Analyzing Car Traffic Data for Urban Mobility Insights
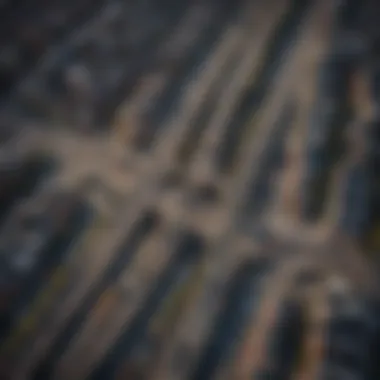
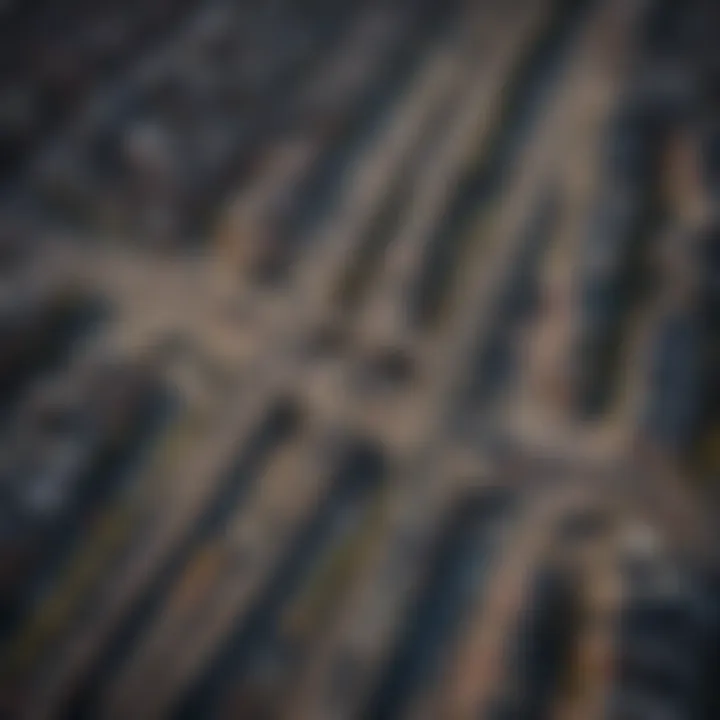
Intro
The examination of car traffic data plays a crucial role in shaping urban environments. Understanding traffic flow patterns informs city planners and policymakers about current mobility trends and identifies areas that require attention. As cities grow and evolve, so do the challenges concerning transportation infrastructure and safety. In this context, analyzing traffic data becomes not just a mathematical exercise but a necessity for ensuring efficient and safe urban spaces.
This article aims to explore traffic data's multifaceted influences, methodologies for collecting accurate information, and the implications this data has on environmental sustainability. Emerging technologies are also reviewed, providing insights into smarter transportation systems. Each section is intended to contribute to a cohesive narrative about how traffic data can lead to better urban planning and development strategies.
Prolusion to Car Traffic Data
The analysis of car traffic data holds significant importance in todayβs rapidly urbanizing world. Understanding patterns in car traffic is essential for effective transportation planning, infrastructure development, and improving road safety. This section underlines its crucial role in contemporary urban mobility, indicating benefits such as enhancing commuting efficiency, reducing traffic congestion, and addressing environmental impacts associated with vehicular emissions.
Definition and Importance
Car traffic data encompasses various metrics that detail the movement and behavior of vehicles on roadways. By analyzing this data, city planners and transportation agencies can derive essential insights into traffic flow, volume, speed, and patterns over time. For instance, knowing peak traffic hours aids in optimizing traffic signal timings, thereby reducing delays and emissions.
The importance of car traffic data extends beyond mere statistics. It informs decision-making processes that ultimately affect public policy, resource allocation, and infrastructure investments. Furthermore, accuracy in these data is paramount, as it represents not just numbers but the daily experiences of commuters. The implications of traffic data thus stretch into areas like public health, environmental sustainability, and economic efficiency.
Historical Perspectives
The evolution of car traffic data can be traced back to the increasing recognition of urban growth and the accompanying challenges in traffic management. Initially, traffic counts were conducted manually, with inspectors recording vehicle numbers at specific locations over set periods. However, technological advancements have transformed this field significantly.
In the 1960s, the advent of electronic counting devices marked a turning point, allowing for real-time data collection and analysis. Over the decades, improvements in digital technology and data analytics have further enhanced our ability to understand car traffic trends. For instance, GPS tracking and mobile applications now provide granular data regarding vehicle movements, paving the way for predictive modeling and deeper insights into traffic behavior.
The historical context not only illustrates the progression of traffic data methodologies but also underscores the increasing importance placed on this information as urban populations continue to rise. Understanding the past equips stakeholders with the knowledge needed to tackle the burgeoning challenges of modern transportation systems.
Types of Car Traffic Data
Understanding the types of car traffic data is essential for any analysis related to urban planning and transportation policies. Each type offers unique insights that can benefit city planners and traffic management professionals. By examining these various data types, we can get a clearer picture of traffic behavior, patterns, and potential areas for improvement. Below, we will detail three main data types: volume data, speed data, and flow data. These categories are crucial for making informed decisions.
Volume Data
Volume data refers to the total number of vehicles passing a specific point within a certain timeframe. This data is fundamental because it gives a first-level assessment of traffic demand on roads. High volume in a particular area can indicate congestion issues, leading to potential investments in infrastructure upgrades or alternative routes to alleviate traffic.
Several methodologies exist for collecting volume data. Automated traffic counters, like the ones from Miovision and Pioneer Electronics, are often employed for continuous monitoring. Manual counting is also prevalent, although it may be less reliable due to human error.
Key benefits of studying volume data include:
- Identifying Traffic Patterns: Understanding peak traffic hours helps in planning resources efficiently.
- Policy Formulation: Insights from volume data guide policymakers in regulating traffic flow and implementing necessary policies.
- Infrastructure Investment: Identifying high-traffic areas can justify investments in new roads or public transport systems.
Speed Data
Speed data captures how fast vehicles travel on specific road segments. Analyzing speed is important because it reflects road conditions and driver behavior. Variations in speed can indicate road issues, such as potholes or curves that may not be well-marked.
Speed data can be collected through radar sensors, GPS devices, or speed cameras installed along roadways. For instance, companies like Kistler and TrafficData offer innovative technologies that aid in gathering this type of information.
The significance of speed data encompasses:
- Safety Assessments: Identifying areas where speed is consistently too high can help in deploying traffic calming measures.
- Regulatory Compliance: Evaluating speed data assists law enforcement with enforcing traffic laws.
- Performance Metrics: It allows city planners to measure the efficiency of their traffic management strategies over time.
Flow Data
Flow data examines the rate at which vehicles travel through a given point. It combines both volume and speed data to offer a comprehensive view of traffic dynamics. This data helps to determine how many vehicles can pass through intersections or segments of road within a specific time frame.
Collecting flow data requires advanced equipment such as inductive loops or video cameras that track vehicle movements. Both Sensys Networks and TrafficLinx provide solutions for managing flow data effectively.
Benefits of flow data analysis include:
- Resource Optimization: This data helps in optimizing traffic signal timings, enhancing the overall efficiency of road networks.
- Predictive Modeling: Utilizing flow data enables analysts to forecast traffic scenarios, which can assist in future planning efforts.
- Mitigating Congestion: Continuous monitoring and adjustments based on flow data can significantly reduce congestion in urban centers.
"Traffic data analysis is not merely about numbers. It's about understanding the dynamics of urban mobility."
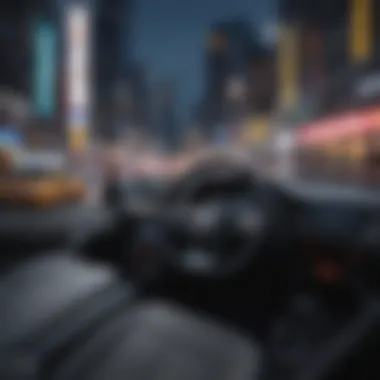
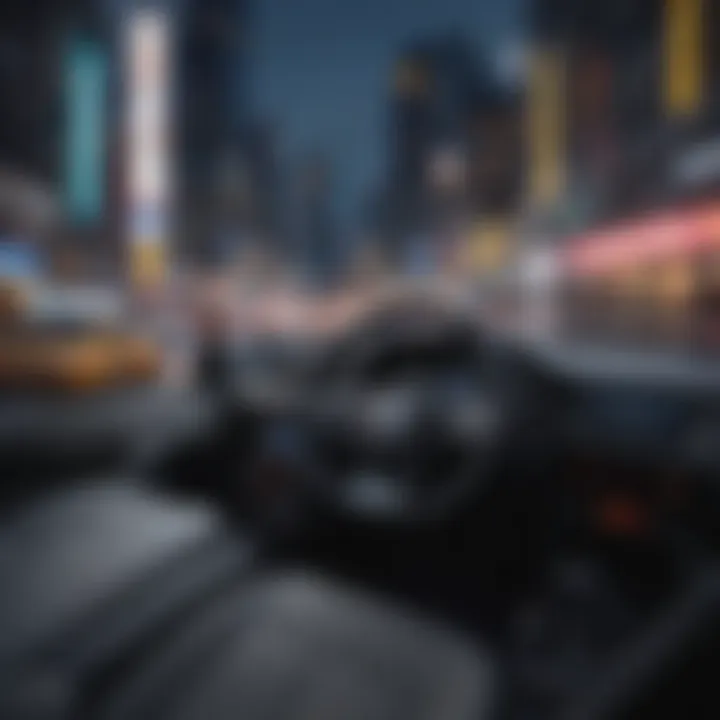
By focusing on volume, speed, and flow data, we can acquire a holistic view of car traffic, paving the way for improved infrastructure and sustainable transportation solutions.
Methods of Collecting Traffic Data
Understanding how data about car traffic is collected is crucial for effective analysis and application. The choice of method can shape the data's reliability, accuracy, and usability. Various methods exist, and each provides unique benefits and challenges. An exploration of these methods reveals how they contribute to our overall comprehension of traffic patterns and behaviors.
Traffic Counting Equipment
Traffic counting equipment consists of various tools designed to measure vehicle volume, speed, and classifications on the road. Examples include inductive loop sensors, infrared sensors, and video cameras.
- Inductive Loop Sensors: These sensors install beneath road surfaces to detect vehicles through changes in inductance. Their accuracy is high, but installation requires roadwork and maintenance.
- Infrared Sensors: These devices use infrared light beams to count vehicles as they pass through a designated area. They are easier to install but can incur accuracy issues in poor weather conditions.
- Video Cameras: Cameras offer a versatile option for traffic data collection. They can monitor not just vehicle counts but also driver behaviors. However, concerns about privacy can arise.
Overall, using traffic counting equipment is essential for creating real-time databases, helping city planners make informed decisions regarding traffic flow and infrastructure needs.
Surveys and Manual Data Collection
Manual data collection methods include on-site surveys and observational studies. While these may seem outdated compared to automated equipment, they still play a vital role.
- On-Site Surveys: Conducting surveys at specific locations allows researchers to gather qualitative data regarding driver behaviors and motivations. This type of data adds context that raw numbers alone cannot provide.
- Observational Studies: Trained personnel observe and record traffic patterns over a defined period. This manual approach can highlight specific issues like road safety challenges or peak traffic times.
Both techniques focus on direct human interaction, which adds a valuable layer of insight. However, they require significant time and resources and may face limitations in scale.
Emerging Technologies in Data Collection
Recent advancements in technology have revolutionized the field of traffic data collection. Methods like drone surveillance, mobile applications, and crowdsourcing contribute fresh perspectives to traffic monitoring.
- Drone Surveillance: Drones can cover large areas rapidly, providing real-time aerial data on traffic conditions. They are especially useful in monitoring accidents or delays.
- Mobile Applications: Apps like Google Maps utilize user-generated data to provide live traffic insights. These platforms can help gauge road conditions and predict future traffic flows.
- Crowdsourcing: Collecting data from various users through reports and feedback can enhance the richness of traffic databases. This offers spontaneous information that traditional methods might miss.
The integration of these technologies yields comprehensive datasets that enhance traditional methods' effectiveness. As research continues, the potential for more sophisticated traffic data collection methods increases.
Analyzing Car Traffic Data
Analyzing car traffic data is essential for developing effective transportation systems and infrastructure. It sheds light on patterns of movement, vehicle types, and peak travel times, thus guiding numerous stakeholders. Professionals in urban planning, traffic engineering, and environmental science utilize these insights to improve road safety and efficiency.
One key element in this analysis is the ability to identify trends over time. By evaluating historical traffic volumes alongside real-time data, decision-makers can predict future conditions. This predictive capability helps anticipate congestion, which is critical for timely interventions. Furthermore, analyzing this data aids in the crafting of policies that promote sustainable transportation solutions.
Another significant consideration in analyzing car traffic data is how it can impact public safety. Studying accident hotspots, for instance, enables authorities to implement safety measures. Improved signage, road infrastructure changes, and traffic law enforcement initiatives can stem from accurate traffic data analysis.
Data Cleaning and Preparation
Data cleaning and preparation serve as the foundational step in traffic data analysis. Raw traffic data often contains inaccuracies or inconsistencies, which can arise from various sources, such as sensor malfunctions or human errors in manual collection methods. Therefore, it is crucial to address these issues before proceeding with any analytical techniques.
The data cleaning process typically involves several actions:
- Identifying Missing Values: Missing values can distort analysis. Strategies to handle them include imputation or removal, depending on the data set's size and significance.
- Outlier Detection: Identifying and addressing outliers is necessary to ensure that extreme values do not skew results. Techniques such as z-scores or IQR can help flag these anomalies.
- Standardizing Formats: Consistency in data format (for example, time and date formats) is important for accurate analysis. This includes converting all measurements into the same units.
Proper preparation of cleaned data enhances the reliability of the subsequent analysis phase.
Statistical Methods and Tools
Statistical methods and tools are vital in the analysis of car traffic data. They transform raw data into actionable insights. Various techniques can be employed, each serving different purposes according to the research goals.
- Descriptive Statistics: These methods summarize the basic characteristics of data. Metrics such as mean, median, and mode provide insight into average traffic patterns and help identify behavioral trends.
- Regression Analysis: This technique examines relationships among variables, such as how traffic volume affects travel time. Linear regression is commonly used to predict outcomes and inform decision-making.
- Time Series Analysis: Analyzing data collected over time helps to uncover seasonal patterns or long-term trends. This is crucial for predicting future traffic conditions based on past data.
- Geospatial Analysis: Utilizing Geographic Information Systems (GIS) can reveal spatial relationships within traffic data, such as the impact of urban areas on traffic congestion.
- Visualization Tools: Graphical representations like charts and heat maps allow stakeholders to interpret complex data intuitively.
These statistical methods and tools enhance the clarity and depth of insights derived from car traffic data, thus improving transportation strategies and policy decisions.
Applications of Traffic Data Analysis
Traffic data analysis plays a crucial role in various aspects of urban life. Understanding car traffic data allows cities to improve their infrastructure, optimize traffic management systems, and enhance safety measures. These applications are not just theoretical; they directly affect how people navigate cities, the effectiveness of public transport, and overall urban development.
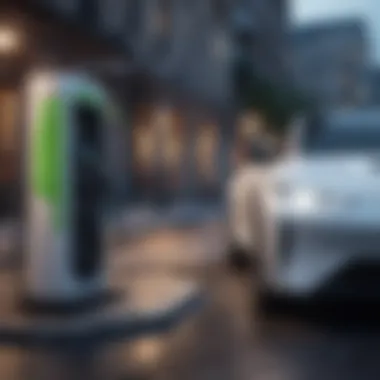
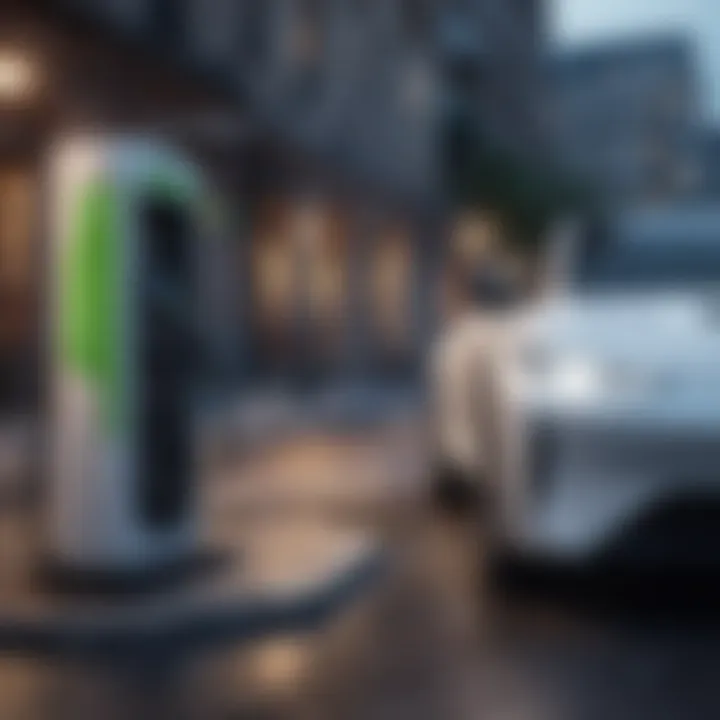
Urban Planning and Infrastructure Development
Urban planning relies significantly on traffic data. Accurate traffic data informs planners about current patterns and predicts future needs. For instance, analyzing traffic volume can lead to identifying bottleneck areas that often result in congestion. By identifying these areas, cities can make evidence-based decisions to expand roads or improve public transport.
Moreover, data on traffic flow can help in determining the timing of traffic lights to facilitate smoother transit through intersections. Moreover, understanding peak times for traffic can assist in planning new facilities or services, such as shopping centers or housing developments. Research shows that cities which effectively use traffic data can reduce congestion by up to 30%.
"Urban mobility concepts are changing; reliable data is key to adaptations in planning and management."
Traffic Management Systems
Traffic management systems benefit immensely from real-time traffic data. Monitoring data allows operators to adapt on-the-fly. For example, when traffic volume spikes unexpectedly, systems can quickly alter traffic light timings or suggest alternative routes to drivers to alleviate congestion. This immediate capability has the potential to cut down on travel time significantly.
In addition, integrating Advanced Traffic Management Systems (ATMS) can result in improved coordination between traffic signals, reducing delays across the board. Modern applications that leverage Artificial Intelligence and machine learning can predict and respond to traffic movements, adjusting controls according to real-time conditions.
Safety Improvement Strategies
Safety on the roads is paramount. Traffic data analysis provides valuable insights into accident hotspots. Data can help identify locations with high incidence rates. By analyzing this data, officials can implement targeted safety measures like better signage, improved lighting, or altered road layouts.
Additionally, conducting thorough analyses of road user behaviors can inform the design of effective public awareness campaigns aimed at reducing accidents. This proactive approach ensures that resources are allocated effectively, based on empirical evidence rather than assumptions. Statistics substantiate that areas implementing traffic safety reforms based on data can see a decrease in traffic-related injuries by 25% or more.
In summary, the applications of traffic data analysis extend beyond just understanding traffic patterns. They touch every facet of urban planning, traffic management, and safety improvement. This interconnectedness demonstrates the indispensable role that traffic data plays in fostering effective and sustainable urban living.
Impact of Traffic Data on Environmental Issues
The relevance of traffic data in addressing environmental issues cannot be overstated. Understanding how vehicles circulate within urban spaces aids in grasping their environmental impact, particularly regarding congestion and emissions.
Efficiently collected data informs policies aimed at reducing vehicular emissions while promoting sustainable practices. Traffic data is an essential tool in pursuing environmental goals, specifically in identifying patterns that lead to increased fuel consumption and unnecessary emissions. The integration of this data with environmental monitoring helps policymakers make well-informed choices that promote eco-friendly transportation methods.
Congestion and Emissions
Traffic congestion is not merely a nuisance; it presents significant environmental challenges. As vehicles idle in traffic, they produce higher emissions, contributing to air pollution and climate change. Research shows that stop-and-go conditions lead to up to 30% more fuel consumption compared to smooth driving. Using traffic data, cities can identify congestion hotspots and deploy targeted interventions to alleviate the strain on roadways.
For instance, real-time traffic data can help optimize traffic signal timings, thus reducing the time vehicles spend idling at intersections. By analyzing historical data, authorities can predict when and where congestions are likely to occur, allowing for proactive measures to enhance traffic flow.
Key benefits of addressing congestion include:
- Reduced greenhouse gas emissions: Less time on the road means lower emissions.
- Improved air quality: Decreased pollution levels contribute to better public health outcomes.
- Economic savings: Reducing traffic jams not only saves fuel but can also decrease commute times, bolstering productivity.
Sustainable Transportation Solutions
Traffic data plays a critical role in fostering sustainable transportation solutions. By analyzing patterns in vehicle usage, cities can design initiatives that encourage public transit, cycling, and walking.
Emerging trends in urban planning advocate for a shift towards multi-modal transport systems, effectively integrating various transportation modes to minimize reliance on single-occupancy vehicles. Such integration results in a comprehensive transportation network, which is more sustainable and capable of serving diverse user needs.
Additionally, traffic data can help assess the effectiveness of these solutions over time. By tracking changes in traffic volume and emission levels, agencies can refine their strategies to enhance user adoption of environmentally-friendly transport.
Examples of sustainable practices informed by traffic data include:
- Carpooling incentives: By analyzing peak travel times, cities can promote carpooling initiatives effectively.
- Bike lanes: Identifying high-traffic areas allows for better placement of bike lanes, enhancing safety and usage.
- Public transport reliability: Data analysis can lead to improved schedules and routes, making public transit a more attractive option.
Challenges in Traffic Data Collection and Analysis
The process of collecting and analyzing traffic data presents numerous challenges that are crucial for understanding the reliability of outcomes drawn from such information. Accurate traffic data is the backbone of effective urban planning, policy making, and safety improvements. However, the methods used to gather and analyze this data are not without significant issues.
Data Privacy Concerns
To analyze car traffic data, agencies often collect information from a variety of sources, including GPS tracking, mobile applications, and traffic cameras. One of the ongoing concerns is data privacy. With increased capabilities to collect detailed and sometimes sensitive information about individual patterns and behaviors, there is a risk of infringing on personal privacy. Inappropriate use of data can lead to mistrust among the public, which can ultimately affect participation in data collection efforts. It is essential for organizations to develop protocols that safeguard user data and ensure that any data collected is anonymized and used only for stated purposes. Regulatory frameworks, such as the General Data Protection Regulation (GDPR) in Europe, are vital in shaping how data is collected and stored. Awareness and transparency about these practices not only protect individuals but also help establish trust in data-driven strategies.
Data Accuracy and Reliability
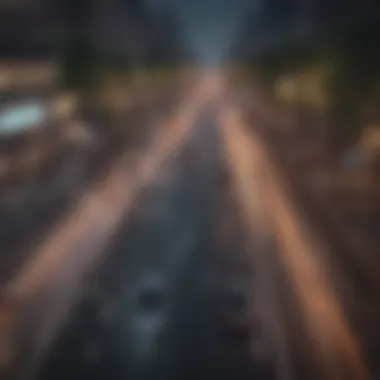
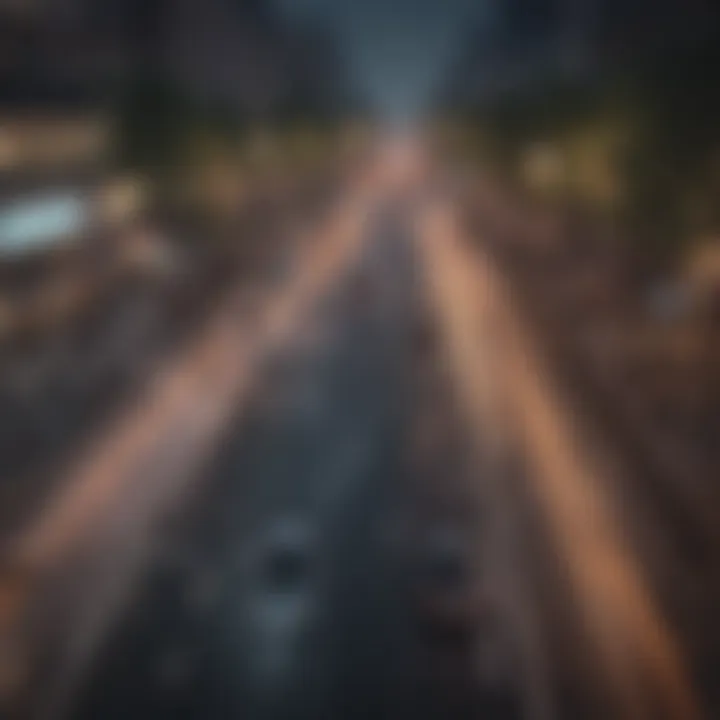
The accuracy and reliability of traffic data is another significant challenge. Various factors can distort data quality, leading to misinterpretation and flawed conclusions. Equipment failures, human errors in manual data collection, and external conditions such as weather can all impact the quality of traffic data. For instance, traffic counters might miscount or fail to register certain vehicles due to maintenance issues. Such inaccuracies can have far-reaching implications for urban planning and traffic management.
To mitigate these issues, it is crucial for agencies to employ multiple data validation techniques. Cross-referencing information from varied sources can ensure a more comprehensive understanding of traffic patterns. Moreover, investing in higher quality data collection technologies, such as infrared or radar sensors, can yield more accurate results. Calibration and regular maintenance of equipment also play a crucial role.
"The efficacy of traffic data analysis hinges significantly on the commitment to data integrity and the ethical standards applied in its collection and use."
Understanding and tackling these issues are foundational steps toward optimizing the management and application of traffic data.
Future Trends in Traffic Data Management
Future trends in traffic data management highlight the dynamic intersection between technology and urban mobility. Understanding these trends is crucial for stakeholders aiming to optimize road usage, enhance safety, and reduce environmental impacts. New methodologies are becoming available that leverage advanced technologies for data collection and analysis. This section discusses three major trends: the integration of smart technologies, predictive analytics in traffic management, and public engagement through technology.
Integration of Smart Technologies
The integration of smart technologies is transforming traffic data management. Devices such as IoT sensors and connected vehicles allow for real-time data collection. These technologies gather information about vehicle speed, traffic flow, and even driver behaviors. The insights generated can help traffic agencies monitor conditions, predict congestion, and implement timely interventions.
Some beneficial smart technologies include:
- Traffic Cameras: Used for monitoring flow and identifying incidents.
- Smart Traffic Signals: These adapt to real-time traffic conditions to optimize vehicle movement.
- Mobile Applications: Allow users to receive real-time traffic updates.
Such technological advancements promise improvements in both traffic efficiency and safety. However, there are also considerations regarding the maintenance and security of these systems that authorities must address.
Predictive Analytics in Traffic Management
Predictive analytics is becoming an essential tool for traffic management. By utilizing historical data and machine learning algorithms, traffic managers can forecast patterns and behaviors. For example, predictive models can determine peak traffic times and identify potential accident hazards.
Some key advantages include:
- Enhanced Decision Making: Insights from predictive models can inform road planning and resource allocation.
- Accident Mitigation: Early identification of high-risk conditions allows for proactive safety measures.
- Improved Public Services: Traffic services can adapt and respond more efficiently to changing patterns.
As traffic patterns continue to evolve, the use of predictive analytics will provide valuable foresight for both urban planners and policy makers.
Public Engagement through Technology
Public engagement is critical in effectively managing traffic and urban mobility. Technology provides platforms for community involvement and feedback. Social media, mobile apps, and collaborative websites empower the public to report traffic conditions or hazards. User-generated data can also inform urban planners about local experiences and perspectives.
Benefits include:
- Increased Awareness: Communities become more informed about traffic conditions.
- Collaborative Solutions: Gathering community input may lead to innovative solutions for local traffic issues.
- Enhanced Trust: City traffic departments can build public trust through transparency and responsiveness.
The End
The conclusion serves as a vital component of this article by encapsulating the intricate journey through car traffic data and its implications for urban planning, transportation policy, and environmental sustainability. It highlights how data plays a crucial role in shaping modern transportation systems, guiding decision-makers to develop solutions that address current challenges in mobility.
By summarizing the key insights, this section reinforces the necessity of comprehensive traffic data analysis to better understand urban mobility trends. It helps readers recognize the benefits of employing meticulous methodologies in data collection and analysis. Traffic data not only assist in devising effective traffic management strategies but also hold the potential to enhance road safety and guide infrastructure development.
Moreover, this conclusion invites reflection on key considerations such as the ethical implications of data use, the impact of emerging technologies, and the necessity of integrating public engagement in traffic data initiatives. Addressing these elements is crucial for ensuring that traffic data analysis remains relevant and beneficial for society at large.
"Understanding and strategically utilizing car traffic data can lead to more effective policies and a significant reduction in urban congestion."
In recapping the vital themes, this conclusion underscores that traffic data is not merely numbers but represents the pulse of urban mobility. By looking forward, we see a landscape where continuous improvements in data management will yield smarter, more resilient cities.
Summary of Insights
Throughout this article, we have explored various aspects of car traffic data. The insights gathered reveal:
- The multifaceted types of data, including volume, speed, and flow, that inform traffic analysis.
- Different methods for collecting data effectively, emphasizing the role of technology in enhancing data quality.
- Applications of traffic analysis which impact urban planning and safety improvements.
- The crucial link between traffic data and environmental sustainability.
- Emerging challenges in maintaining data accuracy and privacy concerns.
Recognizing these insights is instrumental for students, researchers, educators, and professionals working in transportation and urban studies.
The Path Ahead
Looking to the future, the management of traffic data is poised to evolve significantly. Several key trends are emerging:
- Integration of Smart Technologies: Advanced sensors and real-time data processing will become commonplace, offering immediate insights into traffic conditions.
- Predictive Analytics: Utilizing historical data to forecast traffic patterns will allow for preemptive actions, enhancing congestion management.
- Public Engagement: Involving the community through apps and platforms can provide valuable input and fosters transparency in data usage.
These developments could not only improve traffic flow but also promote sustainable solutions to urban mobility issues. The path ahead emphasizes that a strategic approach, rooted in thorough analysis and community involvement, is essential for cities as they navigate the complexities of modern transportation systems.