanding this state is crucial for several reasons. It allows for an informed analysis of AI’s capacity to solve complex problems and enhances decision-making across industries. Moreover, recognizing the current AI landscape helps stakeholders anticipate the future developments and challenges that may arise. The interplay between technology and ethical considerations is becoming more pronounced, making it imperative to discuss the state of AI today.
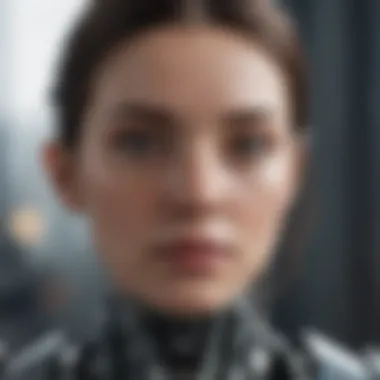
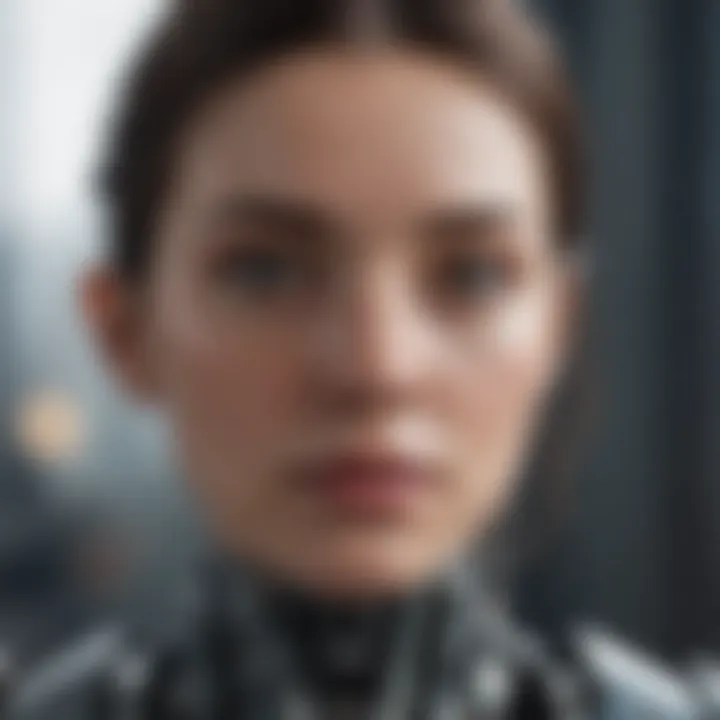
Key Technologies Driving AI Today
Artificial Intelligence today is powered by several key technologies:
- Machine Learning: This subfield enables computers to learn from data patterns without explicit programming. Its ability to adapt and improve algorithms continually makes it a foundation of modern AI applications.
- Natural Language Processing: This technology assists computers in understanding and generating human language. It is essential for applications such as virtual assistants and customer service bots.
- Computer Vision: This technology allows machines to interpret and understand visual information from the world. Vision systems are widely used in sectors including security and healthcare.
- Robotics: Automation through robotics is improving efficiencies in various fields such as manufacturing and healthcare. Robots can perform repetitive tasks with higher accuracy and speed than humans.
These technologies not only enable the development of robust AI systems but also present unique challenges, such as data privacy and ethical considerations.
AI in Various Domains
Healthcare
In healthcare, artificial intelligence is transforming patient care and diagnosis. The use of predictive analytics improves patient outcomes by allowing for early detection of diseases. AI can analyze medical images, facilitating quicker and more precise diagnoses. This technology’s scalability makes it a popular choice in hospitals aiming to enhance treatment protocols.
However, reliance on AI systems can introduce risks, such as misinterpretation of data and potential biases in algorithm training. Therefore, understanding how these systems work and their limitations is essential.
Finance
AI contributes significantly to the finance sector by enhancing fraud detection and risk assessment. Algorithms analyze transaction patterns to flag suspicious activities, making them essential for security. Moreover, AI-driven analytical tools improve investment strategies by predicting market trends based on historical data.
While AI provides financial institutions with tools to optimize operations, it also raises concerns about ethical use and transparency in automated decision-making.
Manufacturing
In manufacturing, AI optimizes supply chain management and quality control. Smart factories incorporate AI to facilitate real-time monitoring and predictive maintenance. This leads to reduced operational costs and enhanced efficiency in production lines.
Despite these benefits, introducing AI into manufacturing can lead to job displacement. It is critical for industry leaders to balance technological advancement with workforce needs.
Transportation
Transportation technology is being revolutionized by AI through advancements such as autonomous vehicles and traffic management systems. AI algorithms help in route optimization, reducing travel times and increases safety on the roads.
Still, challenges such as regulatory hurdles and ethical implications arise with the adoption of such technologies. For instance, questions surrounding liability in the event of accidents involving autonomous vehicles demand careful consideration.
"The integration of AI across sectors demonstrates its vast potential but also emphasizes the need for responsible implementation."
The current state of artificial intelligence is marked by a profound impact on various fields. Each domain benefits from unique AI applications, but they also face distinct challenges that must be navigated with care.
Predictive Models for AI Development
Artificial Intelligence (AI) plays a significant role in shaping future technologies and services. Predictive models are at the core of this development. They allow researchers and professionals to foresee trends and prepare appropriately. Understanding these models is essential as they are influenced by various factors like data quality, algorithm efficiency, and changing market dynamics. The integration of predictive models in AI enhances decision-making, reduces uncertainty, and fosters innovation across industries.
Frameworks for AI Forecasting
Frameworks for AI forecasting serve as structured approaches to understanding and developing AI systems. They offer methodologies for assessing the effects of data inputs on outputs, which helps in refining algorithms for better predictions. Some established frameworks in this domain include:
- CRISP-DM (Cross-Industry Standard Process for Data Mining): This framework outlines key steps, including data understanding and preparation, modeling, evaluation, and deployment. It helps teams manage AI projects from inception through completion.
- KDD (Knowledge Discovery in Databases): This framework emphasizes discovering patterns in data, encompassing data selection, cleaning, and transformation processes before mining the data.
- Agile Methodologies: These frameworks encourage iterative development. Agile practices ensure that teams remain flexible and can adjust to new information or changes in project scope promptly.
Each of these frameworks contributes to a more strategic approach to AI forecasting, aiding organizations in realizing their technological goals while keeping pace with rapid advancements.
Data-Driven Predictions
Data-driven predictions form the backbone of AI advancements. They rely heavily on vast amounts of data and sophisticated algorithms. These predictions help organizations anticipate changes in consumer behavior, market demands, and operational efficiencies. Key elements include:
- Machine Learning Algorithms: These algorithms analyze data to find patterns and make predictions. Techniques such as regression analysis, neural networks, and decision trees are integral to developing accurate models.
- Big Data Analytics: With the surge of data from various sources, businesses utilize big data analytics to transform raw data into actionable insights. This capability allows organizations to forecast trends more accurately.
- Real-Time Analytics: This approach processes data as it comes in. It provides immediate insights, allowing teams to make timely decisions that align with changing circumstances.
Data-driven predictions enhance the strategic outlook of businesses. They allow stakeholders to make informed decisions grounded in empirical evidence rather than assumptions.
"Effective predictive models in AI development initiate proactive strategies that leverage historical data for forward-looking insights."
Ultimately, the role of predictive models is vital in steering AI technologies towards meaningful innovation. The understanding of frameworks and data-driven predictions not only refines current practices but also lays the groundwork for future explorations in AI.
Future AI Innovations
The exploration of future AI innovations is crucial in understanding how artificial intelligence can evolve and shape various sectors in the impending years. As technology continues to advance, AI stands at a pivotal junction where new innovations can either propel advancements or introduce unforeseen challenges. The significance of this topic lies in its ability to forecast not just what is possible but also what is pragmatically feasible. Key elements include the rapid pace of technological development, the potential societal impacts, and the interdisciplinary collaboration necessary to realize the benefits of these innovations.
Emerging Technologies to Watch
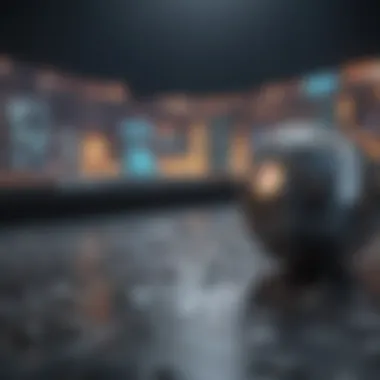
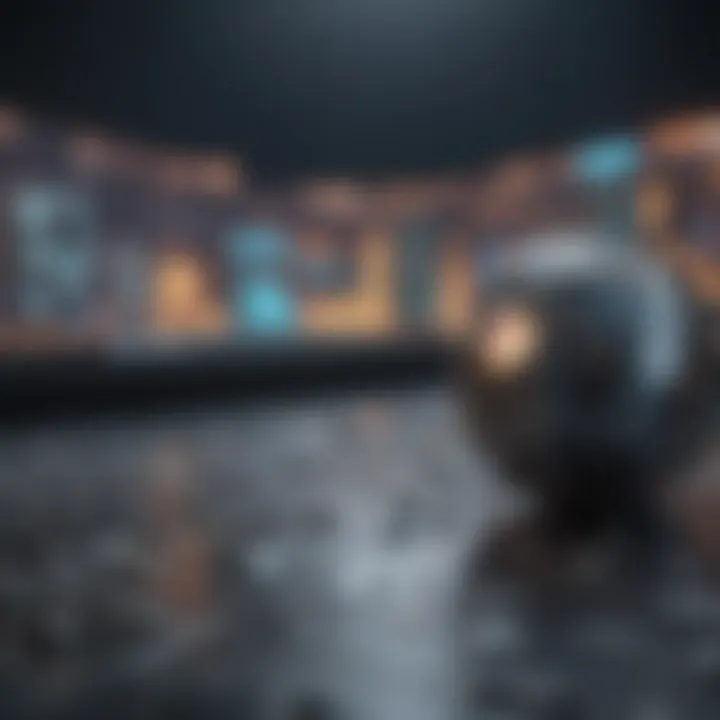
In the landscape of AI, several burgeoning technologies warrant close attention. Among them, machine learning continues to refine itself, incorporating unsupervised and semi-supervised learning approaches that enhance predictive analytics. This progression allows systems to learn from data more effectively.
Another technology is natural language processing, which seeks to improve machine understanding of human language. Innovations in this area will facilitate smoother human-computer interaction, enhancing applications in customer service and personal assistants.
- Adaptive AI: This involves systems that can learn in real time and adapt their behaviors based on user interaction.
- Explainable AI (XAI): As AI implementations grow, so does the demand for transparency in decision-making processes, leading to XAI innovations that make AI outputs understandable to humans.
- AI and IoT: The integration of AI with the Internet of Things enables devices to communicate and make decisions autonomously, improving efficiency.
Each of these technologies not only enhances the capabilities of AI but also poses unique questions regarding ethics and practical application.
The Role of Quantum Computing
Quantum computing represents a groundbreaking shift in computational power, unlocking new possibilities for AI. Traditional computing relies on binary data, but quantum computing uses quantum bits, or qubits, which can exist in multiple states simultaneously. This inherent parallelism gives quantum computers an edge in processing complex algorithms much faster than classical computers.
With the integration of quantum computing, AI could experience leaps in:
- Speed: Tasks that currently take days could be reduced to hours or even minutes.
- Complex Problem Solving: Quantum algorithms might tackle problems in optimization and logistics that are currently intractable.
- Machine Learning: The development of quantum machine learning algorithms could radically enhance the ability to process large datasets, leading to more accurate predictions.
Although still in its nascent stages, the coupling of AI and quantum computing holds transformative potential, challenging traditional paradigms and accelerating innovations. As we look to the future, both emerging technologies and advancements in quantum computing play vital roles in shaping the next chapter for artificial intelligence. They provide pathways toward advancements that can significantly impact various fields and influence everyday life.
"The next decade will see AI not just as a tool but as a partner in solving humanity's most complex challenges."
Strategic Implications of AI Forecasts
Understanding the strategic implications of artificial intelligence forecasts is critical for various stakeholders. This section examines how these forecasts influence economic systems and policymaking, as well as the overall fabric of society. AI's integration into different sectors will create transformative shifts. These shifts bring forth both opportunities and challenges that need careful consideration.
Economic Impacts and Job Displacement
One of the most pressing concerns regarding AI forecasts is the impact on the economy, particularly regarding job displacement. As AI technologies become more sophisticated, they can perform tasks traditionally completed by humans. This transition can lead to significant changes in the labor market. For example, roles in manufacturing have already seen automation reduce the need for manual labor.
- Advantages: There are potential benefits such as increased efficiency and lower operational costs for businesses. Companies can reallocate resources to more strategic positions, focusing on innovation and growth.
- Disadvantages: On the other hand, job displacement can lead to a workforce that is ill-prepared for new roles. Simple jobs may vanish faster than new job creation can occur. Workers in sectors like retail and customer service may find themselves particularly vulnerable.
Governments and organizations need to initiate effective retraining programs. These programs will assist displaced workers in transitioning to new sectors where human-centric skills are required. Addressing these economic impacts is vital for sustaining social coherence in response to significant shifts.
Policy and Governance Challenges
AI forecasts raise significant policy and governance challenges that must be addressed to maximize benefits while minimizing drawbacks. Currently, many regulatory frameworks do not adequately address the complexities introduced by AI technologies. Regulators face challenges in understanding AI's dynamic nature and rapid evolution.
- Policy Development: Governments must develop forward-looking policies that consider the unique attributes of AI. This means engaging with technologists, ethicists, and other stakeholders to craft inclusive policies. Without such measures, there is a risk of falling behind in adaptation to this rapidly changing environment.
- Governance Structures: Establishing governance structures to oversee AI deployment is vital. These structures should focus on ethical use, transparency, and securing user data. Public trust hinges on how well policymakers can create a regulatory environment that protects citizens while encouraging innovation.
As AI continues to permeate various aspects of life, the need for robust policy and governance frameworks becomes ever more pressing.
In summary, understanding the strategic implications of AI forecasts is crucial for navigating the evolving landscape of technology. Economic impacts, especially job displacement, and policy challenges must be approached with a deep sense of responsibility and foresight. Stakeholders across sectors must cooperate to harness AI's potential while safeguarding economic stability and ethical standards.
Ethical Considerations in AI Development
The rapid evolution of artificial intelligence (AI) introduces various ethical considerations that merit thorough examination. As AI systems become more integrated into everyday life, understanding the impact of these technologies is crucial. Ethical considerations in AI development go beyond theoretical discussions; they directly affect society, influencing policies, user experiences, and trust in technology.
One key aspect of ethical AI is the prevention of bias in algorithms. Algorithms learn from data. If the data contains bias, the resulting AI systems may perpetuate discrimination, leading to unfair outcomes in crucial sectors like hiring, law enforcement, and social services. Therefore, developers must prioritize fairness and inclusivity when creating datasets and training algorithms.
Bias and Fairness in Algorithms
Bias in AI systems can arise from various sources. Personal biases exist in the datasets used for training, affecting decisions made by AI. For instance, if an AI system is trained on recruitment data that predominantly reflects a specific demographic, it may favor candidates who fit that profile, neglecting others who may be equally qualified. This can lead to significant implications for diversity and social equity.
To address these biases, organizations should adopt several strategies:
- Diverse Datasets: Ensure that training datasets reflect a broad range of demographics. This minimizes the risk of inherent biases impacting algorithmic decisions.
- Continuous Monitoring: Regularly evaluate AI systems for bias, making adjustments based on new data and changing societal norms.
- Stakeholder Engagement: Involve a diverse group of stakeholders in the development process to gain various perspectives on ethical implications.
By fostering fairness in AI algorithms, developers can enhance the reliability of AI applications while promoting social justice.
Accountability and Transparency
Accountability is another fundamental ethical issue within AI development. Users must trust that AI systems operate fairly and transparently. Lack of accountability can result in mistrust among users, especially if AI decisions have profound implications on their lives.
Transparency involves making algorithms and their decision-making processes understandable to users and stakeholders. When AI systems can explain their reasoning, it enhances trust and allows users to contest decisions they deem unfair.
- Explainable AI (XAI): Develop systems that can articulate their decision-making processes. This can help demystify AI for end-users.
- Regulatory Compliance: Adhere to evolving regulations that demand transparency in AI applications, fostering a culture of responsibility within organizations.
- User Documentation: Provide clear user guidelines regarding how AI systems process information and make decisions, empowering users to understand potential impacts.
Ensuring accountability and transparency is essential for promoting trust in AI technologies, ultimately influencing their adoption and long-term success.
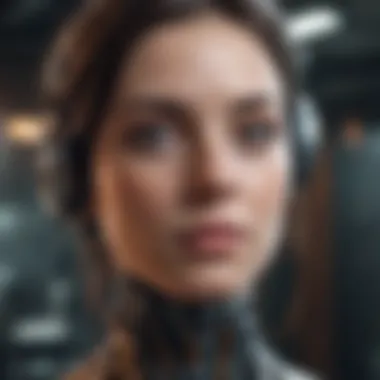
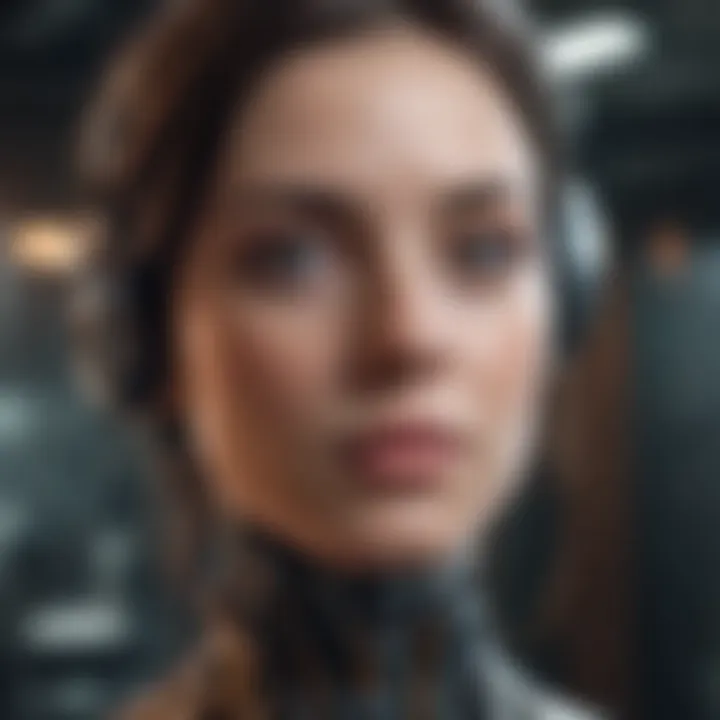
AI's Intersection with Other Disciplines
The intersection of artificial intelligence with various disciplines reveals significant potential for advancements and innovative solutions. This synergetic relationship enhances both the capabilities of AI and the efficacy of the disciplines it integrates with. By collaborating with areas such as data science and environmental science, AI not only broadens its application spectrum but also addresses complex challenges that require interdisciplinary approaches.
The benefits of integrating AI with other fields are numerous. Firstly, AI can bring efficiency and accuracy to data analysis and problem-solving. Secondly, it allows for the amalgamation of diverse knowledge bases, leading to enhanced methodologies and outcomes. Finally, such intersections can yield important insights that are otherwise difficult to achieve in isolation.
Collaboration with Data Science
Data science and AI naturally complement each other. Data science focuses on extracting value from data, while AI uses that data to learn and make predictions. The collaboration enhances the ability to manage vast datasets effectively.
In particular, AI algorithms help to detect patterns and trends that may not be easily visible. This ability is critical in sectors like marketing and finance, where understanding consumer behavior or assessing risk greatly influences decision-making.
Moreover, machine learning techniques, a subset of AI, play a vital role in predictive analytics. They provide the foundation for data models that can accurately forecast outcomes. By integrating AI within data-driven projects, teams can expect:
- Improved accuracy in data interpretation
- Greater speed in processing large datasets
- Enhanced capability for real-time analytics
The relationship between AI and data science is indeed pivotal for advancing knowledge and capabilities in numerous fields.
Integration with Environmental Science
The intersection of AI and environmental science is becoming increasingly important as global concerns about climate change and resource depletion grow. AI offers powerful tools for monitoring and modeling environmental changes, providing insights that can drive policy and conservation efforts.
For instance, machine learning can analyze climate patterns and predict the impact of various factors on ecosystems. AI-driven models help scientists assess risks associated with environmental crises, such as wildfires or floods, enabling timely interventions.
Some specific applications include:
- Analyzing satellite imagery for deforestation tracking
- Modeling pollution dispersion in urban environments
- Optimizing energy consumption through smart grid technologies
The integration of these disciplines not only addresses pressing environmental concerns but also enhances the understanding of complex ecological interactions. As society seeks to develop sustainable practices, this interdisciplinary approach becomes crucial.
"The future of AI applications will increasingly rely on synergy with various scientific disciplines to address global challenges effectively."
Long-Term Predictions for AI
Long-term predictions for artificial intelligence (AI) are critical as they frame the discussions around future implications of technology. Understanding how AI might evolve can assist stakeholders in making informed decisions, steering the development of technology towards beneficial paths. This anticipation involves numerous factors such as technological capabilities, societal needs, and ethical considerations.
AI and Human Interaction
The interaction between AI and humans is a fundamental area of focus in long-term AI predictions. As technologies like natural language processing and machine learning mature, the ways in which humans interact with machines will also evolve. Future applications might include personal AI companions or digital assistants that understand context better than current iterations, providing a more personalized experience.
For instance, imagine a future where AI algorithms can assess emotional cues and provide support in real-time during conversations. This would not only enhance communication but could also serve significant roles in areas such as mental health support and education. Researchers frequently explore the implications of these enhanced interactions, as they could fundamentally change workplace dynamics and personal relationships.
The Singularity Debate
The singularity represents a concept where artificial intelligence surpasses human intelligence and capability. This idea raises numerous questions about control, governance, and the future direction of AI. In the long term, if machines become more capable, they might exhibit autonomy that challenges current ethical frameworks.
Proponents of the singularity argue that it could lead to remarkable advancements, resulting in solutions to diseases or global issues currently beyond our reach. However, this potential includes risks such as the loss of human jobs and ethical dilemmas surrounding decision-making by AI.
Considerations around the singularity reflect deep fears and hopes intertwined with technological progress. Engaging in this debate is essential, as it fosters proactive measures to guide AI development responsibly. These conversations are not just academic; they have real-world repercussions, influencing policy and public perception.
"The implications of AI advancing to a point of singularity require careful consideration from all sectors of society, as they shape the future we will inhabit together."
Culmination: Preparing for an AI-Driven Future
As we move forward in an era dominated by artificial intelligence, it becomes increasingly important to understand how to navigate the challenges and opportunities this technology creates. This conclusion emphasizes the critical need for stakeholders to strategically prepare for an AI-driven future, where the impact of AI will reshape various aspects of life including industry practices, governance, and even personal experiences. Decision-makers, developers, and educators must align their strategies accordingly, fostering an environment that supports responsible and innovative AI deployment.
The potential benefits of AI are vast. It can lead to significant enhancements in efficiency, productivity, and decision-making across different sectors. However, alongside these advantages come considerations that must be addressed proactively. This includes managing job displacement, ensuring ethical standards, and promoting equitable access to AI technologies. Stakeholders must be vigilant in recognizing both the benefits and challenges to create a balanced and sustainable approach.
Successful integration of artificial intelligence requires a clear vision that engages all levels of society and prioritizes ethical implications alongside technological advancements.
Strategies for Stakeholders
Implementing effective strategies is crucial for stakeholders to ensure the responsible adoption of AI. Here are some recommended strategies:
- Investing in Education and Skills Development: Stakeholders should prioritize training programs. This can equip the workforce with necessary skills tailored for a technology-driven future. Fostering an adaptable workforce can minimize the adverse effects of job displacement.
- Fostering Collaboration: Encourage partnerships among industry leaders, governments, and academia. Collaborative efforts can lead to shared knowledge, innovation, and comprehensive solutions to pressing AI challenges.
- Creating Robust Ethical Guidelines: Formulating ethical frameworks is essential. These guidelines should address issues such as bias, transparency, and accountability in AI applications. This responsiveness is crucial to sustaining public trust.
- Engaging in Public Dialogue: Encourage discussions around AI technologies. Engaging the public can help demystify AI and foster a broader understanding of its benefits and risks. This approach nurtures a more informed society.
Embracing Continuous Learning
Continuous learning is vital in the rapidly evolving field of artificial intelligence. Stakeholders should adopt the following practices:
- Lifelong Learning Initiatives: Develop programs that promote lifelong learning. This can help individuals stay abreast of emerging trends and technologies in AI, ensuring they remain competitive in their respective fields.
- Staying Updated with Research: Engage with the latest research findings and innovations. Regularly reviewing scientific literature and participating in professional networks can provide insights that support informed decision-making.
- Experimentation and Adaptation: Encourage a culture of experimentation. Organizations should be willing to test new AI applications and adapt their strategies based on outcomes. This flexibility can lead to breakthroughs in how AI is utilized.
- Fostering an Inquisitive Mindset: Cultivating curiosity encourages exploration. Stakeholders should promote an environment where questioning and exploration of AI technologies is welcome. This mindset is essential for driving innovation and development.
By taking these actions, stakeholders can better prepare for an AI-driven future, transforming potential challenges into opportunities.