AI in Agriculture: Innovations for a Sustainable Future
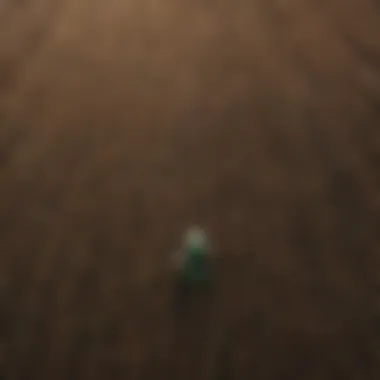
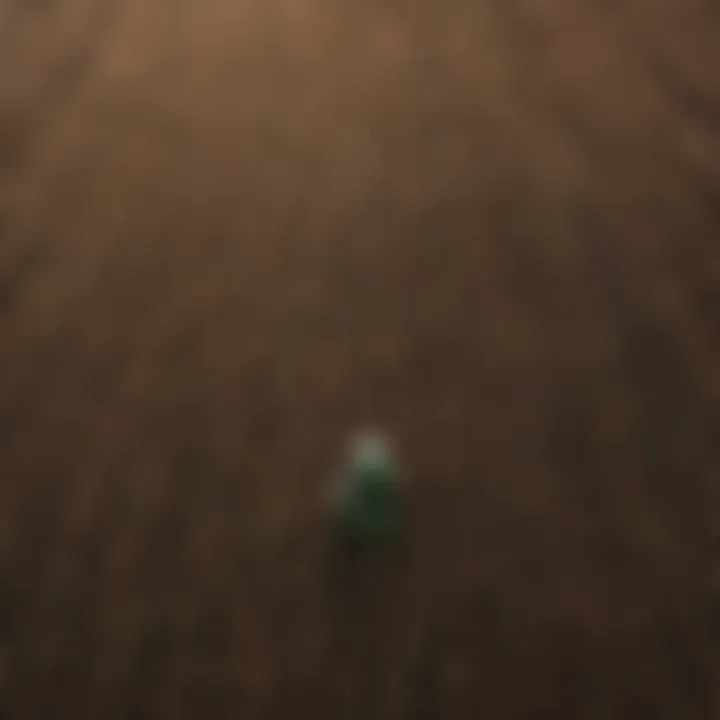
Intro
The incorporation of artificial intelligence (AI) into agriculture is reshaping the landscape of farming. It brings not only a technical evolution but also drastic changes in methodologies, productivity metrics, and sustainability practices. The integration of AI provides farmers with tools to optimize their operations. This is crucial because efficient farming is directly linked to food security and environmental concerns.
As agriculture faces pressures from climate change and population growth, AI offers solutions through precision agriculture, advanced crop management, and predictive analytics. These innovations enable farmers to make data-driven decisions that enhance yield while minimizing resource waste. In exploring the nuances of these developments, this article aims to provide a comprehensive overview of how AI is fundamentally transforming the agricultural sector.
Methodology
Study Design
This examination employs a comparative approach. It analyzes various case studies, highlighting successful implementations of AI technologies in farming. The focus is on practical applications, measured outcomes, and the challenges encountered during integration. This design allows a clear understanding of the impact of AI in diverse agricultural contexts.
Data Collection Techniques
Data for this study was garnered through multiple techniques:
- Field Observations: Direct observations of AI tools utilized by farmers.
- Interviews: Engaging with experts, including agronomists and AI developers.
- Surveys: Collecting quantitative data from farmers on AI adoption rates and levels of satisfaction.
Additionally, secondary data from published studies and industry reports enriches the analysis. Such a multifaceted approach ensures a well-rounded perspective on the intersection of AI and farming.
Discussion
Interpretation of Results
The research indicates a significant enhancement in crop yields and operational efficiencies due to AI. Farmers deploying precision agriculture technologies, such as sensor-based soil monitoring and AI-driven pest management, report noticeable benefits. Also, the predictive models assist in anticipating climate impacts, thereby optimizing planting schedules.
"AI is not just a tool; it is a partner for farmers aiming for sustainability and efficiency."
Limitations of the Study
While the findings are promising, there are limitations. The study relies heavily on case studies from specific regions, which may not translate universally. Some farmers may face resource constraints when adopting AI solutions, leading to discrepancies in outcomes. Furthermore, the rapid pace of technological advancement makes it challenging to capture all dimensions of AI's impact.
Future Research Directions
Further research is required in the following areas:
- Longitudinal studies tracking the long-term impact of AI on agricultural productivity
- Exploration of economic barriers for smallholder farmers in adopting AI technologies
- The role of policy in facilitating or restricting AI integration in farming
By addressing these aspects, future studies can provide deeper insights into the multifaceted effects of AI on agriculture and ensure inclusive growth in the sector.
Preamble to AI in Agriculture
The integration of Artificial Intelligence (AI) in agriculture marks a turning point in how we approach farming and food production. This intersection offers numerous advantages, enhancing efficiency, sustainability, and overall productivity. Understanding the role of AI within agricultural practices is critical for identifying avenues for innovation and future development.
AI technologies present a range of applications that can optimize resource allocation and improve decision-making. As global populations rise and climate challenges escalate, traditional farming methods alone may not suffice. The incorporation of AI can lead to better crop yields, reduced waste, and healthier ecosystems. The knowledge of how AI operates in this field allows stakeholders to leverage its potential fully, leading to smarter agricultural practices.
The importance of this topic extends to multiple areas. For practitioners in agriculture, grasping AI concepts can inform more strategic approaches to crop management and resource utilization.
Students and researchers may find value in analyzing data-driven methodologies that AI introduces. Understanding the mechanics behind AI can also prepare future professionals for the challenges facing the agriculture sector. Therefore, this exploration sets the stage for deeper discussions on specific applications of AI technologies in farming.
Defining Artificial Intelligence
AI refers to the simulation of human intelligence in machines programmed to think and learn. These systems can analyze data, recognize patterns, and make autonomous decisions based on input. In agriculture, AI leverages data from multiple sources, such as soil health, weather patterns, and crop performance. This information enables farmers to optimize their operations significantly.
AI functions through various methodologies, including machine learning and predictive analytics. As agriculture becomes increasingly data-driven, defining AI's capabilities becomes essential. The practical implementations of AI in farming can vary from automated tractors to sophisticated irrigation systems, all contributing towards achieving unprecedented levels of efficiency.
Historical Context of AI in Farming
The historical track of AI in agriculture spans decades. Early technological advancements, like simple automation tools, laid the groundwork for more sophisticated AI integration. However, it was not until the late 20th century that developments in computing power and data analysis catalyzed broader applications of AI in this field.
Initially, AI's application was limited to basic tasks. As technology evolved, more complex systems emerged, allowing for advanced data processing. The rise of precision agriculture in the 2000s signaled the beginning of widespread AI adoption. Farmers began utilizing AI-driven technologies for tasks ranging from soil monitoring to yield prediction. The evolution continues as more tools are made available, pushing the limits of agricultural efficiency.
Today, AI stands as a transformative force in farming, changing the landscape of agriculture globally. This transformation reflects not only technological advancement but also a shift in how we perceive and manage natural resources.
Current AI Technologies Used in Farming
The integration of AI technologies in agriculture marks a turning point in how farming practices operate. As the world faces challenges like climate change, population growth, and diminishing natural resources, current AI technologies are not just an asset, they are becoming a necessity. These technologies improve efficiency, enhance yield, and promote sustainability in ways traditional methods cannot achieve alone.
AI plays a critical role in analyzing large data sets to inform decisions that affect crop yield, pest management, and resource allocation. The benefits of these technologies are multifaceted. For example, they provide more precise data, reduce waste, and optimize the use of inputs such as water and fertilizers. This leads to better crop management and ultimately, more fruitful harvests.
Machine Learning Applications
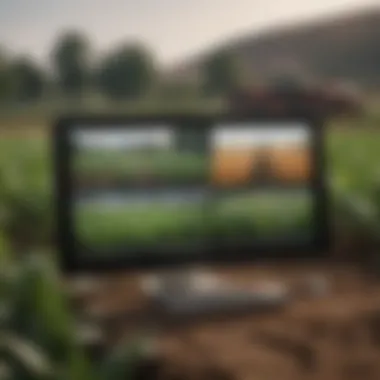
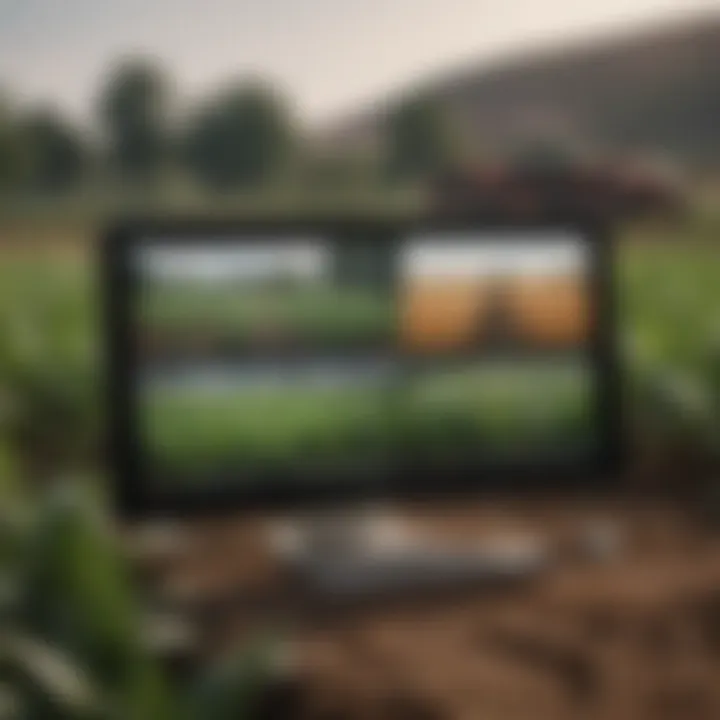
Machine learning is at the forefront of AI technology in farming. It involves utilizing algorithms that can learn from and make predictions based on data. This capability is utilized in various farming applications, such as analyzing soil health, predicting weather patterns, and optimizing planting schedules.
For instance, machine learning models can analyze historical crop data to forecast yields accurately. These forecasts are important for farmers as they help in planning resources effectively. Notably, algorithms can adapt over time, refining their predictions. This adaptability makes machine learning a powerful tool for ongoing improvement in agricultural practices.
Key Benefits:
- Enhanced prediction accuracy
- Continuous learning from new data
- Customized farming solutions
Computer Vision in Crop Monitoring
Computer vision employs AI to interpret and analyze visual data from fields. This technology uses images captured by cameras and satellites to monitor crop conditions in real-time. By identifying issues like disease and nutrient deficiencies early, farmers can take immediate action, minimizing damage and loss.
Computer vision systems are often combined with machine learning to improve their effectiveness. For example, they can analyze aerial images to detect stress in crops before symptoms are visibly noticeable to the human eye. This early detection is crucial for addressing problems before they escalate into larger issues.
Applications Include:
- Early detection of diseases
- Assessing nutrient levels
- Monitoring growth progress over time
Drones and Autonomous Vehicles
Drones and autonomous vehicles represent another significant advancement in AI technologies for farming. These tools provide farmers with capabilities that were once unimaginable. Drones are used for aerial surveillance, facilitating crop mapping and soil analysis efficiently.
Autonomous vehicles, such as tractors and harvesters, are equipped with AI to navigate fields, sow seeds, and harvest crops without human intervention. This technology not only increases efficiency but also reduces labor costs.
Drones and autonomous vehicles possess the potential to revolutionize traditional farming practices, making them more efficient and sustainable.
Benefits of Drones and Autonomous Vehicles:
- Improved accuracy in planting and harvesting
- Reduction in human labor needs
- Ability to gather data across large areas quickly
Precision Agriculture: Enhancing Yields
Precision agriculture signifies a revolutionary approach in farming, using technology to enhance crop yields and resource management. This method optimizes field variability in crops, enabling farmers to produce more food while conserving resources and minimizing environmental impacts. The introduction of AI into precision agriculture has fundamentally shifted how agricultural practitioners monitor and interact with their land.
Soil and Crop Health Monitoring
Soil and crop health monitoring is vital in precision agriculture. It involves using advanced technologies such as sensors, satellite imagery, and drones to collect data about the soil’s nutrient composition and moisture levels. AI algorithms process this data to provide farmers with actionable insights, which inform their decision-making processes.
- Sensors deployed in fields can measure various parameters like pH levels, temperature, and nutrient density. This data helps in identifying specific areas that require attention, ensuring that resources are allocated efficiently.
- AI-driven analysis can predict potential issues before they escalate, enabling preemptive measures that may prevent crop losses due to disease or nutrient deficiencies.
- By accurately assessing soil and crop health, farmers can adjust their input precisely, reducing waste and enhancing yield quality.
Water Management and Irrigation Systems
Water management is another crucial component of precision agriculture. Efficient irrigation systems are essential for maximizing crop yields while minimizing water usage. AI helps in optimizing these systems through sophisticated monitoring and analytics.
- Smart irrigation systems utilize soil moisture sensors and weather data to determine the optimal watering schedule. AI algorithms can analyze patterns and predict moisture needs, ensuring crops receive adequate hydration without over-irrigation.
- Data-driven insights assist farmers in planning irrigation based on rain forecasts and crop requirements, safeguarding against water scarcity.
- This strategic approach to irrigation not only improves yields but also champions sustainability, conserving water resources for future generations.
"Effective soil health and water management are key to the success of precision agriculture, pushing the boundaries of sustainable farming."
AI in Crop Management
Artificial intelligence plays a crucial role in improving crop management. This part of agriculture focuses on enhancing productivity while minimizing negative impacts on the environment. AI applications can lead to better decision-making throughout the crop growth cycle. Utilizing AI in crop management helps farmers better understand crop needs and allows for timely interventions. The integration of technology into agriculture helps to address various challenges that arise in crop production.
Disease Prediction and Management
Disease prediction and management is vital for crop resilience. By leveraging machine learning algorithms, farmers can now predict potential outbreaks of diseases before they escalate. AI solutions analyze historical data and environmental conditions to identify signs of stress in crops. For instance, Google AI has developed tools that use image recognition to spot early signs of diseases in plants. This proactive approach enables farmers to take measures that can include adjusting irrigation or employing fungicides, thus reducing crop loss.
The benefits of AI-driven disease management are numerous:
- Early Detection: Timely identification of problems allows for swift corrective actions.
- Reduced Chemical Use: By targeting specific problems, farmers can avoid excessive application of pesticides and fungicides.
- Higher Yields: Monitoring and managing diseases can directly translate into improved crop yield and quality.
Despite the advantages, there are challenges to consider, such as the requirement for quality data and the need for local adaptation of AI tools. Farmers may need to continuously update their systems to account for evolving strains of pathogens.
Pest Control Strategies
Pest management is another critical area where AI shows promise. Just like disease, pests can cause significant damage to crops. AI systems can help monitor pest populations and suggest control measures based on real-time data analysis. This includes learning patterns of pest movements and their interaction with environment elements. By employing AI, farmers can implement precision pest management strategies.
The following points highlight the advantages of AI applications in pest control:
- Targeted Intervention: AI systems allow for localized pest control measures, reducing overall chemical usage.
- Real-Time Monitoring: Sensors and drones can provide updates on pest populations, assisting in timely responses.
- Cost Efficiency: By minimizing waste and optimizing the use of inputs, farmers can save money in the long run.
Overall, pest control strategies empowered by AI offer a more precise and efficient approach to managing agricultural challenges. As farming methods become increasingly data-driven, the role of AI in crop management continues to grow, emphasizing the importance of adopting such innovations.
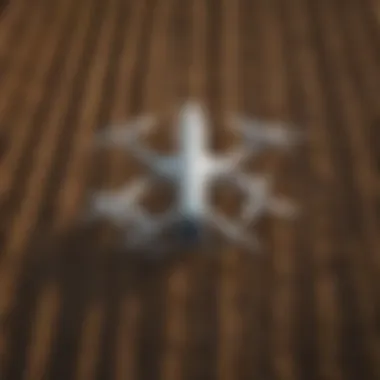
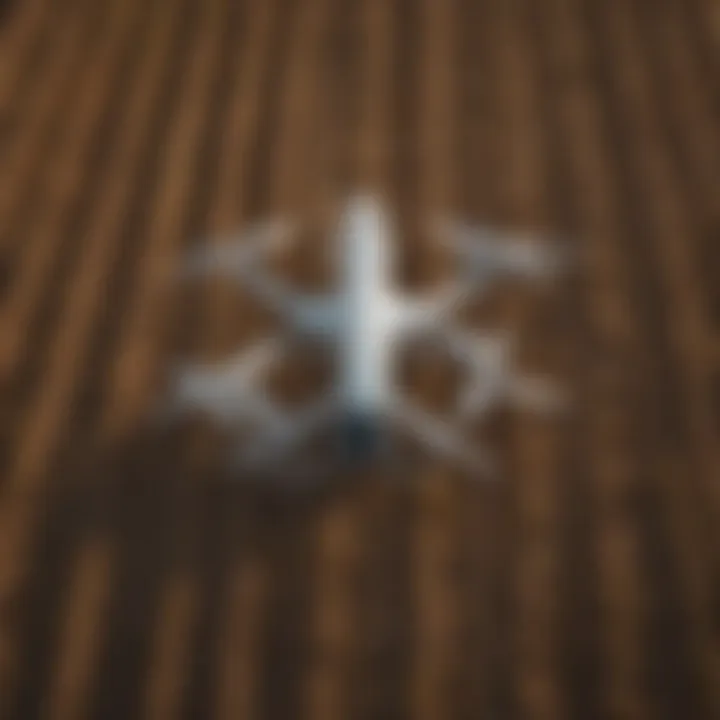
Predictive Analytics in Agriculture
Predictive analytics in agriculture is a pivotal aspect of modern farming. With its ability to analyze vast amounts of data, predictive analytics helps farmers make informed decisions. This technology utilizes historical data, machine learning, and statistical algorithms to forecast future outcomes. The importance of predictive analytics cannot be overstated, as it drives efficiency, enhances productivity, and supports sustainable practices.
Farmers face numerous uncertainties in managing crops and livestock. Weather patterns, market demands, and potential pest outbreaks can all significantly impact yield. By leveraging predictive analytics, farmers gain insights that allow for better planning and resource allocation. Such information can mitigate risks and enhance overall operational efficiency.
Yield Forecasting Models
Yield forecasting models are essential tools within predictive analytics. These models use historical yield data, weather patterns, and soil conditions to predict future crop outputs. Farmers can adjust their strategies based on these forecasts, optimizing planting schedules and resource use. Moreover, accurate yield predictions enable better financial planning. Knowing potential outputs helps farmers make strategic decisions about financing and investment.
Several types of yield forecasting models exist, including:
- Statistical models: These rely on historical data and trends to predict yields.
- Machine learning models: These are more dynamic, adapting to new data inputs to refine predictions over time.
Effective yield forecasting models can lead to considerable economic benefits. Research suggests that accurate forecasting can increase yields by 10 to 20 percent.
Market Prediction and Demand Estimation
Market prediction and demand estimation are equally critical components of predictive analytics in agriculture. Understanding market trends and consumer demands allows farmers to make timely decisions about crop selection and marketing strategies. This responsiveness to market dynamics can significantly enhance profitability.
Farmers can benefit from market prediction through:
- Data-driven insights: By analyzing consumer behavior and market trends, farmers can align their production with demand.
- Risk mitigation: Understanding market fluctuations helps farmers avoid losses during price drops.
- Improved pricing strategies: With better demand estimations, farmers can negotiate prices effectively when selling their produce.
Incorporating predictive analytics into agricultural practices results in smarter farming. With tools aimed at yield forecasting and market estimation, farmers become more adept at navigating complex variables inherent in agriculture. As these models evolve, the agricultural landscape continues to adapt, ensuring productivity and sustainability in a changing world.
"Predictive analytics transforms raw information into actionable insights, promoting more informed and productive farming practices."
By embracing predictive analytics, the agriculture sector not only aims for higher yields, but also fosters a sustainable approach to addressing global food challenges.
AI's Impact on Labor in Agriculture
The introduction of artificial intelligence (AI) in farming brings significant changes to the agricultural labor landscape. This impact is increasingly relevant as society moves towards more innovative farming methods. Understanding the effects of AI on labor is vital for stakeholders in agriculture, including farmers, policy makers, and workers.
AI applications have the potential to improve efficiency but also raise concerns regarding job displacement. As systems become automated, traditional roles may diminish, creating a need for re-skilling and adaptation among the workforce. Hence, a thorough investigation of AI’s intersection with labor is essential.
Automation and Job Displacement
AI-driven automation is transforming agricultural practices. This technology helps in various tasks like planting, harvesting, and monitoring crops. For instance, companies use robotic systems to handle repetitive tasks. This can lead to higher productivity but may also result in job losses in certain areas. Many workers who rely on seasonal jobs may find less employment as machines take over these roles.
Some specific benefits and considerations include:
- Increased Efficiency: Machines can work tirelessly, reducing the time necessary to complete various tasks. This can significantly maximize output and allow farms to scale operations.
- Reduced Labor Costs: Although the upfront investment in AI technology can be substantial, the long-term cost savings from decreased labor needs are appealing to farmers.
- Job Displacement Risks: Workers in roles like manual harvesters may face unemployment. This risk requires serious discussions around economic impacts on communities reliant on seasonal farming jobs.
Adapting to this new reality is paramount. To smooth the transition for displaced workers, agricultural sectors might consider creating programs that reskill these individuals.
New Skill Requirements for Workers
As AI continues to advance, so too must the skills of agricultural workers. The traditional labor force needs to evolve to remain relevant in the changing landscape. New roles will require a combination of agricultural knowledge and technical expertise.
Key points to note about these new skill requirements include:
- Technical Proficiency: Workers will need to understand how to operate and maintain AI systems. Skills in programming and data analysis could become increasingly valuable.
- Adaptability: The ability to embrace and adapt to new technologies will be crucial. Continuous learning will be a mandate as innovation accelerates in agriculture.
- Collaboration: Workers will need to work alongside machines, necessitating collaboration skills. Understanding how to integrate AI into everyday tasks will enhance overall farm productivity.
"The future of agriculture is not just about technology; it’s about people adapting to that technology."
Environmental Implications of AI in Farming
The use of artificial intelligence in agriculture offers not only improvements in crop yield and resource efficiency but also carries significant implications for the environment. As the global population grows, sustainable practices become critical. AI can transform how farms operate, enabling them to use resources more effectively while minimizing their ecological footprint.
AI technologies enable better monitoring of environmental conditions. Through advanced data analytics, farms can assess their impact on ecosystems. This awareness allows them to implement changes that promote sustainability. One of the key benefits AI brings to farming is the optimization of resource management, reducing waste and improving soil and water health.
Sustainability and Resource Management
Sustainability in farming is essential for future generations, and AI plays a central role in this context. Improved precision in resource management means that farmers can apply inputs like fertilizers and water more judiciously. For example, precision irrigation systems powered by AI use data to determine the optimal amount of water needed for specific crops. This reduces water wastage and helps conserve this vital resource in regions that face water scarcity.
AI-driven tools collect and analyze vast amounts of data regarding weather patterns, soil health, and crop requirements. They help farmers make informed decisions that lead to sustainable practices. Here are some important aspects of sustainability achieved through AI:
- Precision Agriculture: Maximizes crop productivity while minimizing resource use.
- Reduced Chemical Use: AI applications help in determining the exact amount of pesticides or fertilizers needed, which decreases chemical runoff into nearby ecosystems.
- Soil Health Monitoring: Continuous assessment of soil quality ensures that essential nutrients are maintained without over-fertilization.
These technologies promote an ecosystem-friendly approach, vital for sustained agricultural production.
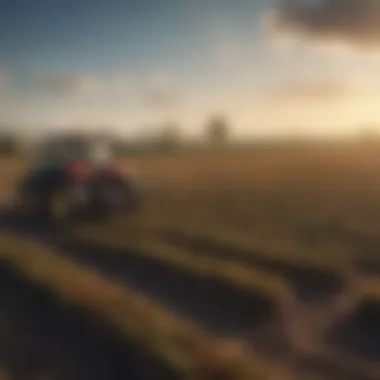
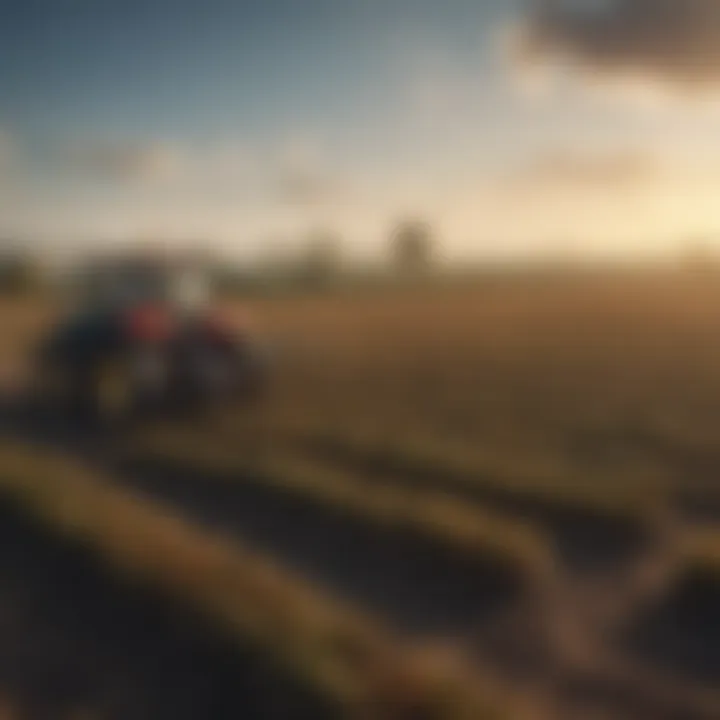
Impact on Biodiversity
The relationship between AI in farming and biodiversity is complex but critically important. Biodiversity contributes to resilience in agricultural systems. By implementing AI solutions, farms can enhance their biodiversity management practices. For instance, AI can help identify and manage non-native or invasive species more effectively.
Using algorithms to analyze data from remote sensors, AI can monitor species diversity and abundance over time. This information is invaluable for making adjustments to farming practices. Here are some ways AI positively impacts biodiversity:
- Habitat Preservation: By optimizing land use, farmers can better manage natural habitats and promote species diversity.
- Pollinator Health: AI helps in tracking pollinator populations, informing farmers about necessary conservation efforts to protect these essential species.
- Crop Diversity: Smart farming encourages the cultivation of diverse crop varieties, promoting ecological balance and resilience against pests and diseases.
"AI has the potential to usher in a new era of thoughtful farming practices that can safeguard biodiversity while ensuring food security."
As agriculture continues to evolve, the integration of AI provides a pathway to not only meet human needs but also promote environmental stewardship. The future of farming, influenced by AI, holds promise for a sustainable and biodiverse environment.
Challenges and Limitations of AI in Agriculture
The use of artificial intelligence in agriculture presents remarkable opportunities. However, it is essential to be aware of the challenges and limitations that accompany this technological shift. Understanding these challenges is crucial as it helps stakeholders navigate potential pitfalls and foster a more sustainable adoption of AI technologies. The importance of addressing these issues cannot be overstated, especially in the context of long-term agricultural practices and the wellbeing of both farmers and consumers.
Data Privacy and Security Concerns
Data privacy and security are paramount in the discourse surrounding AI in agriculture. Collecting vast amounts of farm data can enhance productivity and efficiency. Yet, this also raises significant concerns regarding how this data is stored and used. Farmers must trust that their sensitive information, including crop yields, financial records, and personal details, is protected from unauthorized access and misuse.
Data breaches or cyber attacks could risk not only the farmer's proprietary information but also affect the entire supply chain. As the dependency on data-driven decisions increases, so does the target on farmers' systems from malicious entities. Therefore, implementing robust cybersecurity measures and clear data protection policies is essential to alleviating such concerns.
Infrastructure and Accessibility Issues
Infrastructure plays a critical role in the successful adoption of AI technologies in agriculture. In many rural areas, the necessary digital infrastructure may not be sufficiently developed. This lack of access can severely limit the ability of farmers, especially smallholder farmers, to utilize AI tools effectively. Additionally, there is often a divide between those who can afford the latest technology and those who cannot. This is a barrier to equity in agricultural innovation.
Moreover, educational resources are necessary to understand and implement AI tools. If farmers do not receive adequate training on how to use these technologies, the potential benefits will not be fully realized. Therefore, it is vital for policymakers and industry leaders to focus on enhancing infrastructure and ensuring that all stakeholders have access to education and resources.
"The future of agriculture hinges not just on technology but also on equitable access to that technology."
By comprehensively addressing these challenges, the agricultural sector can create a more inclusive and secure environment for the progression of AI technologies.
Future Trends in AI and Agriculture
The discussion around future trends in agriculture is crucial, as it informs stakeholders in the industry about innovations and shifts that are likely to alter farming practices significantly. Artificial intelligence continues to be a game changer, providing various methods for optimizing production while ensuring sustainability is at the forefront. As technology continues to evolve, its integrated application into farming signifies not just an enhancement in productivity but also a transformation in how farmers approach various challenges.
Integration of AI with IoT
The integration of artificial intelligence with the Internet of Things (IoT) is poised to revolutionize agriculture. This synergy allows data from a multitude of sources to be gathered and analyzed efficiently. IoT devices, such as soil sensors and weather stations, collect valuable data about crop conditions, moisture levels, and nutrient content. When this data is processed through AI algorithms, farmers gain insights that enable precise decision-making. For example, farmers can utilize AI-driven insights to optimize irrigation schedules based on real-time moisture levels, minimizing water use and enhancing crop health.
The benefits of this integration include:
- Improved resource management
- Increased yield prediction accuracy
- Enhanced pest detection systems
Overall, the combination of AI and IoT equips farmers with a robust toolset for adapting to unpredictable conditions and maximizing efficiency.
Ethical Considerations in AI Usage
While the advancements in AI offer numerous benefits, ethical considerations must also be front and center. Issues such as data privacy, algorithmic bias, and the potential for automation to displace labor are significant. As farmers increasingly rely on AI for critical decisions, ensuring that data collected from farms does not infringe on privacy rights is essential. Additionally, the algorithms must be transparent and impartial, as biased AI can lead to harmful practices and unfair advantages in agricultural marketing.
It's also vital to consider the implications of automation on jobs in rural communities. Although AI can lead to efficiency, its rise may result in job losses if workers are not equipped with the necessary skills. Programs should be established that focus on training workers for new roles made possible by AI technology, ensuring a smoother transition for those affected by these shifts.
"The successful implementation of AI in agriculture hinges not only on technology but also on ethical frameworks that guide its development and use."
In summary, navigating the future landscape of AI in farming requires cautious but optimistic engagement with advancements. Attention to integration with IoT enhances operational capabilities, while a focus on ethical implications safeguards the profession's integrity. Understanding these future trends will shape sustainable, efficient, and ethical agriculture in the years ahead.
The End: The Road Ahead for AI in Farming
The integration of artificial intelligence in agriculture not only marks a technological evolution but emphasizes the necessity for sustainable farming practices. As the global population grows, food production must adapt and improve. AI stands as a powerful tool that can bolster productivity, efficiency, and ecological awareness in the farming industry. This conclusion serves as a reflective point on the transformative impacts AI has had and will continue to have on agriculture. It is crucial to embrace these technologies to address current challenges and align with future agricultural trends.
Summarizing Key Insights
In this article, we explored several dimensions of AI in farming. Key insights include the ability of AI to enhance precision agriculture through data-driven decision making. Techniques like soil monitoring, crop health assessment, and effective water management have streamlined farming operations, leading to improved yields and reduced waste. The role of predictive analytics emerged as another critical feature, where data orchestration helps in anticipating weather patterns and market demands. Additionally, the implications of AI on labor have revealed new skill requirements, emphasizing a shift towards more technical skill sets in the workforce.
- Precision Agriculture: Automated systems and sensors for monitoring crops.
- Predictive Analytics: Confirmed that data analysis aids in better decision-making.
- Labor Implications: Highlighted the need for reskilling in agricultural jobs.
Overall, these insights affirm that AI is not simply a tool but a fundamental element reshaping agriculture for the better.
Encouraging Further Research and Development
Advancing AI in farming demands ongoing research and development. As AI technologies become more sophisticated, continuous exploration of their potential applications is essential. There are still significant barriers to overcome, such as data integration and accessibility for rural farmers. Encouraging partnerships between technology developers and agricultural communities will promote innovative solutions.
- Collaboration: Researchers should work closely with farmers to understand practical challenges and needs.
- Investment in Education: Training programs should be established to equip the current and next generation of farmers with AI knowledge.
- Ethical AI Implementation: It’s vital to ensure that AI systems are designed to promote fairness and sustainability.
Research into these areas can uncover new potential, helping the agricultural sector adapt to future demands while conserving resources. More understanding of AI’s implications will guide ethical practices and foster innovation.
The future of farming heavily relies on how well we integrate and adapt AI technologies to cater to both food security and environmental sustainability.